2024
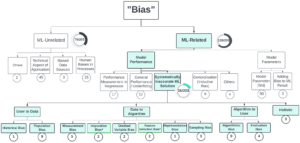
Gesa Götte; Oliver Antons; Andres Herzog; Julia C. Arlinghaus
Perception of biases in machine learning in production research Conference
KI 2024 - 47th German Conference on Artificial Intelligence. Würzburg, 25.-27.09.2024, Workshop Proceedings AI in Production - 1 Hochschule für Technik, Wirtschaft und Kultur Leipzig , 2024.
Abstract | Links | BibTeX | Tags: Articifial Intelligence, Cognitiv Bias, Human Decision-making, Machine learning, Production planning and control
@conference{nokey,
title = {Perception of biases in machine learning in production research},
author = {Gesa G\"{o}tte and Oliver Antons and Andres Herzog and Julia C. Arlinghaus},
url = {https://nbn-resolving.org/urn:nbn:de:bsz:l189-qucosa2-943157},
doi = {10.33968/2024.78},
year = {2024},
date = {2024-10-01},
urldate = {2024-10-01},
booktitle = {KI 2024 - 47th German Conference on Artificial Intelligence. W\"{u}rzburg, 25.-27.09.2024},
publisher = {Hochschule f\"{u}r Technik, Wirtschaft und Kultur Leipzig },
series = {Workshop Proceedings AI in Production - 1},
abstract = {Factories are evolving into Cyber-Physical Production Systems, producing vast data volumes that can be leveraged using computational power. However, an easy and sorrowless integration of machine learning (ML) can lead to too simplistic or false pattern extraction, i.e. biased ML applications. Especially when trained on big data this poses a significant risk when deploying ML. Research has shown that there are sources for undesired biases among the whole ML life cycle and feedback loop between human, data and the ML model. Methods to detect, mitigate and prevent those undesired biases in order to achieve ''fair'' ML solutions have been developed and established in tool boxes in the past years. In this article, we utilize a structured literature review to address the underappreciated biases in ML for production application and highlight the ambiguity of the term bias. It emphasizes the necessity for research on ML biases in production and shows off the most relevant blind spots so far. Filling those blind spots with research and guidelines to incorporate bias screening, treatment and risk assessment in the ML life cycle of industrial applications promises to enhance their robustness, resilience and trustworthiness.},
keywords = {Articifial Intelligence, Cognitiv Bias, Human Decision-making, Machine learning, Production planning and control},
pubstate = {published},
tppubtype = {conference}
}
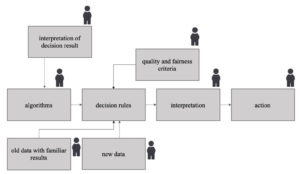
Melanie Kessler; Oliver Antons; Julia C. Arlinghaus
Designing hybrid intelligence: understanding the impact of human decision-making on AI Best Paper Conference
Human Aspects of Advanced Manufacturing, Production Management and Process Control. AHFE, vol. 146, AHFE International, USA, 2024.
Abstract | Links | BibTeX | Tags: Articifial Intelligence, Human Decision-making, Hybrid Intelligence
@conference{nokey,
title = {Designing hybrid intelligence: understanding the impact of human decision-making on AI},
author = {Melanie Kessler and Oliver Antons and Julia C. Arlinghaus },
editor = {Beata Mrugalska, Waldemar Karwowski and Stefan Trzcielinski},
url = {https://oliver.antons.eu/best-paper-award-ahfe-2024-conference/, Best Paper Award
http://doi.org/10.54941/ahfe1005148},
doi = {10.54941/ahfe1005148},
year = {2024},
date = {2024-07-01},
urldate = {2024-07-01},
booktitle = {Human Aspects of Advanced Manufacturing, Production Management and Process Control. AHFE},
volume = {146},
pages = {31-39},
address = {AHFE International, USA},
abstract = {In many domains such as management, production and government, established control approaches struggle to address increasing complexity in a timely manner, resulting in a demand for more agile methods. Hybrid intelligence and decision support systems are useful approaches to augment human decision-making through artificial intelligence (AI). Various application of AI methods to estimate production parameters or to provide forecasts are discussed in the literature or already being implemented, however, human decision-making is still required for either deciding whether to follow specific suggestions or for monitoring their respective implementation. But human behavioral research has shown that human decision-making is rather biased than fully rational, leading to unintended consequences in the collaborative work of humans and machines. Subsequently, the research stream of hybrid intelligence has gained interest recently, aiming to study the collaboration between humans and machines. We contribute to this issue by combining a systematic literature review on AI and cognitive biases combined with practical insights from discussions with experts in order to derive first guidelines addressing the human factor in the design of AI-based decision support systems for complex production environments.},
keywords = {Articifial Intelligence, Human Decision-making, Hybrid Intelligence},
pubstate = {published},
tppubtype = {conference}
}