2023
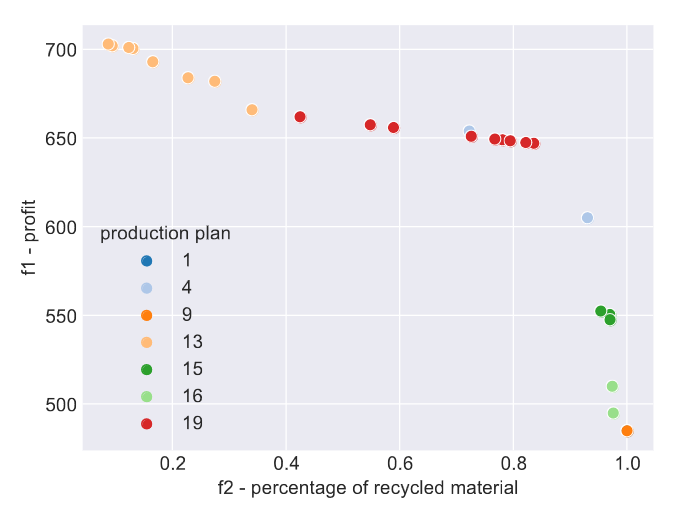
Tobias Benecke; Oliver Antons; Sanaz Mostaghim; Julia C. Arlinghaus
A Coevolution Approach for the Multi-objective Circular Supply Chain Problem Proceedings Article
In: 2023 IEEE Conference on Artificial Intelligence (CAI), pp. 222-223, 2023.
Abstract | Links | BibTeX | Tags: Complexity Theory, Costs, Production Planning, Profitability, Supply chain optimization
@inproceedings{10195126,
title = {A Coevolution Approach for the Multi-objective Circular Supply Chain Problem},
author = {Tobias Benecke and Oliver Antons and Sanaz Mostaghim and Julia C. Arlinghaus},
doi = {10.1109/CAI54212.2023.00103},
year = {2023},
date = {2023-01-01},
urldate = {2023-01-01},
booktitle = {2023 IEEE Conference on Artificial Intelligence (CAI)},
pages = {222-223},
abstract = {As a more sustainable resource use is becoming of greater concern, moving towards a more circular economy seems promising. However, compared to the traditional, mostly linear production processes, this introduces new challenges, as reintroducing recycled materials into production also increases supply chain complexity and therefore cost. The circular supply chain (CSC) problem is modeling these challenges to find good tradeoff solutions between profitability and sustainable resource use. The optimization concerns the production planning and material sourcing of a production plant. This is a complex task due to their inherent dependencies. In this paper, we use a cooperative coevolutionary approach to optimize the CSC problem, by decomposing it to resolve the variable dependencies. Besides presenting the algorithm, a proof of concept evaluation is done to show its feasibility.},
keywords = {Complexity Theory, Costs, Production Planning, Profitability, Supply chain optimization},
pubstate = {published},
tppubtype = {inproceedings}
}
2022
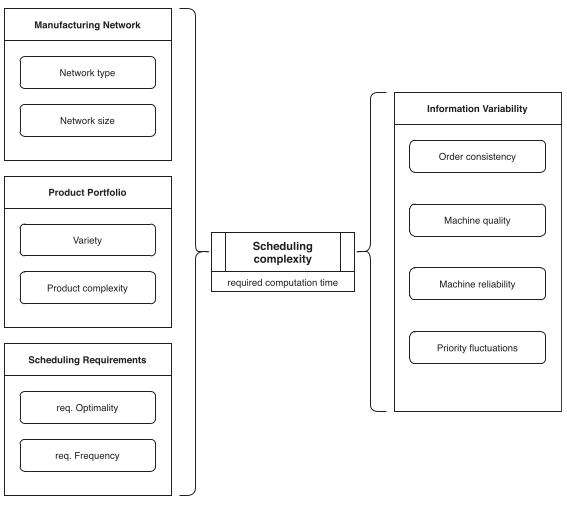
Oliver Antons; Julia C. Arlinghaus
A Manufacturing Scheduling Complexity Framework and Agent-Based Comparison of Centralized and Distributed Control Approaches Journal Article
In: IEEE Journal of Emerging and Selected Topics in Industrial Electronics, vol. 3, no. 1, pp. 31-38, 2022.
Abstract | Links | BibTeX | Tags: Autonomy & Decision-making Authority, Complexity Theory, Decentralized Control, Job-shop scheduling, Manufacturing, Optimization, Production
@article{antons2021ieee,
title = {A Manufacturing Scheduling Complexity Framework and Agent-Based Comparison of Centralized and Distributed Control Approaches},
author = {Oliver Antons and Julia C. Arlinghaus},
url = {https://doi.org/10.1109/JESTIE.2021.3100272},
doi = {10.1109/JESTIE.2021.3100272},
year = {2022},
date = {2022-01-01},
urldate = {2022-01-01},
journal = {IEEE Journal of Emerging and Selected Topics in Industrial Electronics},
volume = {3},
number = {1},
pages = {31-38},
abstract = {Centralized approaches are often employed to control manufacturing networks in practice. The introduction of industrial cyber-physical systems driven by advances in microcontroller, sensor, and networking technologies is providing distributed control systems with the technical requirements needed to mitigate the drawbacks of centralized control, such as long optimization times that result in long planning horizons and inflexibility. While such distributed control approaches respond to the growing challenges faced by industry in terms of flexibility, resilience, and lot sizes, the inherent myopia of autonomous agents may discourage practical application. In this article, we develop a scheduling complexity framework derived from the literature, which allows researchers and prationers alike to evaluate the suitability of both centralized and distributed control approaches for manufacturing planning and control. This framework utilizes quantifiable environment variables, which influence we study by means of a multiagent discrete event simulation.},
keywords = {Autonomy \& Decision-making Authority, Complexity Theory, Decentralized Control, Job-shop scheduling, Manufacturing, Optimization, Production},
pubstate = {published},
tppubtype = {article}
}