Monopolized decision-making authority or distributed autonomy? Decision-making in a digitalized world
Within the last decades digitalization has penetrated nearly all areas of social life and business, revealing various interesting potentials. The potentials are often related to newly and immediately available sources of information and the linking of previously isolated systems. For businesses, the identification, evaluation and realization of suitable potentials is of essential importance, as the continuous drive for optimization and efficiency as without alternative in a fundamentally competitive economic system.
Decision-making takes a special place in this regard. While optimal decision exist in principle, and mathematical tools are available to determine such solutions, the required computation costs render such approaches unpractical for nearly all non-trivial problems. This becomes particularly apparent if the fundamental information on which decision-making is based upon are characterized by fluctuation and uncertainty. The frequency of fluctuation can easily exceed the required computation time for establish, centralized decision-making approaches. Simultaneously, the increase and abundance of potentially useful information raises the question which information are to be considered in the decision-making process due to potentially improving the solution quality.
Manufacturing companies are increasingly faced with this problem. Digitalization has introduced a huge number of sensors to production systems, continuously collecting information. In the last years, numerous events increased the price fluctuation of resources, energy and final goods. The Covid-19 pandemic showed the interdependence and sensitivity of long supply chains to disruption of any kinds, which were especially significant for complex products. Increasing energy prices are becoming an increasingly relevant decision factor for energy-intensive production processes.
In this environment, information are particularly characterized by short validity and relevance. Established optimization and decision-making concepts monopolize all decision-making authority in order to base decisions on a holistic view of the entire problem, in principle allowing for optimal decision-making. While the implementation of such decision-making is comparatively simple, since only one actor accesses all global information in order to make one global decision. This centralization of decision-making, however, allows no parallelization, hence requiring substantial amounts of time for real world problems.
The fluctuation and variability of decision-relevant information can change much quicker on a digitalized market than optimal decisions can be made. Depending on the scale of fluctuation, it is possible that the information has changed to such a degree during the computation of a centralized solution, that the solution cannot be adapted and the entire decision-making process needs to start again.
Simultaneously, the digitalization of production systems allows for new technical potentials. Single machines or event products themselves can be equipped with microprocessors, sensors and network interfaces. As such, these machines and products can assess their own status, communicate and coordinate within a network and use computation capabilities to makes decisions autonomously – based on either locally available information alone, or coordinating within a network. Machines with such capabilities are referred to as Cyber-physical systems (CPS). CPS collect a variety of information concerning all aspects of production and consequently allow for a new level of transparency and control for manufacturing networks.
Due to the number of potentially autonomous entities within a manufacturing network the established concept of centralized decision-making authority has been questioned. Already in the early 1980s distributed control approaches for manufacturing networks have been theorized, their implementation in practice, however, was impractical due to the computation costs of the time. With the increasing digitalization and dissemination of CPS the technical requirements for distributed control approaches are satisfied in modern manufacturing systems.
Centralized decision-making bases its decision on a holistic view of all available information in a central location in a serial matter, i.e. if the decision problem can be split into multiple subproblems, these still have to be solved subsequently. In distributed approaches, were the decision-making authority is also distributed, a multitude of autonomous entities is capable and allowed to make decision on their own for their respective local scope. Thus, in distributed control approaches a large number of smaller subproblems can be solved in parallel, and also quite fast as the respective subproblems are very simple in comparison. However, in order to create a global solution, communication and coordination between the autonomous entities is required, thus increasing the complexity of implementation significantly compared to centralized approaches.
In practice, few manufacturing systems are control by distributed control approaches. One notable example is semiconductor production, which exhibit a priori unknown production plans, since selected production steps have highly stochastic yields and require a unknown number of repetitions until the desired product quality is achieved. The literature, a number of research streams studies the respective advantages and disadvantages of both centralized and distributed control approaches. While a large number of research articles allow for a deep dive into highly specialized circumstances regarding specific manufacturing network conditions, an overarching framework allowing a holistic comparison was missing.
To answer the fundamental question regarding optimal distriution and assignment of decision-making authority within production system, the term decision-making authority was introduce in Distributing decision-making authority: autonomous entities in manufacturing networks [1]. This concept allows to characterize both entirely centralized approaches, in which all decision-making authority is monopolized by a single actor, as well as entirely distributed approaches in which every potentially autonomous actor has some level of decision-making authority that permits local decision making.
In the literature, numerous specialized manufacturing scenarios are studied in which a specific control approach is particularly suitable. In order to achieve a holistic study on the aptitude of both centralized and distributed control approaches for manufacturing systems it is first necessary to identify all influencing factors on both control approaches. These influence factors include among others the size of the manufacturing network, its topology and the complexity of products and production machines. Numerous influencing factors were grouped as environment variables and categorized in structural complexity and information variability, based on which subsequently a Scheduling Complexity Framework was defined. This framework enables researchers and practitioners alike to asses the suitability of a given manufacturing network for both centralized and distributed control approaches by determining a measure of scheduling complexity which is reflected in the required computation time.
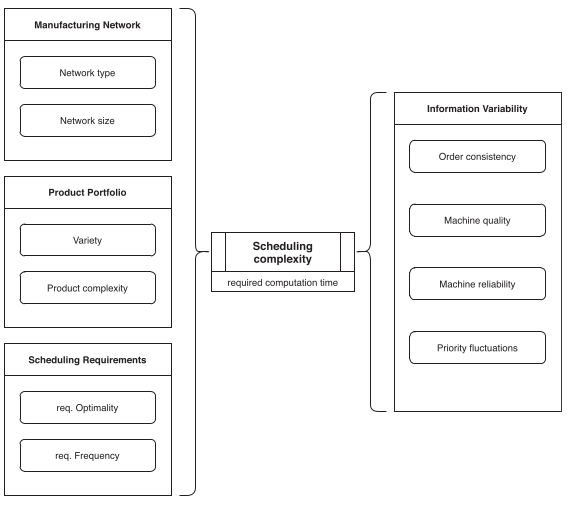
Based on results from the literature regarding specific environment variables first hypotheses regarding the respective influence of said environment variables on the scheduling complexity could be made. In order to systematically evaluate these influences and to assess the importance of subsequent complexity on scheduling a flexible simulation environment was a suitable tool. Due to numerous requirements a proprietary self-developed multi-agent based discrete-event simulation was realized, capable to model any entities of a manufacturing network as either mere command recipients for a centralized control approach as well as enable them to exhibit autonomous decision-making for distributed control approaches. This method allows to study various different decision-making approaches, as the inherent flexibility of agents within the simulation allows modelling any manufacturing networks based on CPS.
Through extensive simulation it was possible to study the influence of all categorized environment variables on the complexity of scheduling. Both an increase in structural complexity such as increased manufacturing network size, the topology of the manufacturing network and the complexity of products as well as increased variability in information such as unreliable machines and fluctuating orders increase the complexity of scheduling. This complexity is directly reflected in the required computation time. In particular, it shows that centralized control approaches can only compensate for limited amounts of scheduling complexity, and only perform best in those scenarios. If scheduling complexity exceeds this limit, driven by frequency and reliability of information, distributed control exhibits far superior performance characteristics regarding solution quality, required computation time and robustness regarding deviations.
The validated Scheduling Complexity Framework is a essential result of the aforementioned dissertation, with implications not limited to production planning and control. Besides research, the framework can be utilized in industry for first assessments regarding suitability of both centralized and distributed control approaches. These first assessments are particularly useful, since the application of distributed control is often associated with intangible benefits and complex implementation. However, modern manufacturing systems based on CPS provide the technical requirements for distributed control in which autonomous entities can realize currently not utilized potentials.
For optimal decision-making structural complexity and information variability are of fundamental importance for the distribution and assignment of decision-making authority. Suitable entities within a decision-making system are capable to make beneficial decisions based on local and topical information nearly instantaneously, exceeding the capabilities of centralized decision-making approaches in sufficiently complex environments.
However, the challenge to make good decisions in ever-decreasing amounts of time and under uncertainty is not limited to industry, but can be found in various areas of our daily lives. The Scheduling Complexity Framework provides first guidance to assess such questions of decision-making authority.
References