2024
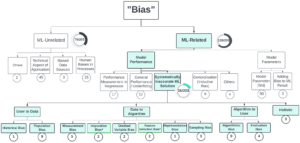
Gesa Götte; Oliver Antons; Andres Herzog; Julia C. Arlinghaus
Perception of biases in machine learning in production research Konferenz
KI 2024 - 47th German Conference on Artificial Intelligence. Würzburg, 25.-27.09.2024, Workshop Proceedings AI in Production - 1 Hochschule für Technik, Wirtschaft und Kultur Leipzig , 2024.
Abstract | Links | BibTeX | Schlagwörter: Articifial Intelligence, Cognitiv Bias, Human Decision-making, Machine learning, Production planning and control
@conference{nokey,
title = {Perception of biases in machine learning in production research},
author = {Gesa G\"{o}tte and Oliver Antons and Andres Herzog and Julia C. Arlinghaus},
url = {https://nbn-resolving.org/urn:nbn:de:bsz:l189-qucosa2-943157},
doi = {10.33968/2024.78},
year = {2024},
date = {2024-10-01},
urldate = {2024-10-01},
booktitle = {KI 2024 - 47th German Conference on Artificial Intelligence. W\"{u}rzburg, 25.-27.09.2024},
publisher = {Hochschule f\"{u}r Technik, Wirtschaft und Kultur Leipzig },
series = {Workshop Proceedings AI in Production - 1},
abstract = {Factories are evolving into Cyber-Physical Production Systems, producing vast data volumes that can be leveraged using computational power. However, an easy and sorrowless integration of machine learning (ML) can lead to too simplistic or false pattern extraction, i.e. biased ML applications. Especially when trained on big data this poses a significant risk when deploying ML. Research has shown that there are sources for undesired biases among the whole ML life cycle and feedback loop between human, data and the ML model. Methods to detect, mitigate and prevent those undesired biases in order to achieve ''fair'' ML solutions have been developed and established in tool boxes in the past years. In this article, we utilize a structured literature review to address the underappreciated biases in ML for production application and highlight the ambiguity of the term bias. It emphasizes the necessity for research on ML biases in production and shows off the most relevant blind spots so far. Filling those blind spots with research and guidelines to incorporate bias screening, treatment and risk assessment in the ML life cycle of industrial applications promises to enhance their robustness, resilience and trustworthiness.},
keywords = {Articifial Intelligence, Cognitiv Bias, Human Decision-making, Machine learning, Production planning and control},
pubstate = {published},
tppubtype = {conference}
}
Factories are evolving into Cyber-Physical Production Systems, producing vast data volumes that can be leveraged using computational power. However, an easy and sorrowless integration of machine learning (ML) can lead to too simplistic or false pattern extraction, i.e. biased ML applications. Especially when trained on big data this poses a significant risk when deploying ML. Research has shown that there are sources for undesired biases among the whole ML life cycle and feedback loop between human, data and the ML model. Methods to detect, mitigate and prevent those undesired biases in order to achieve ''fair'' ML solutions have been developed and established in tool boxes in the past years. In this article, we utilize a structured literature review to address the underappreciated biases in ML for production application and highlight the ambiguity of the term bias. It emphasizes the necessity for research on ML biases in production and shows off the most relevant blind spots so far. Filling those blind spots with research and guidelines to incorporate bias screening, treatment and risk assessment in the ML life cycle of industrial applications promises to enhance their robustness, resilience and trustworthiness.
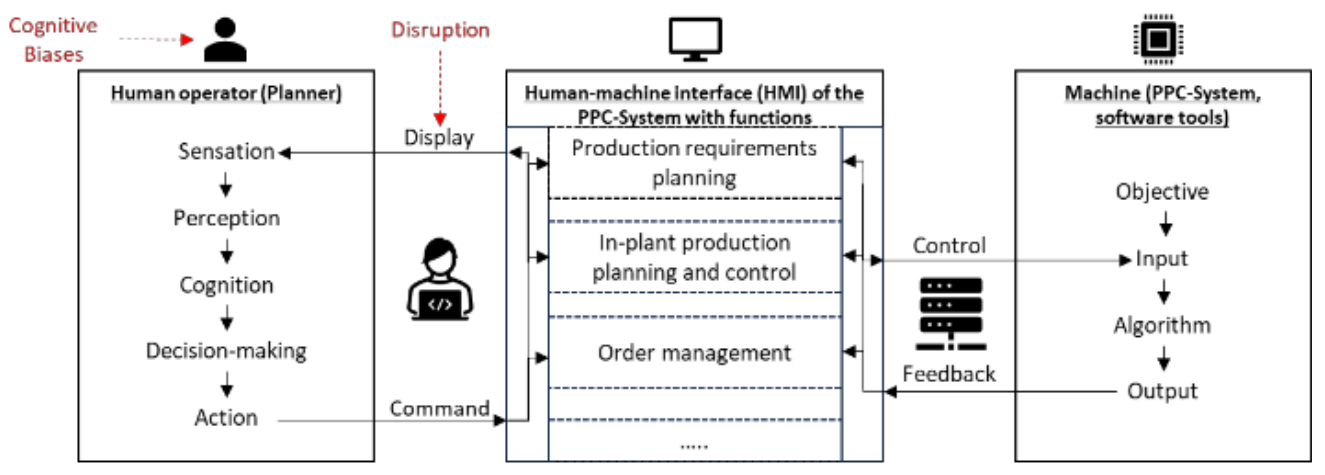
Patrick Rannertshauser; Oliver Antons; Julia C. Arlinghaus
International Conference on Industry 4.0 and Smart Manufacturing, Bd. 232, 2024.
Abstract | Links | BibTeX | Schlagwörter: Cognitiv Bias, Disruption, Production planning and control
@conference{nokey,
title = {Interaction between planners and PPC systems \textendash Derivation of simulation scenarios considering cognitive biases and disruptions},
author = {Patrick Rannertshauser and Oliver Antons and Julia C. Arlinghaus},
editor = {Procedia Computer Science},
url = {https://www.sciencedirect.com/science/article/pii/S1877050924001352?utm_campaign=STMJ_219742_AUTH_SERV_PA\&utm_medium=email\&utm_acid=269271194\&SIS_ID=\&dgcid=STMJ_219742_AUTH_SERV_PA\&CMX_ID=\&utm_in=DM458849\&utm_source=AC_},
doi = {10.1016/j.procs.2024.01.135},
year = {2024},
date = {2024-00-00},
booktitle = {International Conference on Industry 4.0 and Smart Manufacturing},
volume = {232},
pages = {1367-1376},
abstract = {Despite an increase in the availability of digital solutions, planners’ remain at the center of the production planning and control (PPC) decision-making process and have to make numerous decisions involving different information. Due to the inherent complexities, planners’ are supported by PPC systems to fulfil their tasks. Research has shown that humans are influenced by cognitive bias when making decisions, especially in uncertain and complex environments and when disruptions such as machine failures occur. Researchers have therefore proposed debiasing methods to reduce human errors in decision-making. However, there is currently no environment within this research to test these proposed methods. In this study, we aimed to take the first step toward closing this research gap. We combined existing PPC research with a systematic literature review on disruptions in production as well as perspectives from practice through interviews with 12 experts. We investigated the interactions between planners’ and PPC systems with consideration of different cognitive biases and disturbances to derive relevant simulation uses cases.},
keywords = {Cognitiv Bias, Disruption, Production planning and control},
pubstate = {published},
tppubtype = {conference}
}
Despite an increase in the availability of digital solutions, planners’ remain at the center of the production planning and control (PPC) decision-making process and have to make numerous decisions involving different information. Due to the inherent complexities, planners’ are supported by PPC systems to fulfil their tasks. Research has shown that humans are influenced by cognitive bias when making decisions, especially in uncertain and complex environments and when disruptions such as machine failures occur. Researchers have therefore proposed debiasing methods to reduce human errors in decision-making. However, there is currently no environment within this research to test these proposed methods. In this study, we aimed to take the first step toward closing this research gap. We combined existing PPC research with a systematic literature review on disruptions in production as well as perspectives from practice through interviews with 12 experts. We investigated the interactions between planners’ and PPC systems with consideration of different cognitive biases and disturbances to derive relevant simulation uses cases.