2021
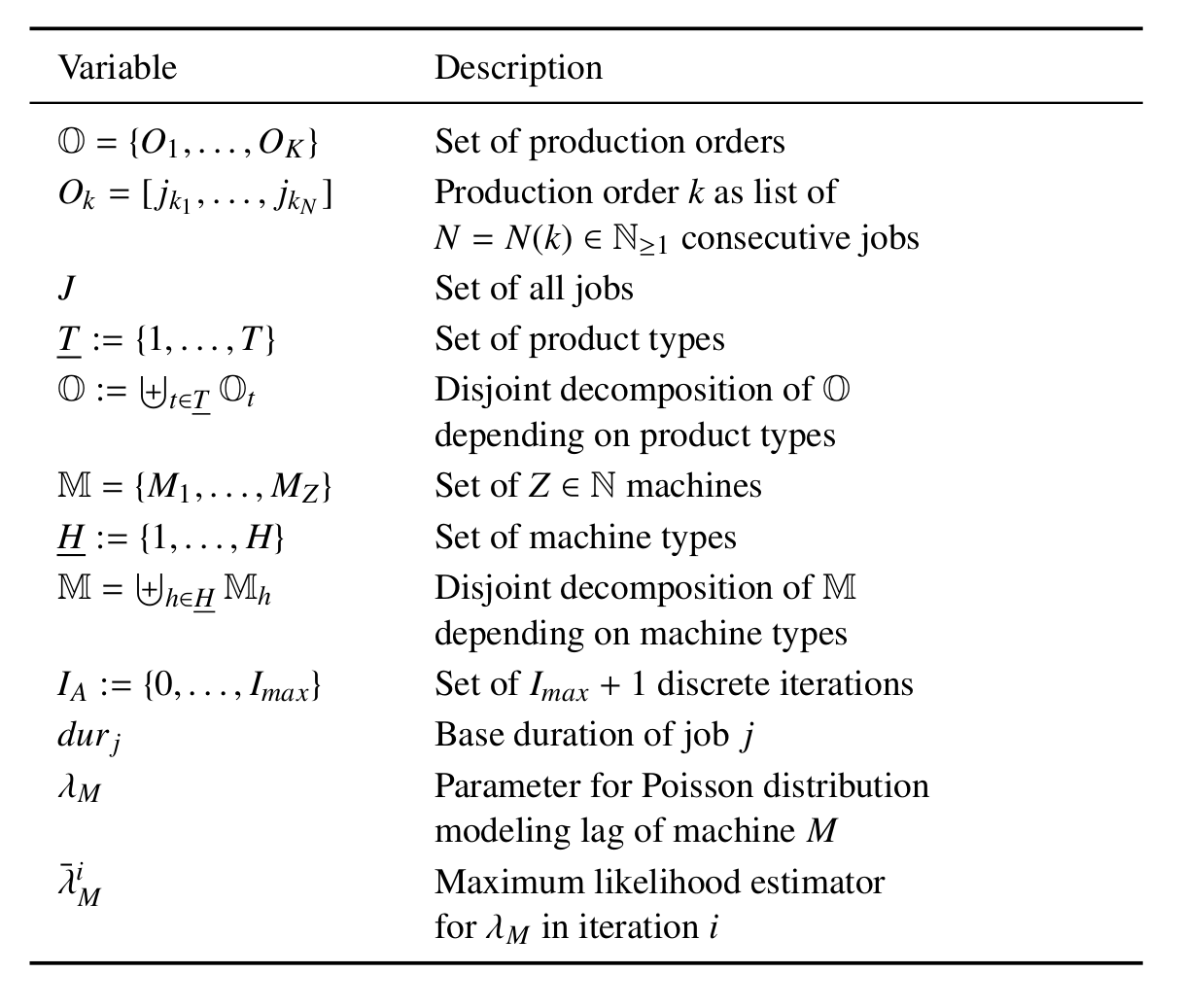
Oliver Antons; Julia C. Arlinghaus
Adaptive self-learning distributed and centralized control approaches for smart factories Proceedings Article
In: pp. 1577-1582, 2021, ISSN: 2212-8271, (54th CIRP CMS 2021 - Towards Digitalized Manufacturing 4.0).
Abstract | Links | BibTeX | Tags: Autonomy, Autonomy & Decision-making Authority, Cyber-physical system, Data analytics, Decision-making, Discrete-event simulation, Distributed control, Industry 4.0, Multi-agent system, Self-learning, Smart factory
@inproceedings{ANTONS20211577,
title = {Adaptive self-learning distributed and centralized control approaches for smart factories},
author = {Oliver Antons and Julia C. Arlinghaus},
url = {https://www.sciencedirect.com/science/article/pii/S2212827121011641},
doi = {https://doi.org/10.1016/j.procir.2021.11.266},
issn = {2212-8271},
year = {2021},
date = {2021-01-01},
urldate = {2021-01-01},
journal = {Procedia CIRP},
volume = {104},
pages = {1577-1582},
abstract = {The increasing application of cyber-physical systems creates a manufacturing environment in which the technical requirements for distributed control approaches, self-learning systems and analytics of previously untapped data are given. While distributed control approaches are capable to evaluate this information locally and react immediately, centralized approaches react inertly to analyzed machine performance data. In this paper, we study the performance and ability to address the ever increasing challenges in industry of both types of control approaches within an established multi-agent based discrete event simulation.},
note = {54th CIRP CMS 2021 - Towards Digitalized Manufacturing 4.0},
keywords = {Autonomy, Autonomy \& Decision-making Authority, Cyber-physical system, Data analytics, Decision-making, Discrete-event simulation, Distributed control, Industry 4.0, Multi-agent system, Self-learning, Smart factory},
pubstate = {published},
tppubtype = {inproceedings}
}
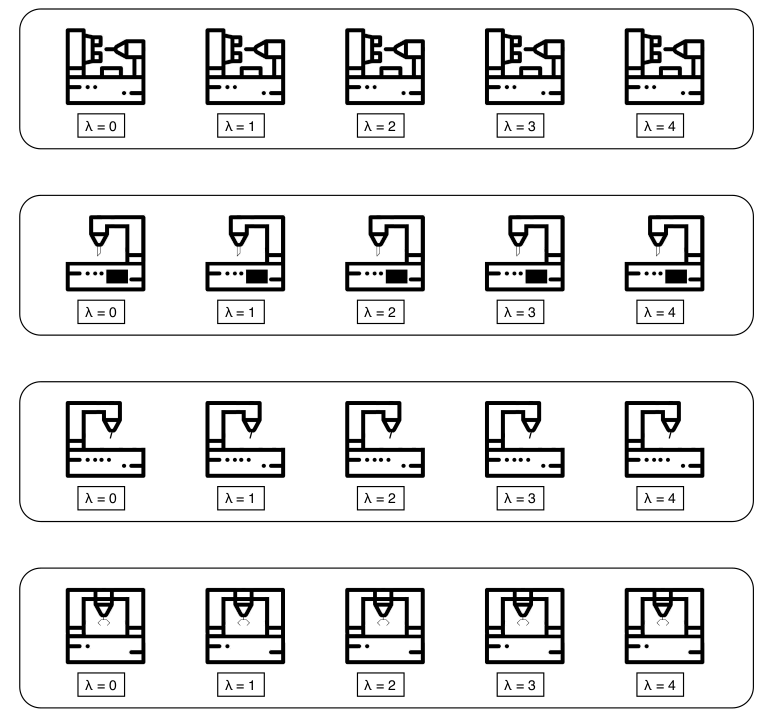
Oliver Antons; Julia C. Arlinghaus
Learning Distributed Control for Job Shops-A Comparative Simulation Study Proceedings Article
In: Service Oriented, Holonic and Multi-Agent Manufacturing Systems for Industry of the Future: Proceedings of SOHOMA 2020, pp. 193–202, Springer International Publishing 2021.
Abstract | Links | BibTeX | Tags: Autonomy & Decision-making Authority, Discrete-event simulation, Job-shop scheduling, Multi-agent system, Production planning and control, Self-learning
@inproceedings{antons2021learning,
title = {Learning Distributed Control for Job Shops-A Comparative Simulation Study},
author = {Oliver Antons and Julia C. Arlinghaus},
url = {https://link.springer.com/book/10.1007/978-3-030-69373-2},
doi = {10.1007/978-3-030-69373-2},
year = {2021},
date = {2021-01-01},
urldate = {2021-01-01},
booktitle = {Service Oriented, Holonic and Multi-Agent Manufacturing Systems for Industry of the Future: Proceedings of SOHOMA 2020},
pages = {193--202},
organization = {Springer International Publishing},
abstract = {This paper studies the potentials of learning and benefits of local data processing in a distributed control setting. We deploy a multi-agent system in the context of a discrete-event simulation to model distributed control for a job shop manufacturing system with variable processing times and multi-stage production processes. Within this simulation, we compare queue length estimation as dispatching rule against a variation with learning capability, which processes additional historic data on a machine agent level, showing the potentials of learning and coordination for distributed control in PPC.},
keywords = {Autonomy \& Decision-making Authority, Discrete-event simulation, Job-shop scheduling, Multi-agent system, Production planning and control, Self-learning},
pubstate = {published},
tppubtype = {inproceedings}
}