2024
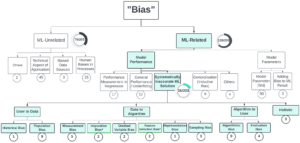
Gesa Götte; Oliver Antons; Andres Herzog; Julia C. Arlinghaus
Perception of biases in machine learning in production research Conference
KI 2024 - 47th German Conference on Artificial Intelligence. Würzburg, 25.-27.09.2024, Workshop Proceedings AI in Production - 1 Hochschule für Technik, Wirtschaft und Kultur Leipzig , 2024.
Abstract | Links | BibTeX | Tags: Articifial Intelligence, Cognitiv Bias, Human Decision-making, Machine learning, Production planning and control
@conference{nokey,
title = {Perception of biases in machine learning in production research},
author = {Gesa G\"{o}tte and Oliver Antons and Andres Herzog and Julia C. Arlinghaus},
url = {https://nbn-resolving.org/urn:nbn:de:bsz:l189-qucosa2-943157},
doi = {10.33968/2024.78},
year = {2024},
date = {2024-10-01},
urldate = {2024-10-01},
booktitle = {KI 2024 - 47th German Conference on Artificial Intelligence. W\"{u}rzburg, 25.-27.09.2024},
publisher = {Hochschule f\"{u}r Technik, Wirtschaft und Kultur Leipzig },
series = {Workshop Proceedings AI in Production - 1},
abstract = {Factories are evolving into Cyber-Physical Production Systems, producing vast data volumes that can be leveraged using computational power. However, an easy and sorrowless integration of machine learning (ML) can lead to too simplistic or false pattern extraction, i.e. biased ML applications. Especially when trained on big data this poses a significant risk when deploying ML. Research has shown that there are sources for undesired biases among the whole ML life cycle and feedback loop between human, data and the ML model. Methods to detect, mitigate and prevent those undesired biases in order to achieve ''fair'' ML solutions have been developed and established in tool boxes in the past years. In this article, we utilize a structured literature review to address the underappreciated biases in ML for production application and highlight the ambiguity of the term bias. It emphasizes the necessity for research on ML biases in production and shows off the most relevant blind spots so far. Filling those blind spots with research and guidelines to incorporate bias screening, treatment and risk assessment in the ML life cycle of industrial applications promises to enhance their robustness, resilience and trustworthiness.},
keywords = {Articifial Intelligence, Cognitiv Bias, Human Decision-making, Machine learning, Production planning and control},
pubstate = {published},
tppubtype = {conference}
}
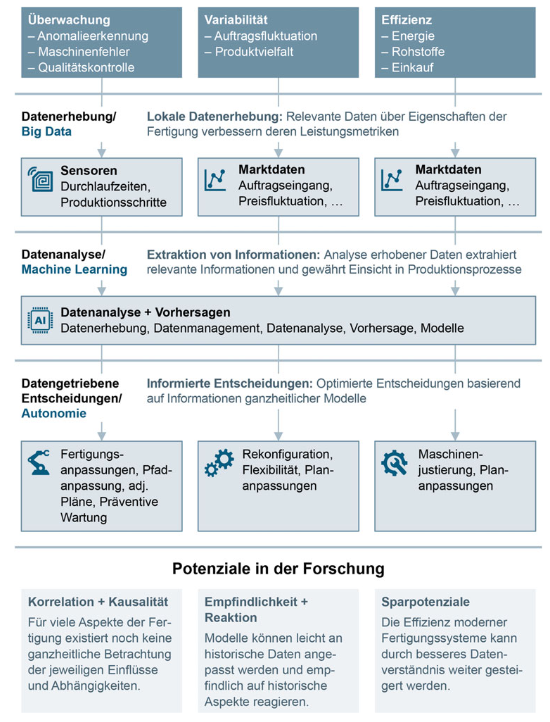
Julia C. Arlinghaus; Oliver Antons
Planung und Steuerung für die digitale Produktion Book Chapter
In: Berlin Springer Vieweg, Heidelberg (Ed.): Handbuch Unternehmensorganisation, Springer Vieweg, Berlin, Heidelberg, 2024, ISBN: 978-3-642-45370-0.
Abstract | Links | BibTeX | Tags: Autonomous production control, Autonomy & Decision-making Authority, Cyber-physical system, Digitalization, Industry 4.0, Production planning and control, Smart manufacutring systems
@inbook{Arlinghaus2024,
title = {Planung und Steuerung f\"{u}r die digitale Produktion},
author = {Julia C. Arlinghaus and Oliver Antons},
editor = {Springer Vieweg, Berlin, Heidelberg},
url = {https://link.springer.com/referenceworkentry/10.1007/978-3-642-45370-0_63-2},
doi = {10.1007/978-3-642-45370-0_63-2},
isbn = {978-3-642-45370-0},
year = {2024},
date = {2024-07-01},
urldate = {2024-07-01},
booktitle = {Handbuch Unternehmensorganisation},
publisher = {Springer Vieweg, Berlin, Heidelberg},
abstract = {Im vergangenen Jahrzehnt wurde die produzierende Industrie mit einem zunehmend volatileren Umfeld konfrontiert. Unterschiedlichste Krisen haben etablierte Lieferketten ersch\"{u}ttert und die Notwendigkeit resilienter und flexibler Produktionsplanung und -steuerung aufgezeigt. Zeitgleich hat eine voranschreitende Digitalisierung der Produktionsanlagen neue Herausforderungen, Potenziale und Chancen aufgeworfen. Cyber-physikalische Systeme und ein Industrial Internet of Things erm\"{o}glichen Digitale Zwillinge der Produktion und erf\"{u}llen die technischen Voraussetzungen f\"{u}r eine autonome Entscheidungsfindung auf einzelnen Produktionssystemen. In diesem Kontext stellt sich f\"{u}r Unternehmen eine fundamentale Frage der Organisation hinsichtlich der Architektur von Produktionsplanung und -steuerung. Mit zentralisierter sowie verteilter Produktionsplanung und -steuerung stehen Unternehmen zwei gegenl\"{a}ufige Ans\"{a}tze zur Verf\"{u}gung, die in diesem Beitrag n\"{a}her betrachtet werden.},
keywords = {Autonomous production control, Autonomy \& Decision-making Authority, Cyber-physical system, Digitalization, Industry 4.0, Production planning and control, Smart manufacutring systems},
pubstate = {published},
tppubtype = {inbook}
}
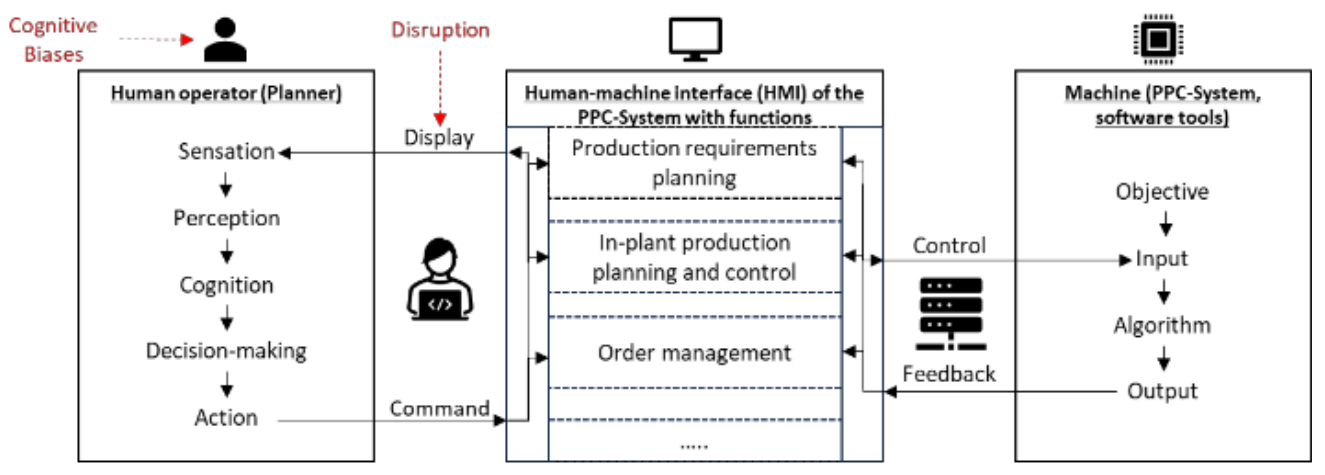
Patrick Rannertshauser; Oliver Antons; Julia C. Arlinghaus
International Conference on Industry 4.0 and Smart Manufacturing, vol. 232, 2024.
Abstract | Links | BibTeX | Tags: Cognitiv Bias, Disruption, Production planning and control
@conference{nokey,
title = {Interaction between planners and PPC systems \textendash Derivation of simulation scenarios considering cognitive biases and disruptions},
author = {Patrick Rannertshauser and Oliver Antons and Julia C. Arlinghaus},
editor = {Procedia Computer Science},
url = {https://www.sciencedirect.com/science/article/pii/S1877050924001352?utm_campaign=STMJ_219742_AUTH_SERV_PA\&utm_medium=email\&utm_acid=269271194\&SIS_ID=\&dgcid=STMJ_219742_AUTH_SERV_PA\&CMX_ID=\&utm_in=DM458849\&utm_source=AC_},
doi = {10.1016/j.procs.2024.01.135},
year = {2024},
date = {2024-00-00},
booktitle = {International Conference on Industry 4.0 and Smart Manufacturing},
volume = {232},
pages = {1367-1376},
abstract = {Despite an increase in the availability of digital solutions, planners’ remain at the center of the production planning and control (PPC) decision-making process and have to make numerous decisions involving different information. Due to the inherent complexities, planners’ are supported by PPC systems to fulfil their tasks. Research has shown that humans are influenced by cognitive bias when making decisions, especially in uncertain and complex environments and when disruptions such as machine failures occur. Researchers have therefore proposed debiasing methods to reduce human errors in decision-making. However, there is currently no environment within this research to test these proposed methods. In this study, we aimed to take the first step toward closing this research gap. We combined existing PPC research with a systematic literature review on disruptions in production as well as perspectives from practice through interviews with 12 experts. We investigated the interactions between planners’ and PPC systems with consideration of different cognitive biases and disturbances to derive relevant simulation uses cases.},
keywords = {Cognitiv Bias, Disruption, Production planning and control},
pubstate = {published},
tppubtype = {conference}
}
2023
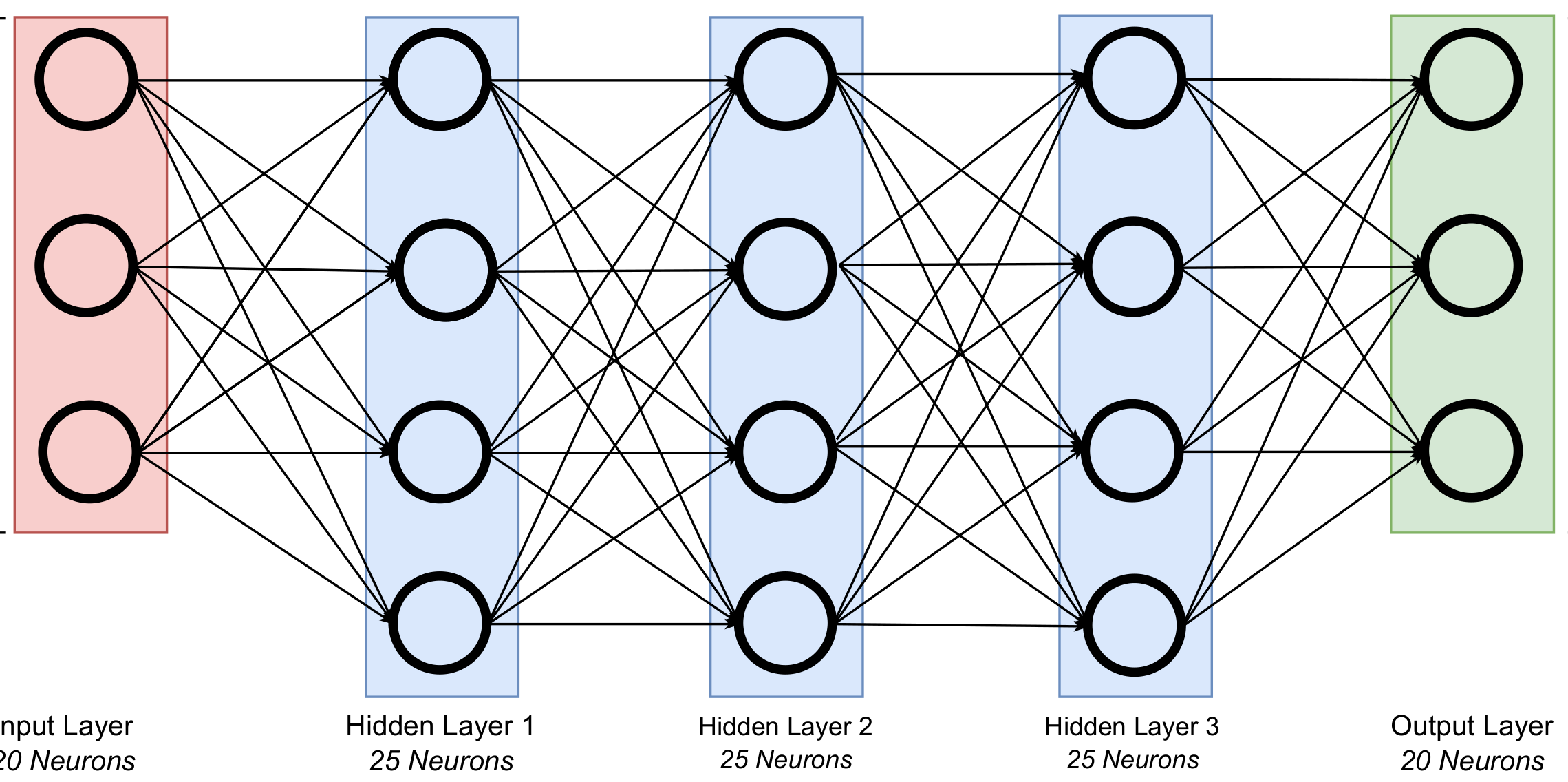
Oliver Antons; Julia C. Arlinghaus
Maximum Likelihood and Neural Network Estimators for Distributed Production Control Proceedings Article
In: IFAC-PapersOnLine , pp. 10327-10332, 2023, ISSN: 2405-8963, (22nd IFAC World Congress).
Abstract | Links | BibTeX | Tags: Autonomy & Decision-making Authority, Cyber-physical system, Distributed control, Machine learning, Production planning and control, Smart manufacutring systems
@inproceedings{nokey,
title = {Maximum Likelihood and Neural Network Estimators for Distributed Production Control},
author = {Oliver Antons and Julia C. Arlinghaus},
url = {https://www.sciencedirect.com/science/article/pii/S2405896323014210},
doi = {10.1016/j.ifacol.2023.10.1038},
issn = {2405-8963},
year = {2023},
date = {2023-07-14},
urldate = {2023-07-14},
booktitle = {IFAC-PapersOnLine },
volume = {56},
number = {2},
pages = {10327-10332},
abstract = {Cyber-physical systems have become increasingly common in recent years, providing a multitude of information regarding production processes. At the same time, increasing volatilities, uncertainties, complexity and ambiguity (VUCA) are challenging existing production control approaches for manufacturing networks. Data-driven control approaches are an avenue to address VUCA, but require further study in research and practice. We utilize a multi-agent based discrete-event simulation to compare the aptitudes of a maximum likelihood and neural network based estimator for distributed production control, and provide insights into application of machine learning to address ever increasing information and VUCA.},
note = {22nd IFAC World Congress},
keywords = {Autonomy \& Decision-making Authority, Cyber-physical system, Distributed control, Machine learning, Production planning and control, Smart manufacutring systems},
pubstate = {published},
tppubtype = {inproceedings}
}
Oliver Antons; Julia C. Arlinghaus
Designing distributed decision-making authorities for smart factories – understanding the role of manufacturing network architecture Journal Article
In: International Journal of Production Research, vol. 0, no. 0, pp. 1-19, 2023.
Abstract | Links | BibTeX | Tags: Autonomous production control, Autonomy & Decision-making Authority, Centralized control, Decision-making authority, Distributed control, Manufacturing network topology, Production planning and control
@article{doi:10.1080/00207543.2023.2217285,
title = {Designing distributed decision-making authorities for smart factories \textendash understanding the role of manufacturing network architecture},
author = {Oliver Antons and Julia C. Arlinghaus},
url = {https://doi.org/10.1080/00207543.2023.2217285},
doi = {10.1080/00207543.2023.2217285},
year = {2023},
date = {2023-01-01},
urldate = {2023-01-01},
journal = {International Journal of Production Research},
volume = {0},
number = {0},
pages = {1-19},
publisher = {Taylor \& Francis},
abstract = {The availability of cyber-physical systems (CPS) in modern manufacturing networks provides a multitude of interesting opportunities from a manufacturing control perspective. Providing sensors, data gathering, local computation and communication capabilities modern CPS fulfil the technical requirements to act completely autonomously in a manufacturing network. While the distribution of decision-making authority to autonomous entities is feasible given such requirements, practice often sees the monopolisation of decision-making authority for centralised control. However, distributed production control approaches might be better suited given current manufacturing challenges, ranging from unreliable supply chains over highly volatile markets, to the demand for increasingly efficient and highly customisable production. In this article, we extend an existing scheduling complexity framework which enables practitioners and researchers alike to assess the aptitude of given manufacturing networks for both centralised and distributed control. In particular, we study the influence of a manufacturing network's topology ranging from assembly line to job shops on the aforementioned aptitude, with total production costs as objective.
We utilise a multi-agent-based discrete-event simulation comparing an MILP-based centralised control approach and an autonomy based distributed control approach with weighted costs as decision function to evaluate this framework. Our results provide novel insights regarding the influence of manufacturing network topologies on the scheduling complexity of manufacturing networks.},
keywords = {Autonomous production control, Autonomy \& Decision-making Authority, Centralized control, Decision-making authority, Distributed control, Manufacturing network topology, Production planning and control},
pubstate = {published},
tppubtype = {article}
}
We utilise a multi-agent-based discrete-event simulation comparing an MILP-based centralised control approach and an autonomy based distributed control approach with weighted costs as decision function to evaluate this framework. Our results provide novel insights regarding the influence of manufacturing network topologies on the scheduling complexity of manufacturing networks.
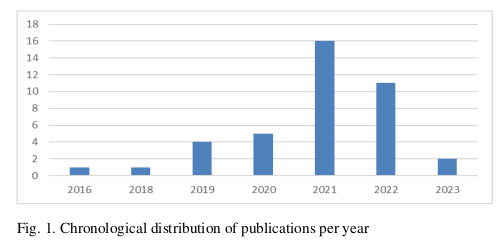
Konstantin Büttner; Oliver Antons; Julia C. Arlinghaus
Exploring Implementation Barriers of Machine Learning in Production Planning and Control Conference
vol. 120, 2023, (56th CIRP CMS 2023 - Manufacturing in an age of disruption).
Abstract | Links | BibTeX | Tags: Articifial Intelligence, Machine learning, Production planning and control
@conference{nokey,
title = {Exploring Implementation Barriers of Machine Learning in Production Planning and Control},
author = {Konstantin B\"{u}ttner and Oliver Antons and Julia C. Arlinghaus},
url = {https://www.sciencedirect.com/science/article/pii/S2212827123009435},
doi = {10.1016/j.procir.2023.09.211},
year = {2023},
date = {2023-00-00},
urldate = {2023-00-00},
volume = {120},
pages = {1546-1551},
abstract = {This paper studies the challenges encountered during the implementation of machine learning (ML) applications in production planning and control (PPC) functions of manufacturing companies. The application of ML in PPC promises numerous benefits, and several studies have revealed the positive impact of such an implementation on key performance indicators of production systems, such as lead time, due date reliability, and inventory levels. However, despite the theoretical potential and the increased research interest in the field over the last five years, practical applications remain rare and discourse on the existence of this gap between research and practice remains relatively sparse. In this paper, we identify general ML implementation barriers the manufacturing domain based on a structured literature review and evaluate the relevance of these barriers within the PPC domain by interviewing ML and PPC experts. This research is beneficial for practitioners and researchers who aim to implement ML applications in PPC by enabling them to evaluate the possible barriers and challenges during implementation projects within their organizations.},
note = {56th CIRP CMS 2023 - Manufacturing in an age of disruption},
keywords = {Articifial Intelligence, Machine learning, Production planning and control},
pubstate = {published},
tppubtype = {conference}
}
2022
Oliver Antons
Distributing decision-making authority: autonomous entities in manufacturing networks PhD Thesis
Rheinisch-Westfälische Technische Hochschule Aachen, 2022, (Veröffentlicht auf dem Publikationsserver der RWTH Aachen University; Dissertation, Rheinisch-Westfälische Technische Hochschule Aachen, 2022).
Abstract | Links | BibTeX | Tags: Autonomy, Autonomy & Decision-making Authority, Decision-making authority, Discrete-event simulation, Multi-agent system, Production planning and control
@phdthesis{Antons:856980,
title = {Distributing decision-making authority: autonomous entities in manufacturing networks},
author = {Oliver Antons},
url = {https://oliver.antons.eu/research/decision-making-authority/ , Autonomy \& Decision-making Authority
https://publications.rwth-aachen.de/record/856980 , RWTH Publication Server},
doi = {10.18154/RWTH-2022-11291},
year = {2022},
date = {2022-12-16},
urldate = {2022-12-16},
pages = {1 Online-Ressource : Illustrationen},
publisher = {RWTH Aachen University},
address = {Aachen},
school = {Rheinisch-Westf\"{a}lische Technische Hochschule Aachen},
abstract = {Industrial production was faced with increasing challenges in the last years. Market volatility, rising energy costs and disrupted supply networks resulted in an ever-increasing information variability, which decreases productivity and complicates production planning and control (PPC). Moreover, the ever-increasing demand for, and differentiation through customization also makes planning more difficult. At the same time manufacturing networks have seen a further computerization on a machine level by the introduction of cyber-physical systems (CPS). Capable to process information, gather sensor data locally and communicate within a network, these machine provide an enormous increase in potentials for manufacturing networks. Thus, the technical requirements for distributed production control approaches are fulfilled, based on CPS acting as autonomous entities within a manufacturing network. Such distributed production control approaches feature a number of interesting characteristics, which are often quite contrary to established concepts of traditional, centralized production control. In the literature, many research streams are concerned with the advantages and disadvantages of both centralized and distributed control. While many articles provide deep insights into the workings of specialized control approaches for specific manufacturing environments, an overarching framework allowing a holistic comparison between the two fundamental production control approaches is lacking. In this thesis, the term decision-making authority is introduced to describe the level of autonomy an entity is allowed to exhibit with regard to the potential decisions it could make. Furthermore, both centralized and distributed production control approaches for manufacturing networks based on potentially autonomous entities are explored. In the former case, every entity but one central controller is not allowed to exhibit any decision-making authority, acting purely as command recipients. In the latter case, however, the aforementioned entities have a predefined degree of decision-making authority, enabling them to make certain decisions of the production scheduling on their own. Based on environment variables derived by extensive literature review, a sophisticated simulation framework is developed in form of a multi-agent based discrete-event simulation (MAS-DES). This simulation framework represents all objects of a manufacturing network, such as machines and products as agents. These agents can either follow a global plan, derived from a mixed-integer linear program modeling a centralized production control approach, or act autonomously within the scope of their respective decision-making authority in a distributed production control approach. The main part of this thesis consists of five research articles, presented in Chapters II - VI. Chapter II reviews the historic ply between centralized and decentralized control, followed by a structured literature review regarding autonomy in production planning and control, manufacturing and related research streams. Extending this, Chapter III studies the difference in information scopes of different classes of potentially autonomous entities in a manufacturing network. Chapter IV provides guidance to both researchers and practitioners alike by introducing a scheduling complexity framework, based on environment variables derived from the literature. A multi-agent based discrete-event simulation is utilized to validate the framework quantitatively. Following, Chapter V extends the simulations to study the influence of a manufacturing network’s topology on its aptitude for both centralized and distributed production control approaches. Chapter VI explores synergistic potentials between machine learning and distributed production control for manufacturing networks. Lastly, the thesis ends with a conclusion summarizing results, noting limitations and presenting avenues for future research.},
note = {Ver\"{o}ffentlicht auf dem Publikationsserver der RWTH Aachen
University; Dissertation, Rheinisch-Westf\"{a}lische Technische
Hochschule Aachen, 2022},
keywords = {Autonomy, Autonomy \& Decision-making Authority, Decision-making authority, Discrete-event simulation, Multi-agent system, Production planning and control},
pubstate = {published},
tppubtype = {phdthesis}
}
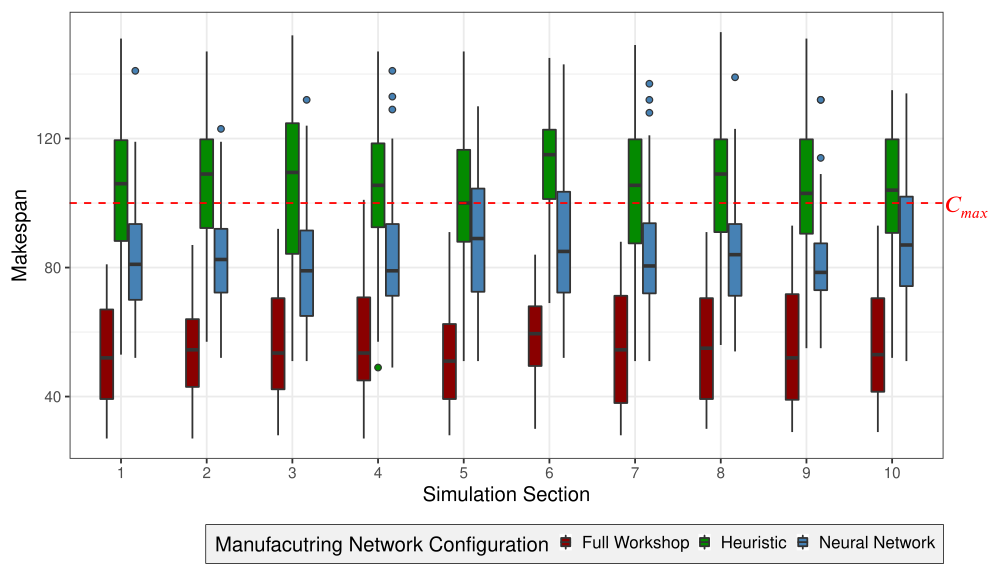
Oliver Antons; Julia C. Arlinghaus
Data-driven and autonomous manufacturing control in cyber-physical production systems Journal Article
In: Computers in Industry, vol. 141, pp. 103711, 2022, ISSN: 0166-3615.
Abstract | Links | BibTeX | Tags: Autonomous production control, Autonomy & Decision-making Authority, Machine learning, Neural network, Production planning and control
@article{ANTONS2022103711,
title = {Data-driven and autonomous manufacturing control in cyber-physical production systems},
author = {Oliver Antons and Julia C. Arlinghaus},
url = {https://www.sciencedirect.com/science/article/pii/S0166361522001087},
doi = {https://doi.org/10.1016/j.compind.2022.103711},
issn = {0166-3615},
year = {2022},
date = {2022-01-01},
urldate = {2022-01-01},
journal = {Computers in Industry},
volume = {141},
pages = {103711},
abstract = {Modern manufacturing networks consist of cyber-physical systems (CPS) which offer an array of interesting capabilities, ranging from local computation over data generation to communication capabilities. As traditional control approaches fail to fully leverage these capabilities, the last decade has seen a renewed interest in distributed control approaches based on autonomous entities. In this article, we study the synergistic potentials of autonomous control and machine learning in a job-shop setting, addressing challenges of modern manufacturing such as market fluctuation and process time variance, thus leveraging the potentials of CPS in order to flexibly configure manufacturing networks and achieve cost-minimal production. We utilize a multi-agent based discrete-event simulation to compare this novel approach to a traditional heuristic, underlining the potentials and advantages of data-driven control approaches.},
keywords = {Autonomous production control, Autonomy \& Decision-making Authority, Machine learning, Neural network, Production planning and control},
pubstate = {published},
tppubtype = {article}
}
2021
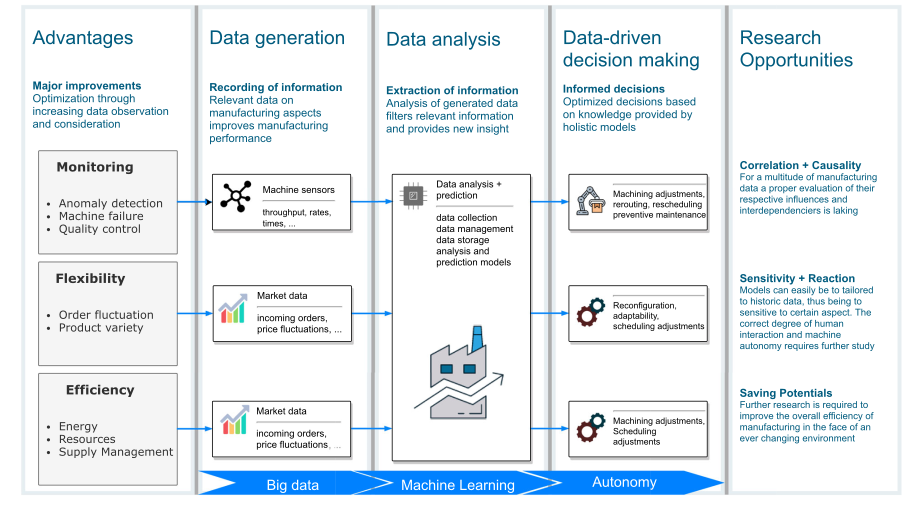
Oliver Antons; Julia C. Arlinghaus
Distributing decision-making authority in manufacturing – review and roadmap for the factory of the future Journal Article
In: International Journal of Production Research, vol. 60, iss. 13, no. 0, pp. 1-19, 2021.
Abstract | Links | BibTeX | Tags: Autonomous production control, Autonomy, Autonomy & Decision-making Authority, Decentralized Control, Industry 4.0, Production planning and control
@article{Antons2021Distributing,
title = {Distributing decision-making authority in manufacturing \textendash review and roadmap for the factory of the future},
author = {Oliver Antons and Julia C. Arlinghaus},
url = {https://doi.org/10.1080/00207543.2022.2057255},
doi = {10.1080/00207543.2022.2057255},
year = {2021},
date = {2021-07-27},
urldate = {2021-07-27},
journal = {International Journal of Production Research},
volume = {60},
number = {0},
issue = {13},
pages = {1-19},
publisher = {Taylor \& Francis},
abstract = {The question of the benefits of autonomous control is more important than ever: production managers, governments and society hope that the vision of smart and digital production systems with high flexibility and low costs may save the value adding and therefore welfare in the high wage, industrialised countries. At the same time, the discussion on the social implications of autonomous objects and decentralised control approaches is growing. Looking back on the history of production research and practice, we find that there has been a constant ply among scholars and production managers between the advantages of the two concepts of centralised and decentralised control approaches. In this article, we study the concept of autonomy in production planning and control, enabled by cyber-physical systems and the distribution of decision-making authority. Based on a profound structured literature review, we analyse the perception of autonomy, the technological requirements and the increasing complexities of modern smart manufacturing. Moreover, we find that recently several research streams suggest the advantages and benefits of autonomous control concepts compared to traditional centralised approaches based on qualitative analysis and identify a distinct lack of quantitative results.},
keywords = {Autonomous production control, Autonomy, Autonomy \& Decision-making Authority, Decentralized Control, Industry 4.0, Production planning and control},
pubstate = {published},
tppubtype = {article}
}
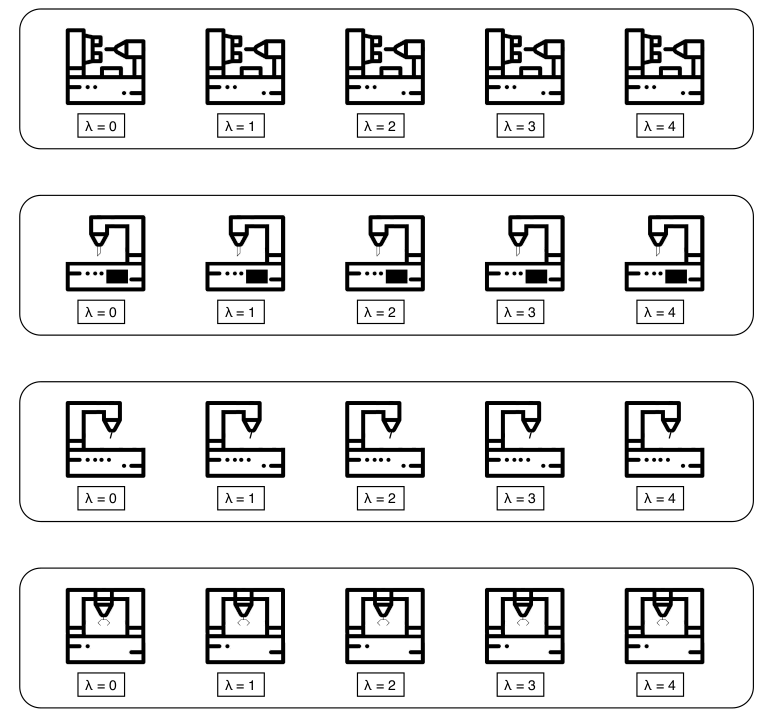
Oliver Antons; Julia C. Arlinghaus
Learning Distributed Control for Job Shops-A Comparative Simulation Study Proceedings Article
In: Service Oriented, Holonic and Multi-Agent Manufacturing Systems for Industry of the Future: Proceedings of SOHOMA 2020, pp. 193–202, Springer International Publishing 2021.
Abstract | Links | BibTeX | Tags: Autonomy & Decision-making Authority, Discrete-event simulation, Job-shop scheduling, Multi-agent system, Production planning and control, Self-learning
@inproceedings{antons2021learning,
title = {Learning Distributed Control for Job Shops-A Comparative Simulation Study},
author = {Oliver Antons and Julia C. Arlinghaus},
url = {https://link.springer.com/book/10.1007/978-3-030-69373-2},
doi = {10.1007/978-3-030-69373-2},
year = {2021},
date = {2021-01-01},
urldate = {2021-01-01},
booktitle = {Service Oriented, Holonic and Multi-Agent Manufacturing Systems for Industry of the Future: Proceedings of SOHOMA 2020},
pages = {193--202},
organization = {Springer International Publishing},
abstract = {This paper studies the potentials of learning and benefits of local data processing in a distributed control setting. We deploy a multi-agent system in the context of a discrete-event simulation to model distributed control for a job shop manufacturing system with variable processing times and multi-stage production processes. Within this simulation, we compare queue length estimation as dispatching rule against a variation with learning capability, which processes additional historic data on a machine agent level, showing the potentials of learning and coordination for distributed control in PPC.},
keywords = {Autonomy \& Decision-making Authority, Discrete-event simulation, Job-shop scheduling, Multi-agent system, Production planning and control, Self-learning},
pubstate = {published},
tppubtype = {inproceedings}
}
2020
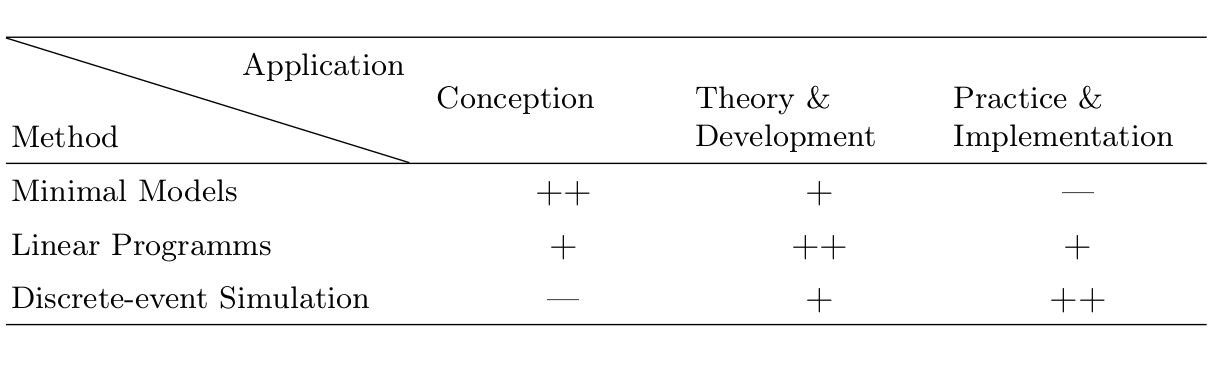
Oliver Antons; Julia C. Arlinghaus
Modelling Autonomous Production Control: A Guide to Select the Most Suitable Modelling Approach Proceedings Article
In: International Conference on Dynamics in Logistics, pp. 245–253, Springer 2020.
Abstract | Links | BibTeX | Tags: Autonomous production control, Autonomy & Decision-making Authority, Discrete-event simulation, Linear programming, Minimal models, Production planning and control
@inproceedings{antons2020modelling,
title = {Modelling Autonomous Production Control: A Guide to Select the Most Suitable Modelling Approach},
author = {Oliver Antons and Julia C. Arlinghaus},
url = {https://link.springer.com/chapter/10.1007/978-3-030-44783-0_24},
doi = {10.1007/978-3-030-44783-0_24},
year = {2020},
date = {2020-01-01},
urldate = {2020-01-01},
booktitle = {International Conference on Dynamics in Logistics},
pages = {245--253},
organization = {Springer},
abstract = {This paper studies and compares Minimal Models, Linear Programming and Discrete-event Simulation as approaches to model Production Planning and Control with regard to their ability to include the concept of autonomous control. After a brief explanation of autonomous control in production planning, the three aforementioned concepts are introduced in detail. We derive their benefits and drawbacks for different scenarios, and subsequently give advice when to deploy each method, applicable for researchers and practioners alike.},
keywords = {Autonomous production control, Autonomy \& Decision-making Authority, Discrete-event simulation, Linear programming, Minimal models, Production planning and control},
pubstate = {published},
tppubtype = {inproceedings}
}
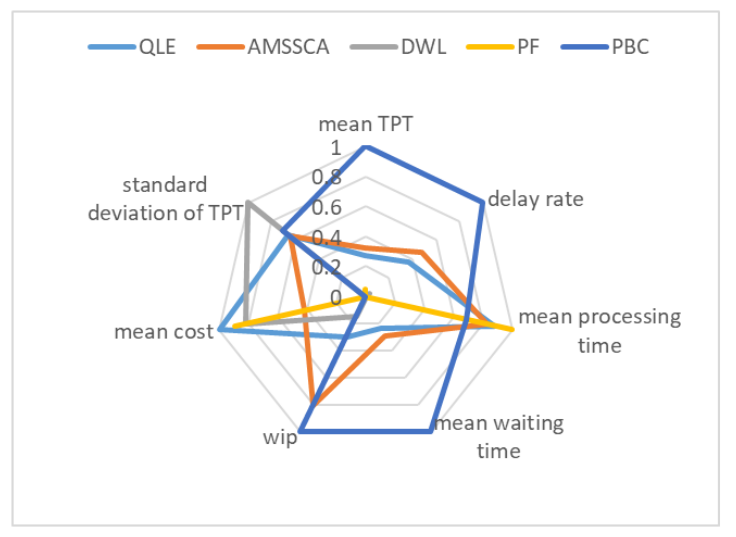
Ziqi Zhao; Oliver Antons; Julia C. Arlinghaus
Autonomous Production Control Methods-Job Shop Simulations Proceedings Article
In: International Conference on Dynamics in Logistics, pp. 227–235, Springer 2020.
Abstract | Links | BibTeX | Tags: Autonomous production control, Industry 4.0, Production planning and control
@inproceedings{zhao2020autonomous,
title = {Autonomous Production Control Methods-Job Shop Simulations},
author = {Ziqi Zhao and Oliver Antons and Julia C. Arlinghaus},
url = {https://link.springer.com/chapter/10.1007/978-3-030-44783-0_22},
doi = {10.1007/978-3-030-44783-0_22},
year = {2020},
date = {2020-01-01},
urldate = {2020-01-01},
booktitle = {International Conference on Dynamics in Logistics},
pages = {227--235},
organization = {Springer},
abstract = {With the development of Industry 4.0 and the Internet of Things, autonomous production control is regarded as a feasible and promising approach for meeting the increasing challenges of complexity and flexibility. To implement autonomous production control methods in the practice, a deeper understanding of their characteristics is necessary. This research provides a comparative perspective on existing methods. We study selected autonomous production control methods under various scenarios, and derive insights for the design of such systems in industrial practice.},
keywords = {Autonomous production control, Industry 4.0, Production planning and control},
pubstate = {published},
tppubtype = {inproceedings}
}