2023
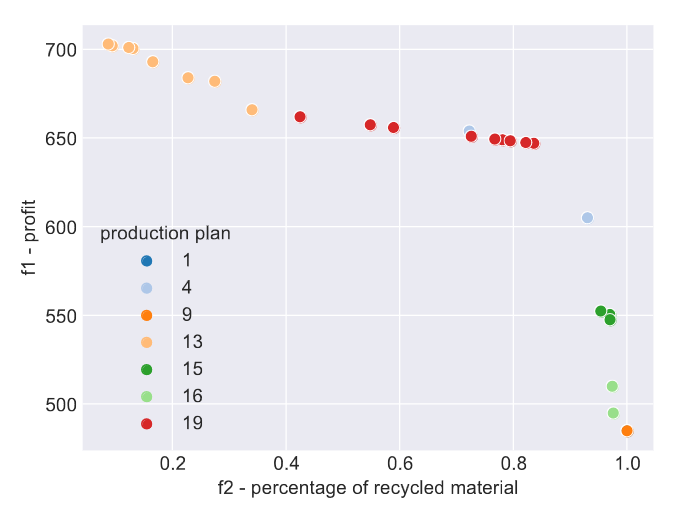
Tobias Benecke; Oliver Antons; Sanaz Mostaghim; Julia C. Arlinghaus
A Coevolution Approach for the Multi-objective Circular Supply Chain Problem Proceedings Article
In: 2023 IEEE Conference on Artificial Intelligence (CAI), pp. 222-223, 2023.
Abstract | Links | BibTeX | Tags: Complexity Theory, Costs, Production Planning, Profitability, Supply chain optimization
@inproceedings{10195126,
title = {A Coevolution Approach for the Multi-objective Circular Supply Chain Problem},
author = {Tobias Benecke and Oliver Antons and Sanaz Mostaghim and Julia C. Arlinghaus},
doi = {10.1109/CAI54212.2023.00103},
year = {2023},
date = {2023-01-01},
urldate = {2023-01-01},
booktitle = {2023 IEEE Conference on Artificial Intelligence (CAI)},
pages = {222-223},
abstract = {As a more sustainable resource use is becoming of greater concern, moving towards a more circular economy seems promising. However, compared to the traditional, mostly linear production processes, this introduces new challenges, as reintroducing recycled materials into production also increases supply chain complexity and therefore cost. The circular supply chain (CSC) problem is modeling these challenges to find good tradeoff solutions between profitability and sustainable resource use. The optimization concerns the production planning and material sourcing of a production plant. This is a complex task due to their inherent dependencies. In this paper, we use a cooperative coevolutionary approach to optimize the CSC problem, by decomposing it to resolve the variable dependencies. Besides presenting the algorithm, a proof of concept evaluation is done to show its feasibility.},
keywords = {Complexity Theory, Costs, Production Planning, Profitability, Supply chain optimization},
pubstate = {published},
tppubtype = {inproceedings}
}
2022
Konstantin Büttner; Oliver Antons; Julia C. Arlinghaus
Applied Machine Learning for Production Planning and Control: Overview and Potentials Proceedings Article
In: 10th IFAC Conference on Manufacturing Modelling, Management and Control, pp. 6, Elsevier, 2022.
Abstract | Links | BibTeX | Tags: Control, Machine learning, Production control, Production Planning
@inproceedings{Buettner2022,
title = {Applied Machine Learning for Production Planning and Control: Overview and Potentials},
author = {Konstantin B\"{u}ttner and Oliver Antons and Julia C. Arlinghaus},
url = {https://www.sciencedirect.com/science/article/pii/S2405896322021152},
doi = {https://doi.org/10.1016/j.ifacol.2022.10.106},
year = {2022},
date = {2022-10-01},
urldate = {2022-10-01},
booktitle = {10th IFAC Conference on Manufacturing Modelling, Management and Control},
volume = {55},
number = {10},
pages = {6},
publisher = {Elsevier},
abstract = {Manufacturing companies are under constant pressure to increase efficiency and to achieve logistical objectives. Improving production planning and control (PPC) has significant impact on these efforts. At the same time, increasing complexity and dynamics of PPC environments make PPC more difficult. One way to cope with this situation is the application of machine learning (ML) methods. In this article, we therefore address the current state of PPC-ML research and show, based on the Aachen PPC model, in which PPC tasks and subtasks ML is already applied and to what degree the task is covered by ML. The analysis is limited to core and cross-sectional tasks of the Aachen PPC model, procurement and network tasks are not included. Furthermore, a broad analysis of the targeted data mining, business and logistic objectives is conducted. In addition, we also identify motivations which prompted researchers to apply ML in PPC. },
keywords = {Control, Machine learning, Production control, Production Planning},
pubstate = {published},
tppubtype = {inproceedings}
}
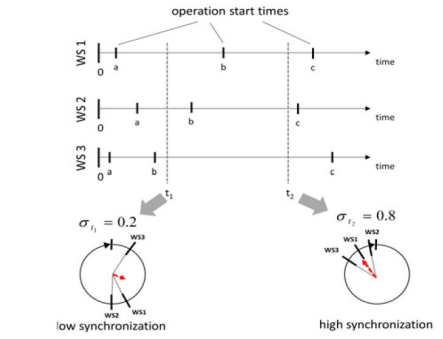
Florian Knapp; Oliver Antons; Julia C. Arlinghaus
Opportunities for Synchronization in Manufacturing as Key Performance Indicator Proceedings Article
In: pp. 1467-1472, 2022, ISSN: 2212-8271, (Leading manufacturing systems transformation – Proceedings of the 55th CIRP Conference on Manufacturing Systems 2022).
Abstract | Links | BibTeX | Tags: Control, Key Performance Indicators, Manufacturing, Manufacturing Networks, Production Planning, Synchronization
@inproceedings{KNAPP20221467,
title = {Opportunities for Synchronization in Manufacturing as Key Performance Indicator},
author = {Florian Knapp and Oliver Antons and Julia C. Arlinghaus},
url = {https://www.sciencedirect.com/science/article/pii/S2212827122004607},
doi = {https://doi.org/10.1016/j.procir.2022.05.176},
issn = {2212-8271},
year = {2022},
date = {2022-01-01},
urldate = {2022-01-01},
journal = {Procedia CIRP},
volume = {107},
pages = {1467-1472},
abstract = {In the complex and dynamic conditions of global markets it is increasingly challenging for manufacturing companies to find or identify new reliable and meaningful key performance indicators. Traditional performance indicators like lead time, inventory level, on-time delivery rate and capacity utilization are still in focus, but interdisciplinary research suggests the inclusion of more innovative performance indicators, such as synchronization. The synchronization level of a manufacturing system can be viewed twofold: logistic and physical synchronization. Physical synchronization is defined as "the rhythm and repetitive behaviour of production processes", while logistical synchronization describes "the coupling of work systems that are linked by material flows." Both types of synchronization correlate with the logistics performance of companies. Previous research on this topic has already provided first insights, highlighting potentials and fields of application. In this paper, we provide a structured literature review underlining relevant applications and explore their respective potentials. Thus, we show how synchronization effects can be exploited, and which systemic properties, such as network topology, characteristics and process time variation influence the occurrence of synchronization.},
note = {Leading manufacturing systems transformation \textendash Proceedings of the 55th CIRP Conference on Manufacturing Systems 2022},
keywords = {Control, Key Performance Indicators, Manufacturing, Manufacturing Networks, Production Planning, Synchronization},
pubstate = {published},
tppubtype = {inproceedings}
}