2022
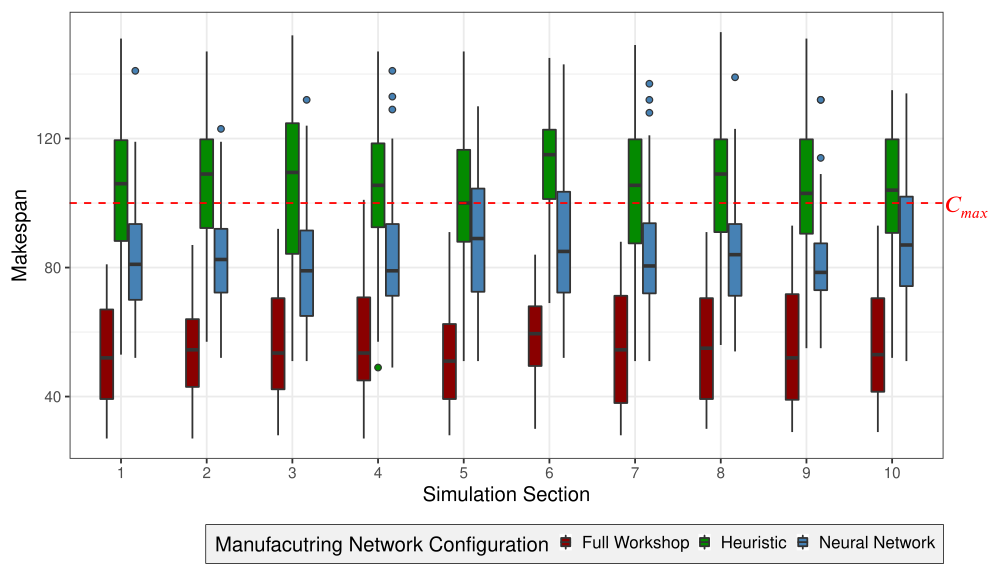
Oliver Antons; Julia C. Arlinghaus
Data-driven and autonomous manufacturing control in cyber-physical production systems Journal Article
In: Computers in Industry, vol. 141, pp. 103711, 2022, ISSN: 0166-3615.
Abstract | Links | BibTeX | Tags: Autonomous production control, Autonomy & Decision-making Authority, Machine learning, Neural network, Production planning and control
@article{ANTONS2022103711,
title = {Data-driven and autonomous manufacturing control in cyber-physical production systems},
author = {Oliver Antons and Julia C. Arlinghaus},
url = {https://www.sciencedirect.com/science/article/pii/S0166361522001087},
doi = {https://doi.org/10.1016/j.compind.2022.103711},
issn = {0166-3615},
year = {2022},
date = {2022-01-01},
urldate = {2022-01-01},
journal = {Computers in Industry},
volume = {141},
pages = {103711},
abstract = {Modern manufacturing networks consist of cyber-physical systems (CPS) which offer an array of interesting capabilities, ranging from local computation over data generation to communication capabilities. As traditional control approaches fail to fully leverage these capabilities, the last decade has seen a renewed interest in distributed control approaches based on autonomous entities. In this article, we study the synergistic potentials of autonomous control and machine learning in a job-shop setting, addressing challenges of modern manufacturing such as market fluctuation and process time variance, thus leveraging the potentials of CPS in order to flexibly configure manufacturing networks and achieve cost-minimal production. We utilize a multi-agent based discrete-event simulation to compare this novel approach to a traditional heuristic, underlining the potentials and advantages of data-driven control approaches.},
keywords = {Autonomous production control, Autonomy \& Decision-making Authority, Machine learning, Neural network, Production planning and control},
pubstate = {published},
tppubtype = {article}
}
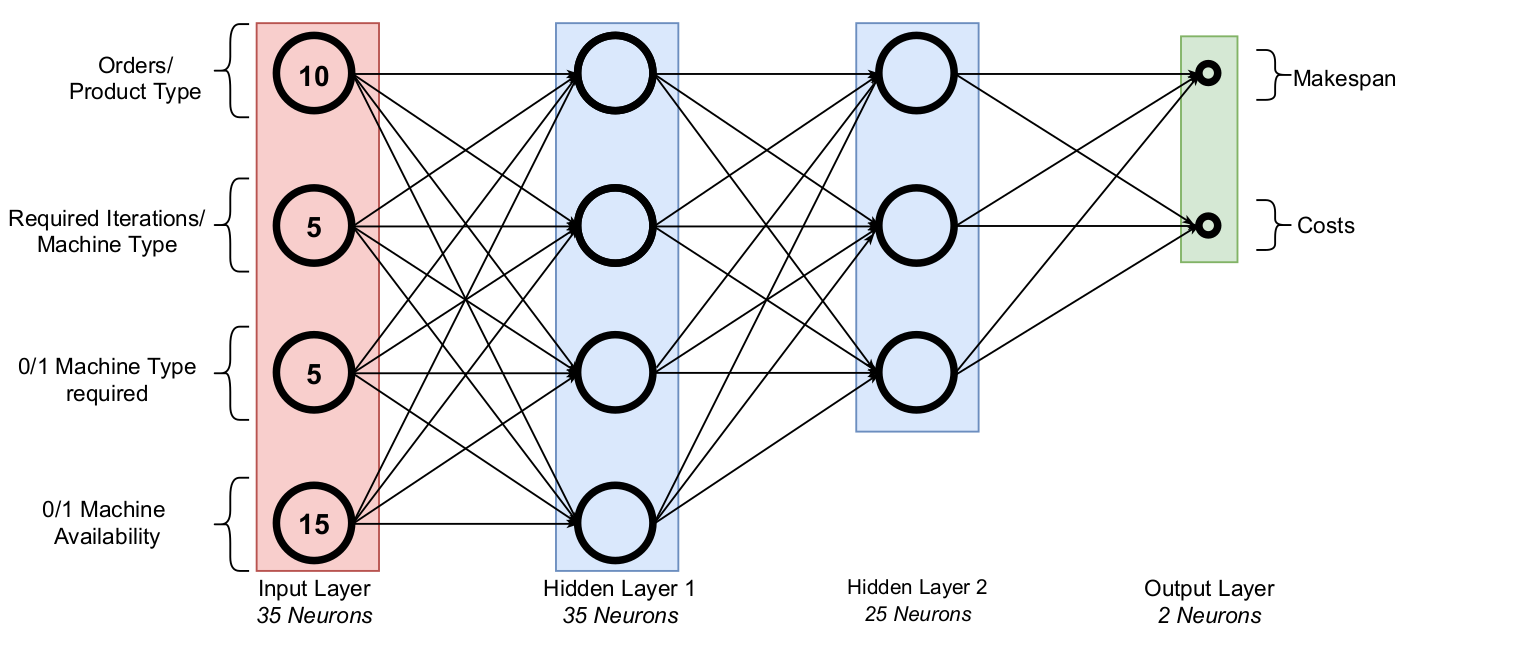
Oliver Antons; Julia C. Arlinghaus
Machine Learning and Autonomous Control—A Synergy for Manufacturing Proceedings Article
In: Borangiu, Theodor; Trentesaux, Damien; Leitão, Paulo; Cardin, Olivier; Joblot, Laurent (Ed.): Service Oriented, Holonic and Multi-agent Manufacturing Systems for Industry of the Future, pp. 417–428, Springer International Publishing, Cham, 2022, ISBN: 978-3-030-99108-1.
Abstract | Links | BibTeX | Tags: Autonomy & Decision-making Authority, Distributed control, Machine learning, Manufacturing, Neural network
@inproceedings{10.1007/978-3-030-99108-1_30,
title = {Machine Learning and Autonomous Control\textemdashA Synergy for Manufacturing},
author = {Oliver Antons and Julia C. Arlinghaus},
editor = {Theodor Borangiu and Damien Trentesaux and Paulo Leit\~{a}o and Olivier Cardin and Laurent Joblot},
url = {https://link.springer.com/chapter/10.1007/978-3-030-99108-1_30},
doi = {10.1007/978-3-030-99108-1_30 },
isbn = {978-3-030-99108-1},
year = {2022},
date = {2022-01-01},
urldate = {2022-01-01},
booktitle = {Service Oriented, Holonic and Multi-agent Manufacturing Systems for Industry of the Future},
pages = {417\textendash428},
publisher = {Springer International Publishing},
address = {Cham},
abstract = {This papers studies the synergistic potentials of machine learning and distributed control approaches in a job-shop setting. We utilize a multi-agent based discrete-event simulation to model distributed control in conjunction with a neural network to predict the optimal workshop configuration given fluctuating production demands. Within this simulation model, we study the potential cost and time savings, showing various potentials in the synergistic utilization of distributed control and machine learning for production planning and control in a job-shop manufacturing network.},
keywords = {Autonomy \& Decision-making Authority, Distributed control, Machine learning, Manufacturing, Neural network},
pubstate = {published},
tppubtype = {inproceedings}
}