2022
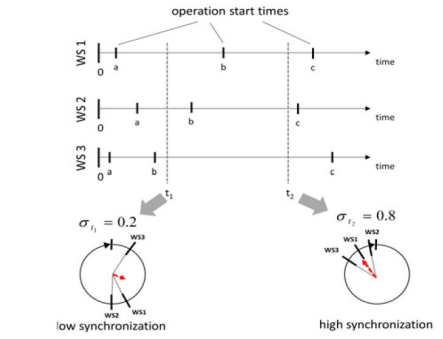
Florian Knapp; Oliver Antons; Julia C. Arlinghaus
Opportunities for Synchronization in Manufacturing as Key Performance Indicator Proceedings Article
In: pp. 1467-1472, 2022, ISSN: 2212-8271, (Leading manufacturing systems transformation – Proceedings of the 55th CIRP Conference on Manufacturing Systems 2022).
Abstract | Links | BibTeX | Tags: Control, Key Performance Indicators, Manufacturing, Manufacturing Networks, Production Planning, Synchronization
@inproceedings{KNAPP20221467,
title = {Opportunities for Synchronization in Manufacturing as Key Performance Indicator},
author = {Florian Knapp and Oliver Antons and Julia C. Arlinghaus},
url = {https://www.sciencedirect.com/science/article/pii/S2212827122004607},
doi = {https://doi.org/10.1016/j.procir.2022.05.176},
issn = {2212-8271},
year = {2022},
date = {2022-01-01},
urldate = {2022-01-01},
journal = {Procedia CIRP},
volume = {107},
pages = {1467-1472},
abstract = {In the complex and dynamic conditions of global markets it is increasingly challenging for manufacturing companies to find or identify new reliable and meaningful key performance indicators. Traditional performance indicators like lead time, inventory level, on-time delivery rate and capacity utilization are still in focus, but interdisciplinary research suggests the inclusion of more innovative performance indicators, such as synchronization. The synchronization level of a manufacturing system can be viewed twofold: logistic and physical synchronization. Physical synchronization is defined as "the rhythm and repetitive behaviour of production processes", while logistical synchronization describes "the coupling of work systems that are linked by material flows." Both types of synchronization correlate with the logistics performance of companies. Previous research on this topic has already provided first insights, highlighting potentials and fields of application. In this paper, we provide a structured literature review underlining relevant applications and explore their respective potentials. Thus, we show how synchronization effects can be exploited, and which systemic properties, such as network topology, characteristics and process time variation influence the occurrence of synchronization.},
note = {Leading manufacturing systems transformation \textendash Proceedings of the 55th CIRP Conference on Manufacturing Systems 2022},
keywords = {Control, Key Performance Indicators, Manufacturing, Manufacturing Networks, Production Planning, Synchronization},
pubstate = {published},
tppubtype = {inproceedings}
}
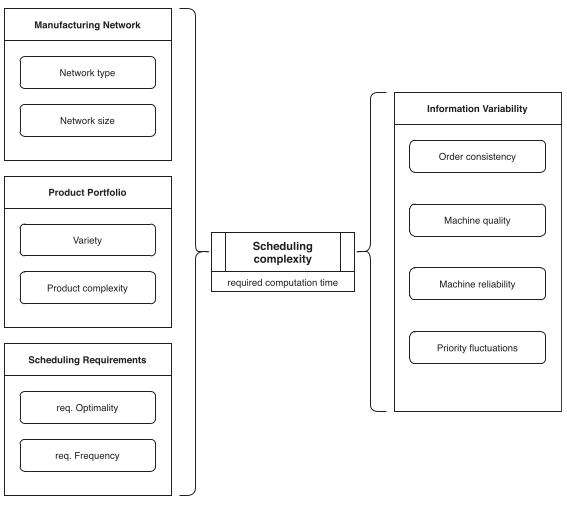
Oliver Antons; Julia C. Arlinghaus
A Manufacturing Scheduling Complexity Framework and Agent-Based Comparison of Centralized and Distributed Control Approaches Journal Article
In: IEEE Journal of Emerging and Selected Topics in Industrial Electronics, vol. 3, no. 1, pp. 31-38, 2022.
Abstract | Links | BibTeX | Tags: Autonomy & Decision-making Authority, Complexity Theory, Decentralized Control, Job-shop scheduling, Manufacturing, Optimization, Production
@article{antons2021ieee,
title = {A Manufacturing Scheduling Complexity Framework and Agent-Based Comparison of Centralized and Distributed Control Approaches},
author = {Oliver Antons and Julia C. Arlinghaus},
url = {https://doi.org/10.1109/JESTIE.2021.3100272},
doi = {10.1109/JESTIE.2021.3100272},
year = {2022},
date = {2022-01-01},
urldate = {2022-01-01},
journal = {IEEE Journal of Emerging and Selected Topics in Industrial Electronics},
volume = {3},
number = {1},
pages = {31-38},
abstract = {Centralized approaches are often employed to control manufacturing networks in practice. The introduction of industrial cyber-physical systems driven by advances in microcontroller, sensor, and networking technologies is providing distributed control systems with the technical requirements needed to mitigate the drawbacks of centralized control, such as long optimization times that result in long planning horizons and inflexibility. While such distributed control approaches respond to the growing challenges faced by industry in terms of flexibility, resilience, and lot sizes, the inherent myopia of autonomous agents may discourage practical application. In this article, we develop a scheduling complexity framework derived from the literature, which allows researchers and prationers alike to evaluate the suitability of both centralized and distributed control approaches for manufacturing planning and control. This framework utilizes quantifiable environment variables, which influence we study by means of a multiagent discrete event simulation.},
keywords = {Autonomy \& Decision-making Authority, Complexity Theory, Decentralized Control, Job-shop scheduling, Manufacturing, Optimization, Production},
pubstate = {published},
tppubtype = {article}
}
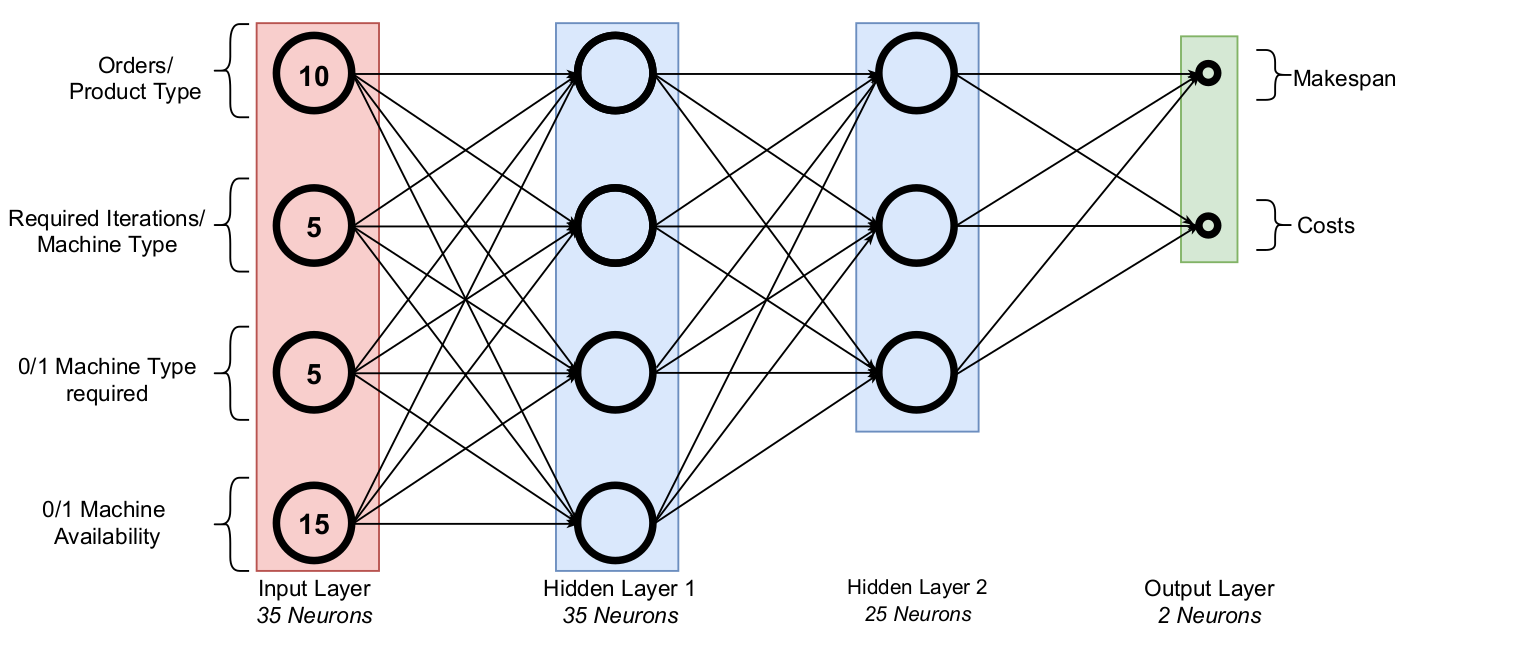
Oliver Antons; Julia C. Arlinghaus
Machine Learning and Autonomous Control—A Synergy for Manufacturing Proceedings Article
In: Borangiu, Theodor; Trentesaux, Damien; Leitão, Paulo; Cardin, Olivier; Joblot, Laurent (Ed.): Service Oriented, Holonic and Multi-agent Manufacturing Systems for Industry of the Future, pp. 417–428, Springer International Publishing, Cham, 2022, ISBN: 978-3-030-99108-1.
Abstract | Links | BibTeX | Tags: Autonomy & Decision-making Authority, Distributed control, Machine learning, Manufacturing, Neural network
@inproceedings{10.1007/978-3-030-99108-1_30,
title = {Machine Learning and Autonomous Control\textemdashA Synergy for Manufacturing},
author = {Oliver Antons and Julia C. Arlinghaus},
editor = {Theodor Borangiu and Damien Trentesaux and Paulo Leit\~{a}o and Olivier Cardin and Laurent Joblot},
url = {https://link.springer.com/chapter/10.1007/978-3-030-99108-1_30},
doi = {10.1007/978-3-030-99108-1_30 },
isbn = {978-3-030-99108-1},
year = {2022},
date = {2022-01-01},
urldate = {2022-01-01},
booktitle = {Service Oriented, Holonic and Multi-agent Manufacturing Systems for Industry of the Future},
pages = {417\textendash428},
publisher = {Springer International Publishing},
address = {Cham},
abstract = {This papers studies the synergistic potentials of machine learning and distributed control approaches in a job-shop setting. We utilize a multi-agent based discrete-event simulation to model distributed control in conjunction with a neural network to predict the optimal workshop configuration given fluctuating production demands. Within this simulation model, we study the potential cost and time savings, showing various potentials in the synergistic utilization of distributed control and machine learning for production planning and control in a job-shop manufacturing network.},
keywords = {Autonomy \& Decision-making Authority, Distributed control, Machine learning, Manufacturing, Neural network},
pubstate = {published},
tppubtype = {inproceedings}
}