2024
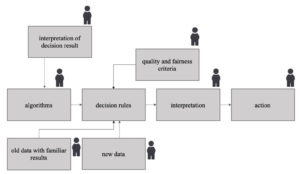
Melanie Kessler; Oliver Antons; Julia C. Arlinghaus
Designing hybrid intelligence: understanding the impact of human decision-making on AI Best Paper Konferenz
Human Aspects of Advanced Manufacturing, Production Management and Process Control. AHFE, Bd. 146, AHFE International, USA, 2024.
Abstract | Links | BibTeX | Schlagwörter: Articifial Intelligence, Human Decision-making, Hybrid Intelligence
@conference{nokey,
title = {Designing hybrid intelligence: understanding the impact of human decision-making on AI},
author = {Melanie Kessler and Oliver Antons and Julia C. Arlinghaus },
editor = {Beata Mrugalska, Waldemar Karwowski and Stefan Trzcielinski},
url = {https://oliver.antons.eu/best-paper-award-ahfe-2024-conference/, Best Paper Award
http://doi.org/10.54941/ahfe1005148},
doi = {10.54941/ahfe1005148},
year = {2024},
date = {2024-07-01},
urldate = {2024-07-01},
booktitle = {Human Aspects of Advanced Manufacturing, Production Management and Process Control. AHFE},
volume = {146},
pages = {31-39},
address = {AHFE International, USA},
abstract = {In many domains such as management, production and government, established control approaches struggle to address increasing complexity in a timely manner, resulting in a demand for more agile methods. Hybrid intelligence and decision support systems are useful approaches to augment human decision-making through artificial intelligence (AI). Various application of AI methods to estimate production parameters or to provide forecasts are discussed in the literature or already being implemented, however, human decision-making is still required for either deciding whether to follow specific suggestions or for monitoring their respective implementation. But human behavioral research has shown that human decision-making is rather biased than fully rational, leading to unintended consequences in the collaborative work of humans and machines. Subsequently, the research stream of hybrid intelligence has gained interest recently, aiming to study the collaboration between humans and machines. We contribute to this issue by combining a systematic literature review on AI and cognitive biases combined with practical insights from discussions with experts in order to derive first guidelines addressing the human factor in the design of AI-based decision support systems for complex production environments.},
keywords = {Articifial Intelligence, Human Decision-making, Hybrid Intelligence},
pubstate = {published},
tppubtype = {conference}
}
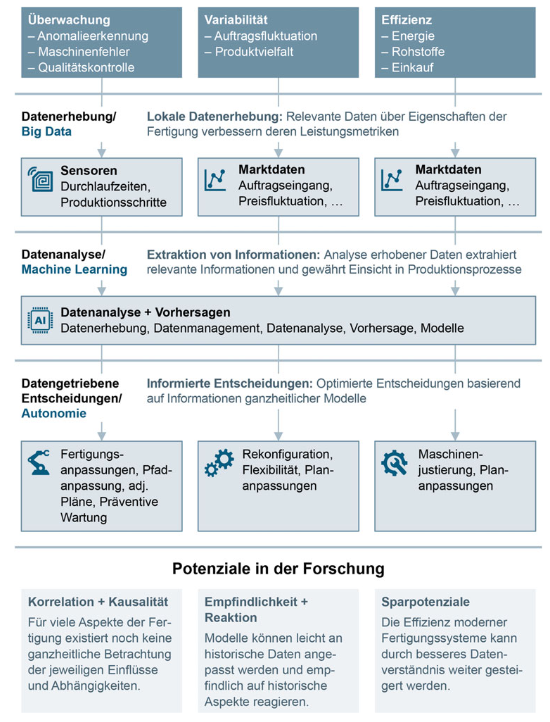
Julia C. Arlinghaus; Oliver Antons
Planung und Steuerung für die digitale Produktion Buchkapitel
In: Berlin Springer Vieweg, Heidelberg (Hrsg.): Handbuch Unternehmensorganisation, Springer Vieweg, Berlin, Heidelberg, 2024, ISBN: 978-3-642-45370-0.
Abstract | Links | BibTeX | Schlagwörter: Autonomous production control, Cyber-physical system, Digitalization, Industry 4.0, Production planning and control, Smart manufacutring systems
@inbook{Arlinghaus2024,
title = {Planung und Steuerung f\"{u}r die digitale Produktion},
author = {Julia C. Arlinghaus and Oliver Antons},
editor = {Springer Vieweg, Berlin, Heidelberg},
url = {https://link.springer.com/referenceworkentry/10.1007/978-3-642-45370-0_63-2},
doi = {10.1007/978-3-642-45370-0_63-2},
isbn = {978-3-642-45370-0},
year = {2024},
date = {2024-07-01},
urldate = {2024-07-01},
booktitle = {Handbuch Unternehmensorganisation},
publisher = {Springer Vieweg, Berlin, Heidelberg},
abstract = {Im vergangenen Jahrzehnt wurde die produzierende Industrie mit einem zunehmend volatileren Umfeld konfrontiert. Unterschiedlichste Krisen haben etablierte Lieferketten ersch\"{u}ttert und die Notwendigkeit resilienter und flexibler Produktionsplanung und -steuerung aufgezeigt. Zeitgleich hat eine voranschreitende Digitalisierung der Produktionsanlagen neue Herausforderungen, Potenziale und Chancen aufgeworfen. Cyber-physikalische Systeme und ein Industrial Internet of Things erm\"{o}glichen Digitale Zwillinge der Produktion und erf\"{u}llen die technischen Voraussetzungen f\"{u}r eine autonome Entscheidungsfindung auf einzelnen Produktionssystemen. In diesem Kontext stellt sich f\"{u}r Unternehmen eine fundamentale Frage der Organisation hinsichtlich der Architektur von Produktionsplanung und -steuerung. Mit zentralisierter sowie verteilter Produktionsplanung und -steuerung stehen Unternehmen zwei gegenl\"{a}ufige Ans\"{a}tze zur Verf\"{u}gung, die in diesem Beitrag n\"{a}her betrachtet werden.},
keywords = {Autonomous production control, Cyber-physical system, Digitalization, Industry 4.0, Production planning and control, Smart manufacutring systems},
pubstate = {published},
tppubtype = {inbook}
}
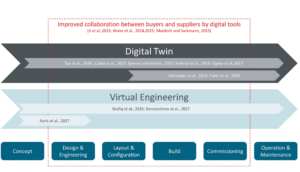
Luca Janecki; Oliver Antons; Daniel Reh; Julia C. Arlinghaus
Comparing Digital Twins and Virtual Engineering in Buyer Supplier Relationships for Complex Production Facilities Proceedings Article
In: Thürer, Matthias; Riedel, Ralph; Cieminski, Gregor; Romero, David (Hrsg.): Advances in Production Management Systems. Production Management Systems for Volatile, Uncertain, Complex, and Ambiguous Environments, S. 180–193, Springer Nature Switzerland, Cham, 2024, ISBN: 978-3-031-71645-4.
Abstract | Links | BibTeX | Schlagwörter: Buyer-Supplier Relationship, Digital Twin, Production, Virtual Engineering
@inproceedings{10.1007/978-3-031-71645-4_13,
title = {Comparing Digital Twins and Virtual Engineering in Buyer Supplier Relationships for Complex Production Facilities},
author = {Luca Janecki and Oliver Antons and Daniel Reh and Julia C. Arlinghaus},
editor = {Matthias Th\"{u}rer and Ralph Riedel and Gregor Cieminski and David Romero},
url = {https://link.springer.com/chapter/10.1007/978-3-031-71645-4_13},
doi = {10.1007/978-3-031-71645-4_13},
isbn = {978-3-031-71645-4},
year = {2024},
date = {2024-01-01},
urldate = {2024-01-01},
booktitle = {Advances in Production Management Systems. Production Management Systems for Volatile, Uncertain, Complex, and Ambiguous Environments},
pages = {180\textendash193},
publisher = {Springer Nature Switzerland},
address = {Cham},
abstract = {In today's highly competitive and interconnected global marketplace, the effective management of buyer-supplier relationships is essential for organizations seeking to stay ahead. With the rise of complex production facilities, ensuring product quality and optimizing communication between buyers and suppliers presents an escalating challenge. This complexity is further compounded by the integration of Digital Twins and Virtual Engineering, requiring innovative solutions to navigate the intricacies of modern supply chain dynamics. This article explores the role of Digital Twins and Virtual Engineering as a strategic approach to enhance buyer-supplier relationship management within the context of complex production facilities using insights from the Principal Agent Theory. Based on a systematic literature review, we explore current approaches to the use of virtual engineering and digital twins to overcome existing tensions from principal-agent theory.},
keywords = {Buyer-Supplier Relationship, Digital Twin, Production, Virtual Engineering},
pubstate = {published},
tppubtype = {inproceedings}
}
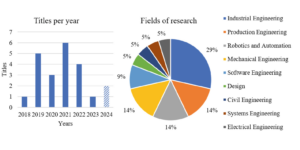
Adrian Schröder; Oliver Antons; Julia C. Arlinghaus
A Literature Review on the Cross-Domain Usage of Digital Factory Twins Within Design Time Proceedings Article
In: Thürer, Matthias; Riedel, Ralph; Cieminski, Gregor; Romero, David (Hrsg.): Advances in Production Management Systems. Production Management Systems for Volatile, Uncertain, Complex, and Ambiguous Environments, S. 315–329, Springer Nature Switzerland, Cham, 2024, ISBN: 978-3-031-71629-4.
Abstract | Links | BibTeX | Schlagwörter: Digital Twin
@inproceedings{10.1007/978-3-031-71629-4_22,
title = {A Literature Review on the Cross-Domain Usage of Digital Factory Twins Within Design Time},
author = {Adrian Schr\"{o}der and Oliver Antons and Julia C. Arlinghaus},
editor = {Matthias Th\"{u}rer and Ralph Riedel and Gregor Cieminski and David Romero},
url = {https://link.springer.com/chapter/10.1007/978-3-031-71629-4_22},
doi = {10.1007/978-3-031-71629-4_22},
isbn = {978-3-031-71629-4},
year = {2024},
date = {2024-01-01},
urldate = {2024-01-01},
booktitle = {Advances in Production Management Systems. Production Management Systems for Volatile, Uncertain, Complex, and Ambiguous Environments},
pages = {315\textendash329},
publisher = {Springer Nature Switzerland},
address = {Cham},
abstract = {In a globalized production environment companies are confronted with shortening product life cycles leading to shortening factory system life cycles. To overcome this issue, factories have to be (re-)built faster. The design time is a crucial phase in which many different disciplines of different domains have to work together in a major planning project. Currently, various experts in the respective domains of e.g. production system planning, automation and building are commonly working on their silo models resulting in different and sometimes contradictory information depending on the perspective of planning. While model-orientated, collaborative planning approaches like Building Information Modeling (BIM) have become familiar with the domain of factory planning, there is still a lack of combining the different factory data models holistically to connect all elements of production regarding information of products, processes and resources. Besides the BIM methodology several other forms of virtual factory descriptions, like the digital factory twin have emerged. In this work, a systematic literature review is conducted to present the current perspective on creating factory data models about a cross-domain usage and modeling approach. In analyzing the current use case definition of factory models the opportunity is seen to point out the importance of the combination of holistically linked factory data models with a multipurpose design. In doing so, a possibility is seen to overcome the mentioned obstacles of planning while raising the value of the created models. This demonstrates the need for a concept of modeling a digital factory twin, created for cross-domain usage.},
keywords = {Digital Twin},
pubstate = {published},
tppubtype = {inproceedings}
}
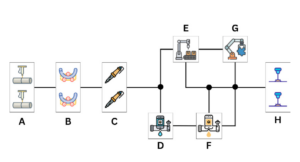
Andrea Somma; Oliver Antons; Alberto Petrillo; Stefania Santini; Teresa Murino
In: IFAC-PapersOnLine, Bd. 58, Nr. 19, S. 217-222, 2024, ISSN: 2405-8963, (18th IFAC Symposium on Information Control Problems in Manufacturing INCOM 2024).
Abstract | Links | BibTeX | Schlagwörter: Control, Digital-Model-Based Simulation, Distributed control, Multi-Job Shop Scheduling, Smart Manufacturing Planning
@article{SOMMA2024217,
title = {On the Verification of Distributed Control for Multi Job Shop Assignment Problem in Smart Manufacturing System},
author = {Andrea Somma and Oliver Antons and Alberto Petrillo and Stefania Santini and Teresa Murino},
url = {https://www.sciencedirect.com/science/article/pii/S2405896324015866},
doi = {https://doi.org/10.1016/j.ifacol.2024.09.171},
issn = {2405-8963},
year = {2024},
date = {2024-01-01},
urldate = {2024-01-01},
journal = {IFAC-PapersOnLine},
volume = {58},
number = {19},
pages = {217-222},
abstract = {The increasing need of product individualization and rapid demand fluctuation leads industrial manufacturers to leveraging new control solutions for production planning and control. Among these, distributed control approaches for the multi job shop scheduling problem could be a promising solution to make smart manufacturing systems more responsive to the market. In this context, this paper aims at investigating the effectiveness and the benefits of distributed control approaches for solving the multi job shop assignment problem on a real-world smart manufacturing process involving the production of high-vacuum solar panels. The validation is carried out by leveraging Cyber-Physical Systems modelling and a digital model-based procedure which, interacting with the physical plant, provides the proper job assignment in order to optimize the overall makespan and work-in-progress in a fully-distributed fashion.},
note = {18th IFAC Symposium on Information Control Problems in Manufacturing INCOM 2024},
keywords = {Control, Digital-Model-Based Simulation, Distributed control, Multi-Job Shop Scheduling, Smart Manufacturing Planning},
pubstate = {published},
tppubtype = {article}
}
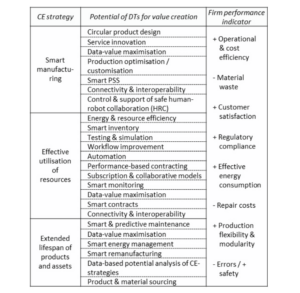
Rodrigo Torres Adelsberger; Oliver Antons; Julia Arlinghaus
Digital Twins and their Implications for Business Models: Overview and Potentials Artikel
In: IFAC-PapersOnLine, Bd. 58, Nr. 19, S. 409-414, 2024, ISSN: 2405-8963, (18th IFAC Symposium on Information Control Problems in Manufacturing INCOM 2024).
Abstract | Links | BibTeX | Schlagwörter: Business Model, Circular Business Model, Circular Economy, Digital Twin, Digital Twin-enabled Business Model, Industry 4.0, Servitisation, Smart Product-Service System
@article{ADELSBERGER2024409,
title = {Digital Twins and their Implications for Business Models: Overview and Potentials},
author = {Rodrigo Torres Adelsberger and Oliver Antons and Julia Arlinghaus},
url = {https://www.sciencedirect.com/science/article/pii/S2405896324016756},
doi = {https://doi.org/10.1016/j.ifacol.2024.09.246},
issn = {2405-8963},
year = {2024},
date = {2024-01-01},
urldate = {2024-01-01},
journal = {IFAC-PapersOnLine},
volume = {58},
number = {19},
pages = {409-414},
abstract = {Digital Twins are key elements to develop the complex digital systems required for an effective Circular Economy transition. This study explores the intersection of Digital Twins and Business Models within Industry 4.0 and offers a comprehensive theoretical background of the advancements in Digital Twins and their potentials to innovate Business Models. Through a literature review, we identify and discuss eleven Digital Twin-enabled Business Models. The study suggests the necessity of further research efforts to validate theoretical perspectives and identifies future research venues, such as the exploration of Digital Twin-enabled Circular Business Models.},
note = {18th IFAC Symposium on Information Control Problems in Manufacturing INCOM 2024},
keywords = {Business Model, Circular Business Model, Circular Economy, Digital Twin, Digital Twin-enabled Business Model, Industry 4.0, Servitisation, Smart Product-Service System},
pubstate = {published},
tppubtype = {article}
}
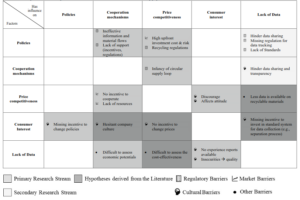
Anna-Kristin Behnert; Oliver Antons; Julia Arlinghaus
Exploring the Challenges of Circular Economy Adoption: A Supply Chain Perspective Artikel
In: IFAC-PapersOnLine, Bd. 58, Nr. 19, S. 211-216, 2024, ISSN: 2405-8963, (18th IFAC Symposium on Information Control Problems in Manufacturing INCOM 2024).
Abstract | Links | BibTeX | Schlagwörter: Cause-And-Effect Relationships, Circular Economy, Circular Supply Chain, Supply Chain Management, Sustainability
@article{BEHNERT2024211,
title = {Exploring the Challenges of Circular Economy Adoption: A Supply Chain Perspective},
author = {Anna-Kristin Behnert and Oliver Antons and Julia Arlinghaus},
url = {https://www.sciencedirect.com/science/article/pii/S2405896324015829},
doi = {https://doi.org/10.1016/j.ifacol.2024.09.168},
issn = {2405-8963},
year = {2024},
date = {2024-01-01},
urldate = {2024-01-01},
journal = {IFAC-PapersOnLine},
volume = {58},
number = {19},
pages = {211-216},
abstract = {The prevailing linear economic structure and our complex global supply chains are reaching their tangible limits, as evidenced by the impact of current crises such as energy scarcity. A shift from a linear to a Circular Economy is necessary to meet the urgent environmental and economic challenges. This paper aims to address a significant gap in the literature by utilizing a structured literature review and the Supply Chain Operation Reference (SCOR) model. Rather than focusing on specific contexts or industries, this paper aims to examine the intercorrelation and interdependencies between the barriers from a supply chain perspective. Therefore, the diverse conceptual background of the Circular Economy and the complexity of its implementation due to the interconnectedness of barriers that span regulatory, cultural, market, and technological aspects are analyzed. The interdependence of barriers in the different supply chain stages is highlighted in a qualitative and explorative research approach. The key findings reveal that a successful supply chain management strategy that integrates Circular Economy principles along the whole supply chain is crucial for successfully transitioning from a linear to a Circular Economy. We identify necessary lines of future research to facilitate the successful transition and overcome the current barriers along the supply chain.},
note = {18th IFAC Symposium on Information Control Problems in Manufacturing INCOM 2024},
keywords = {Cause-And-Effect Relationships, Circular Economy, Circular Supply Chain, Supply Chain Management, Sustainability},
pubstate = {published},
tppubtype = {article}
}
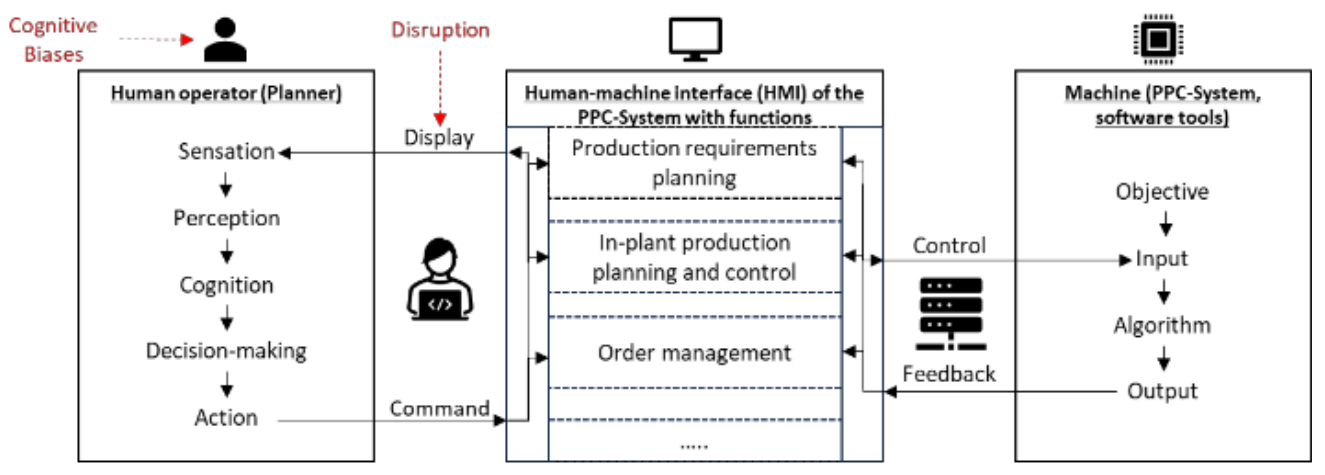
Patrick Rannertshauser; Oliver Antons; Julia C. Arlinghaus
International Conference on Industry 4.0 and Smart Manufacturing, Bd. 232, 2024.
Abstract | Links | BibTeX | Schlagwörter: Cognitiv Bias, Disruption, Production planning and control
@conference{nokey,
title = {Interaction between planners and PPC systems \textendash Derivation of simulation scenarios considering cognitive biases and disruptions},
author = {Patrick Rannertshauser and Oliver Antons and Julia C. Arlinghaus},
editor = {Procedia Computer Science},
url = {https://www.sciencedirect.com/science/article/pii/S1877050924001352?utm_campaign=STMJ_219742_AUTH_SERV_PA\&utm_medium=email\&utm_acid=269271194\&SIS_ID=\&dgcid=STMJ_219742_AUTH_SERV_PA\&CMX_ID=\&utm_in=DM458849\&utm_source=AC_},
doi = {10.1016/j.procs.2024.01.135},
year = {2024},
date = {2024-00-00},
booktitle = {International Conference on Industry 4.0 and Smart Manufacturing},
volume = {232},
pages = {1367-1376},
abstract = {Despite an increase in the availability of digital solutions, planners’ remain at the center of the production planning and control (PPC) decision-making process and have to make numerous decisions involving different information. Due to the inherent complexities, planners’ are supported by PPC systems to fulfil their tasks. Research has shown that humans are influenced by cognitive bias when making decisions, especially in uncertain and complex environments and when disruptions such as machine failures occur. Researchers have therefore proposed debiasing methods to reduce human errors in decision-making. However, there is currently no environment within this research to test these proposed methods. In this study, we aimed to take the first step toward closing this research gap. We combined existing PPC research with a systematic literature review on disruptions in production as well as perspectives from practice through interviews with 12 experts. We investigated the interactions between planners’ and PPC systems with consideration of different cognitive biases and disturbances to derive relevant simulation uses cases.},
keywords = {Cognitiv Bias, Disruption, Production planning and control},
pubstate = {published},
tppubtype = {conference}
}
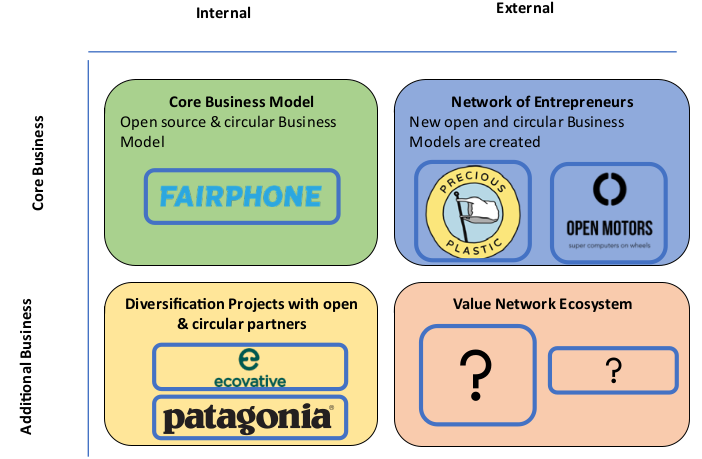
Anna-Kristin Behnert; Oliver Antons; Julia C. Arlinghaus
International Conference on Industry 4.0 and Smart Manufacturing, Bd. 232, 2024.
Abstract | Links | BibTeX | Schlagwörter: Circular Business Models, Circular Economy, Open Business Models, Open Source
@conference{nokey,
title = {Characterizing Circular and Open Business Models in a profit-driven environment through Business Model Patterns},
author = {Anna-Kristin Behnert and Oliver Antons and Julia C. Arlinghaus},
url = {https://www.sciencedirect.com/science/article/pii/S1877050924000437},
doi = {10.1016/j.procs.2024.01.043},
year = {2024},
date = {2024-00-00},
booktitle = {International Conference on Industry 4.0 and Smart Manufacturing},
volume = {232},
pages = {436-445},
abstract = {In recent years, multiple crises have shown the fragility of global supply chains. In particular, climate change shed light on the never-ending waste of resources caused by the linear economic model. In response, circular economy emerges as a promising alternative. For a systemic transition, companies must rethink and innovate their business models, product life cycles, and underlying mindsets. Cross-sectoral collaboration in networks is necessary to redesign, reuse, recycle, and remanufacture products and close the loop of material flows. First, projects and research on the concepts of open source hardware, open innovation, and open design show that openness can be a game-changer for the circular economy and circular business models. Although there has been growing interest, there is still a lack of comprehensive research examining possible synergies of openness and circularity and identifying patterns for integrating these concepts into business models. This paper explores possible ways, prerequisites, and obstacles of open source concepts enabling circular value creation in the industry. We combine explorative data from a literature review and more than 10 interviews with open source and circular economy experts. Furthermore, a total of 38 case studies of circular or open or open \& circular business models were identified and selected for analysis. The empirical results were triangulated with existing business model patterns from the literature. The study presents a framework to describe open and circular business models and four prototypical patterns. In an exploratory approach, we identify necessary lines of future research in order to facilitate open source movements toward a circular economy. The paper provides insights for academics and practitioners from production, supply chain, and management as it combines interdisciplinary perspectives to unlock the potential of open source concepts and collaboration for enabling a circular economy.},
keywords = {Circular Business Models, Circular Economy, Open Business Models, Open Source},
pubstate = {published},
tppubtype = {conference}
}
2023
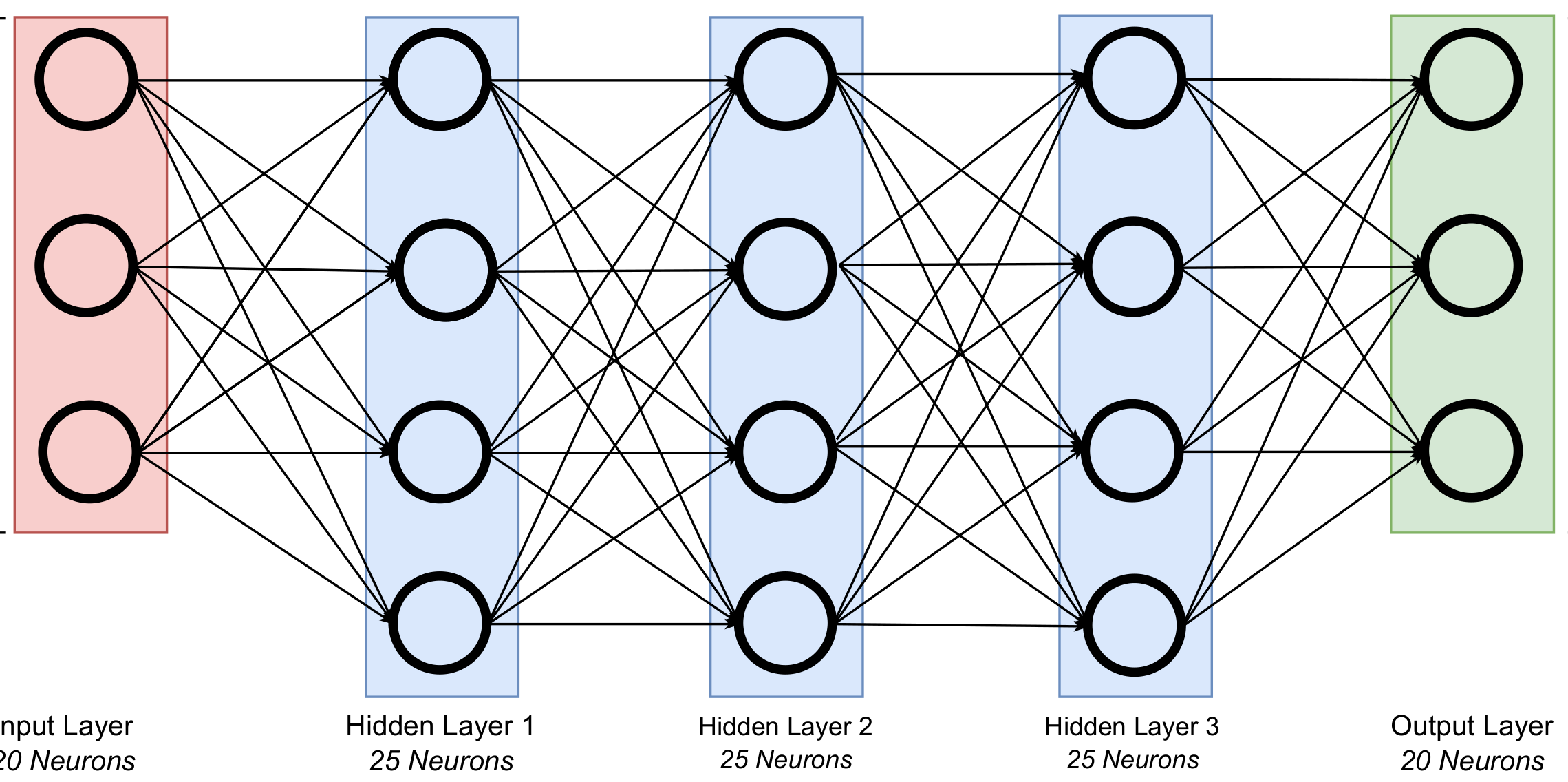
Oliver Antons; Julia C. Arlinghaus
Maximum Likelihood and Neural Network Estimators for Distributed Production Control Proceedings Article
In: IFAC-PapersOnLine , S. 10327-10332, 2023, ISSN: 2405-8963, (22nd IFAC World Congress).
Abstract | Links | BibTeX | Schlagwörter: Cyber-physical system, Distributed control, Machine learning, Production planning and control, Smart manufacutring systems
@inproceedings{nokey,
title = {Maximum Likelihood and Neural Network Estimators for Distributed Production Control},
author = {Oliver Antons and Julia C. Arlinghaus},
url = {https://www.sciencedirect.com/science/article/pii/S2405896323014210},
doi = {10.1016/j.ifacol.2023.10.1038},
issn = {2405-8963},
year = {2023},
date = {2023-07-14},
urldate = {2023-07-14},
booktitle = {IFAC-PapersOnLine },
volume = {56},
number = {2},
pages = {10327-10332},
abstract = {Cyber-physical systems have become increasingly common in recent years, providing a multitude of information regarding production processes. At the same time, increasing volatilities, uncertainties, complexity and ambiguity (VUCA) are challenging existing production control approaches for manufacturing networks. Data-driven control approaches are an avenue to address VUCA, but require further study in research and practice. We utilize a multi-agent based discrete-event simulation to compare the aptitudes of a maximum likelihood and neural network based estimator for distributed production control, and provide insights into application of machine learning to address ever increasing information and VUCA.},
note = {22nd IFAC World Congress},
keywords = {Cyber-physical system, Distributed control, Machine learning, Production planning and control, Smart manufacutring systems},
pubstate = {published},
tppubtype = {inproceedings}
}
Oliver Antons; Julia C. Arlinghaus
In: International Journal of Production Research, Bd. 0, Nr. 0, S. 1-19, 2023.
Abstract | Links | BibTeX | Schlagwörter: Autonomous production control, Centralized control, Decision-making authority, Distributed control, Manufacturing network topology, Production planning and control
@article{doi:10.1080/00207543.2023.2217285,
title = {Designing distributed decision-making authorities for smart factories \textendash understanding the role of manufacturing network architecture},
author = {Oliver Antons and Julia C. Arlinghaus},
url = {https://doi.org/10.1080/00207543.2023.2217285},
doi = {10.1080/00207543.2023.2217285},
year = {2023},
date = {2023-01-01},
urldate = {2023-01-01},
journal = {International Journal of Production Research},
volume = {0},
number = {0},
pages = {1-19},
publisher = {Taylor \& Francis},
abstract = {The availability of cyber-physical systems (CPS) in modern manufacturing networks provides a multitude of interesting opportunities from a manufacturing control perspective. Providing sensors, data gathering, local computation and communication capabilities modern CPS fulfil the technical requirements to act completely autonomously in a manufacturing network. While the distribution of decision-making authority to autonomous entities is feasible given such requirements, practice often sees the monopolisation of decision-making authority for centralised control. However, distributed production control approaches might be better suited given current manufacturing challenges, ranging from unreliable supply chains over highly volatile markets, to the demand for increasingly efficient and highly customisable production. In this article, we extend an existing scheduling complexity framework which enables practitioners and researchers alike to assess the aptitude of given manufacturing networks for both centralised and distributed control. In particular, we study the influence of a manufacturing network's topology ranging from assembly line to job shops on the aforementioned aptitude, with total production costs as objective.
We utilise a multi-agent-based discrete-event simulation comparing an MILP-based centralised control approach and an autonomy based distributed control approach with weighted costs as decision function to evaluate this framework. Our results provide novel insights regarding the influence of manufacturing network topologies on the scheduling complexity of manufacturing networks.},
keywords = {Autonomous production control, Centralized control, Decision-making authority, Distributed control, Manufacturing network topology, Production planning and control},
pubstate = {published},
tppubtype = {article}
}
We utilise a multi-agent-based discrete-event simulation comparing an MILP-based centralised control approach and an autonomy based distributed control approach with weighted costs as decision function to evaluate this framework. Our results provide novel insights regarding the influence of manufacturing network topologies on the scheduling complexity of manufacturing networks.
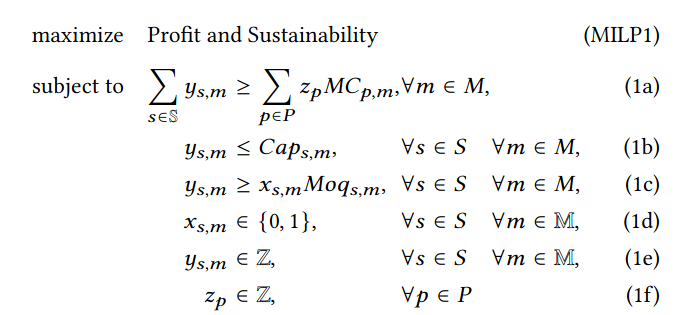
Tobias Benecke; Oliver Antons; Sanaz Mostaghim; Julia C. Arlinghaus
A Generalized Circular Supply Chain Problem for Multi-Objective Evolutionary Algorithms Proceedings Article
In: Proceedings of the Companion Conference on Genetic and Evolutionary Computation, S. 355–358, Association for Computing Machinery, Lisbon, Portugal, 2023, ISBN: 9798400701207.
Abstract | Links | BibTeX | Schlagwörter: Benchmarking, Evolutionary algorithms, Multi-objective optimization, Supply chain optimization
@inproceedings{10.1145/3583133.3590742,
title = {A Generalized Circular Supply Chain Problem for Multi-Objective Evolutionary Algorithms},
author = {Tobias Benecke and Oliver Antons and Sanaz Mostaghim and Julia C. Arlinghaus},
url = {https://doi.org/10.1145/3583133.3590742},
doi = {10.1145/3583133.3590742},
isbn = {9798400701207},
year = {2023},
date = {2023-01-01},
urldate = {2023-01-01},
booktitle = {Proceedings of the Companion Conference on Genetic and Evolutionary Computation},
pages = {355\textendash358},
publisher = {Association for Computing Machinery},
address = {Lisbon, Portugal},
series = {GECCO '23 Companion},
abstract = {The idea of a circular economy proves promising with the evergrowing need for more sustainable production methods and resource utilization. However, this introduces new challenges compared to the traditional, mostly linear production processes and often leads to a tradeoff between sustainability and costs. In these environments, multi-objective evolutionary algorithms (MOEAs) are a great tool to tackle the increased complexity of supply chains in a circular economy. While MOEAs have been used to optimize circular supply chain models in the past, it was usually done for specific industries and using standard operators. In this paper, we propose a generalized test problem to provide a tool for evaluating MOEAs with respect to a circular supply chain (CSC) problem. In this problem, we try to optimize the product plan as well as the material sourcing at the same time, considering the objectives of maximizing the profit and sustainable resource use.},
keywords = {Benchmarking, Evolutionary algorithms, Multi-objective optimization, Supply chain optimization},
pubstate = {published},
tppubtype = {inproceedings}
}
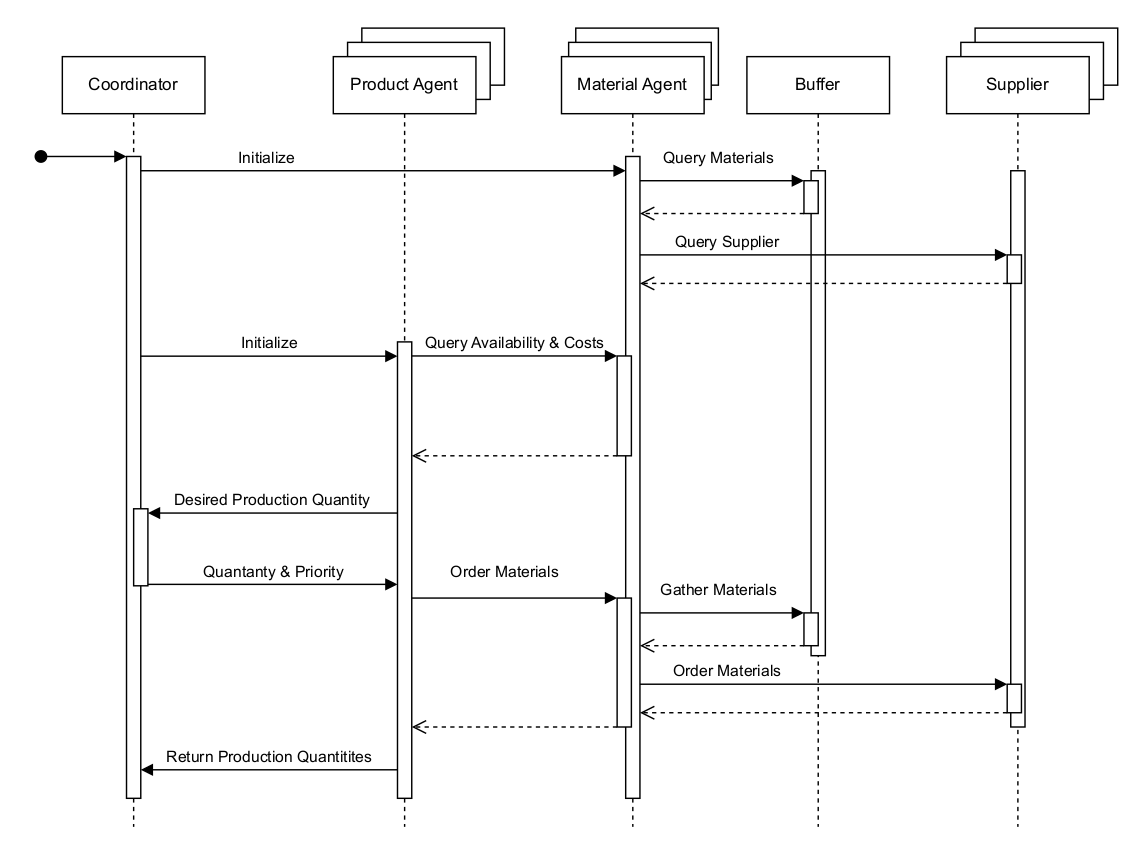
Oliver Antons; Tobias Benecke; Sanaz Mostaghim; Julia C. Arlinghaus
Sustainability in Chemical Production–Multi-Objective Distributed Control Buchabschnitt
In: New Trends in Intelligent Software Methodologies, Tools and Techniques, S. 211–219, IOS Press, 2023.
Abstract | Links | BibTeX | Schlagwörter: Chemical industry, Circular Economy, Distributed control, Optimization, Sustainability
@incollection{antons2023sustainability,
title = {Sustainability in Chemical Production\textendashMulti-Objective Distributed Control},
author = {Oliver Antons and Tobias Benecke and Sanaz Mostaghim and Julia C. Arlinghaus},
doi = {10.3233/FAIA230236},
year = {2023},
date = {2023-01-01},
urldate = {2023-01-01},
booktitle = {New Trends in Intelligent Software Methodologies, Tools and Techniques},
pages = {211\textendash219},
publisher = {IOS Press},
abstract = {Chemical industry provides a multitude of intermediaries and final products essential to society, ranging from fertilizers and plastics to sophisticated pharmaceuticals. The underlying production processes are typically linear, utilizing finite resources in an unsustainable manner and creating unnecessary waste over a products lifetime. While a shift towards sustainability and circular economy is desired, the current market and political framework lead to conflicting objectives ranging from sustainability to profit maximization. In this article, we build upon a first minimal multi-objective MILP model and extend thereupon, reducing the overall level of required abstraction compared to the first model. Thereafter, we present a multi-agent based distributed optimization approach for a sequence of the extended MILP formulation.},
keywords = {Chemical industry, Circular Economy, Distributed control, Optimization, Sustainability},
pubstate = {published},
tppubtype = {incollection}
}
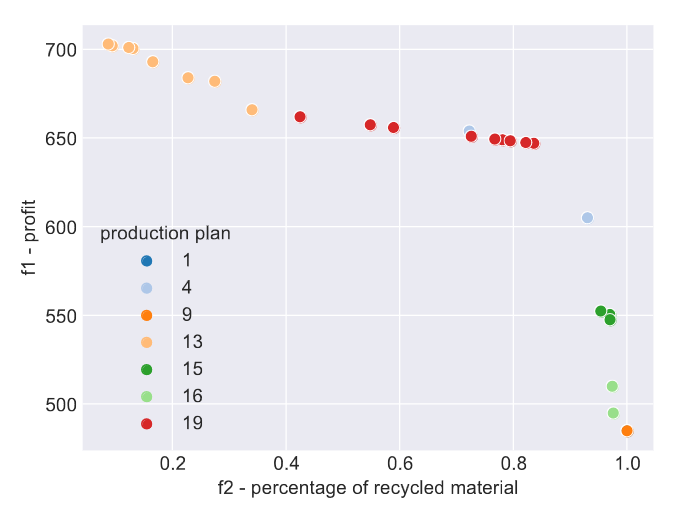
Tobias Benecke; Oliver Antons; Sanaz Mostaghim; Julia C. Arlinghaus
A Coevolution Approach for the Multi-objective Circular Supply Chain Problem Proceedings Article
In: 2023 IEEE Conference on Artificial Intelligence (CAI), S. 222-223, 2023.
Abstract | Links | BibTeX | Schlagwörter: Complexity Theory, Costs, Production Planning, Profitability, Supply chain optimization
@inproceedings{10195126,
title = {A Coevolution Approach for the Multi-objective Circular Supply Chain Problem},
author = {Tobias Benecke and Oliver Antons and Sanaz Mostaghim and Julia C. Arlinghaus},
doi = {10.1109/CAI54212.2023.00103},
year = {2023},
date = {2023-01-01},
urldate = {2023-01-01},
booktitle = {2023 IEEE Conference on Artificial Intelligence (CAI)},
pages = {222-223},
abstract = {As a more sustainable resource use is becoming of greater concern, moving towards a more circular economy seems promising. However, compared to the traditional, mostly linear production processes, this introduces new challenges, as reintroducing recycled materials into production also increases supply chain complexity and therefore cost. The circular supply chain (CSC) problem is modeling these challenges to find good tradeoff solutions between profitability and sustainable resource use. The optimization concerns the production planning and material sourcing of a production plant. This is a complex task due to their inherent dependencies. In this paper, we use a cooperative coevolutionary approach to optimize the CSC problem, by decomposing it to resolve the variable dependencies. Besides presenting the algorithm, a proof of concept evaluation is done to show its feasibility.},
keywords = {Complexity Theory, Costs, Production Planning, Profitability, Supply chain optimization},
pubstate = {published},
tppubtype = {inproceedings}
}
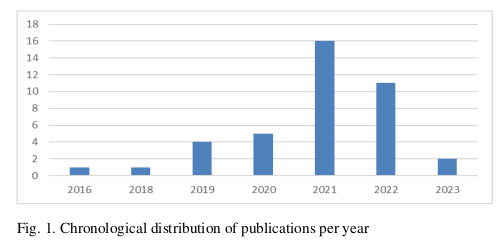
Konstantin Büttner; Oliver Antons; Julia C. Arlinghaus
Exploring Implementation Barriers of Machine Learning in Production Planning and Control Konferenz
Bd. 120, 2023, (56th CIRP CMS 2023 - Manufacturing in an age of disruption).
Abstract | Links | BibTeX | Schlagwörter:
@conference{nokey,
title = {Exploring Implementation Barriers of Machine Learning in Production Planning and Control},
author = {Konstantin B\"{u}ttner and Oliver Antons and Julia C. Arlinghaus},
url = {https://www.sciencedirect.com/science/article/pii/S2212827123009435},
doi = {10.1016/j.procir.2023.09.211},
year = {2023},
date = {2023-00-00},
urldate = {2023-00-00},
volume = {120},
pages = {1546-1551},
abstract = {This paper studies the challenges encountered during the implementation of machine learning (ML) applications in production planning and control (PPC) functions of manufacturing companies. The application of ML in PPC promises numerous benefits, and several studies have revealed the positive impact of such an implementation on key performance indicators of production systems, such as lead time, due date reliability, and inventory levels. However, despite the theoretical potential and the increased research interest in the field over the last five years, practical applications remain rare and discourse on the existence of this gap between research and practice remains relatively sparse. In this paper, we identify general ML implementation barriers the manufacturing domain based on a structured literature review and evaluate the relevance of these barriers within the PPC domain by interviewing ML and PPC experts. This research is beneficial for practitioners and researchers who aim to implement ML applications in PPC by enabling them to evaluate the possible barriers and challenges during implementation projects within their organizations.},
note = {56th CIRP CMS 2023 - Manufacturing in an age of disruption},
keywords = {},
pubstate = {published},
tppubtype = {conference}
}
2022
Oliver Antons
Distributing decision-making authority: autonomous entities in manufacturing networks Promotionsarbeit
Rheinisch-Westfälische Technische Hochschule Aachen, 2022, (Veröffentlicht auf dem Publikationsserver der RWTH Aachen University; Dissertation, Rheinisch-Westfälische Technische Hochschule Aachen, 2022).
Abstract | Links | BibTeX | Schlagwörter: Autonomy, Decision-making authority, Discrete-event simulation, Multi-agent system, Production planning and control
@phdthesis{Antons:856980,
title = {Distributing decision-making authority: autonomous entities in manufacturing networks},
author = {Oliver Antons},
url = {https://oliver.antons.eu/research/decision-making-authority/ , Autonomy \& Decision-making Authority
https://publications.rwth-aachen.de/record/856980 , RWTH Publication Server},
doi = {10.18154/RWTH-2022-11291},
year = {2022},
date = {2022-12-16},
urldate = {2022-12-16},
pages = {1 Online-Ressource : Illustrationen},
publisher = {RWTH Aachen University},
address = {Aachen},
school = {Rheinisch-Westf\"{a}lische Technische Hochschule Aachen},
abstract = {Industrial production was faced with increasing challenges in the last years. Market volatility, rising energy costs and disrupted supply networks resulted in an ever-increasing information variability, which decreases productivity and complicates production planning and control (PPC). Moreover, the ever-increasing demand for, and differentiation through customization also makes planning more difficult. At the same time manufacturing networks have seen a further computerization on a machine level by the introduction of cyber-physical systems (CPS). Capable to process information, gather sensor data locally and communicate within a network, these machine provide an enormous increase in potentials for manufacturing networks. Thus, the technical requirements for distributed production control approaches are fulfilled, based on CPS acting as autonomous entities within a manufacturing network. Such distributed production control approaches feature a number of interesting characteristics, which are often quite contrary to established concepts of traditional, centralized production control. In the literature, many research streams are concerned with the advantages and disadvantages of both centralized and distributed control. While many articles provide deep insights into the workings of specialized control approaches for specific manufacturing environments, an overarching framework allowing a holistic comparison between the two fundamental production control approaches is lacking. In this thesis, the term decision-making authority is introduced to describe the level of autonomy an entity is allowed to exhibit with regard to the potential decisions it could make. Furthermore, both centralized and distributed production control approaches for manufacturing networks based on potentially autonomous entities are explored. In the former case, every entity but one central controller is not allowed to exhibit any decision-making authority, acting purely as command recipients. In the latter case, however, the aforementioned entities have a predefined degree of decision-making authority, enabling them to make certain decisions of the production scheduling on their own. Based on environment variables derived by extensive literature review, a sophisticated simulation framework is developed in form of a multi-agent based discrete-event simulation (MAS-DES). This simulation framework represents all objects of a manufacturing network, such as machines and products as agents. These agents can either follow a global plan, derived from a mixed-integer linear program modeling a centralized production control approach, or act autonomously within the scope of their respective decision-making authority in a distributed production control approach. The main part of this thesis consists of five research articles, presented in Chapters II - VI. Chapter II reviews the historic ply between centralized and decentralized control, followed by a structured literature review regarding autonomy in production planning and control, manufacturing and related research streams. Extending this, Chapter III studies the difference in information scopes of different classes of potentially autonomous entities in a manufacturing network. Chapter IV provides guidance to both researchers and practitioners alike by introducing a scheduling complexity framework, based on environment variables derived from the literature. A multi-agent based discrete-event simulation is utilized to validate the framework quantitatively. Following, Chapter V extends the simulations to study the influence of a manufacturing network’s topology on its aptitude for both centralized and distributed production control approaches. Chapter VI explores synergistic potentials between machine learning and distributed production control for manufacturing networks. Lastly, the thesis ends with a conclusion summarizing results, noting limitations and presenting avenues for future research.},
note = {Ver\"{o}ffentlicht auf dem Publikationsserver der RWTH Aachen
University; Dissertation, Rheinisch-Westf\"{a}lische Technische
Hochschule Aachen, 2022},
keywords = {Autonomy, Decision-making authority, Discrete-event simulation, Multi-agent system, Production planning and control},
pubstate = {published},
tppubtype = {phdthesis}
}
Konstantin Büttner; Oliver Antons; Julia C. Arlinghaus
Applied Machine Learning for Production Planning and Control: Overview and Potentials Proceedings Article
In: 10th IFAC Conference on Manufacturing Modelling, Management and Control, S. 6, Elsevier, 2022.
Abstract | Links | BibTeX | Schlagwörter: Control, Machine learning, Production control, Production Planning
@inproceedings{Buettner2022,
title = {Applied Machine Learning for Production Planning and Control: Overview and Potentials},
author = {Konstantin B\"{u}ttner and Oliver Antons and Julia C. Arlinghaus},
url = {https://www.sciencedirect.com/science/article/pii/S2405896322021152},
doi = {https://doi.org/10.1016/j.ifacol.2022.10.106},
year = {2022},
date = {2022-10-01},
urldate = {2022-10-01},
booktitle = {10th IFAC Conference on Manufacturing Modelling, Management and Control},
volume = {55},
number = {10},
pages = {6},
publisher = {Elsevier},
abstract = {Manufacturing companies are under constant pressure to increase efficiency and to achieve logistical objectives. Improving production planning and control (PPC) has significant impact on these efforts. At the same time, increasing complexity and dynamics of PPC environments make PPC more difficult. One way to cope with this situation is the application of machine learning (ML) methods. In this article, we therefore address the current state of PPC-ML research and show, based on the Aachen PPC model, in which PPC tasks and subtasks ML is already applied and to what degree the task is covered by ML. The analysis is limited to core and cross-sectional tasks of the Aachen PPC model, procurement and network tasks are not included. Furthermore, a broad analysis of the targeted data mining, business and logistic objectives is conducted. In addition, we also identify motivations which prompted researchers to apply ML in PPC. },
keywords = {Control, Machine learning, Production control, Production Planning},
pubstate = {published},
tppubtype = {inproceedings}
}
Julia C. Arlinghaus; Oliver Antons
Management for Digitalization and Industry 4.0 Buchkapitel
In: Berlin Springer, Heidelberg (Hrsg.): Bd. Handbook Industry 4.0, S. 927-948, 2022.
Abstract | Links | BibTeX | Schlagwörter:
@inbook{Arlinghaus2022,
title = {Management for Digitalization and Industry 4.0},
author = {Julia C. Arlinghaus and Oliver Antons},
editor = {Springer, Berlin, Heidelberg},
url = {https://doi.org/10.1007/978-3-662-64448-5_49},
doi = {10.1007/978-3-662-64448-5_49},
year = {2022},
date = {2022-06-23},
urldate = {2022-06-23},
volume = {Handbook Industry 4.0},
pages = {927-948},
abstract = {Global trends are changing how and what European companies manufacture. Progressive globalization and constant and immediate availability of relevant information through the Internet are not only changing the structure of global value networks but also compelling companies to operate under increasingly intense time, quality, innovation and cost pressure. Hence, the associated complexity of products, value networks and the planning and control processes is consequently growing. European companies are thus facing numerous seemingly conflicting challenges that their respective management has to reconcile.},
keywords = {},
pubstate = {published},
tppubtype = {inbook}
}
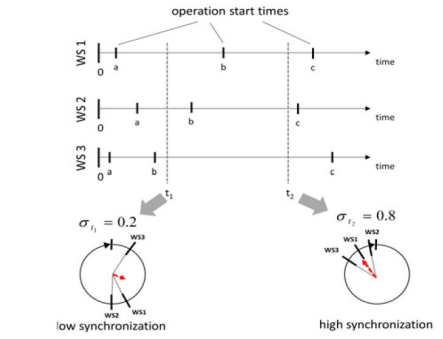
Florian Knapp; Oliver Antons; Julia C. Arlinghaus
Opportunities for Synchronization in Manufacturing as Key Performance Indicator Proceedings Article
In: S. 1467-1472, 2022, ISSN: 2212-8271, (Leading manufacturing systems transformation – Proceedings of the 55th CIRP Conference on Manufacturing Systems 2022).
Abstract | Links | BibTeX | Schlagwörter: Control, Key Performance Indicators, Manufacturing, Manufacturing Networks, Production Planning, Synchronization
@inproceedings{KNAPP20221467,
title = {Opportunities for Synchronization in Manufacturing as Key Performance Indicator},
author = {Florian Knapp and Oliver Antons and Julia C. Arlinghaus},
url = {https://www.sciencedirect.com/science/article/pii/S2212827122004607},
doi = {https://doi.org/10.1016/j.procir.2022.05.176},
issn = {2212-8271},
year = {2022},
date = {2022-01-01},
urldate = {2022-01-01},
journal = {Procedia CIRP},
volume = {107},
pages = {1467-1472},
abstract = {In the complex and dynamic conditions of global markets it is increasingly challenging for manufacturing companies to find or identify new reliable and meaningful key performance indicators. Traditional performance indicators like lead time, inventory level, on-time delivery rate and capacity utilization are still in focus, but interdisciplinary research suggests the inclusion of more innovative performance indicators, such as synchronization. The synchronization level of a manufacturing system can be viewed twofold: logistic and physical synchronization. Physical synchronization is defined as "the rhythm and repetitive behaviour of production processes", while logistical synchronization describes "the coupling of work systems that are linked by material flows." Both types of synchronization correlate with the logistics performance of companies. Previous research on this topic has already provided first insights, highlighting potentials and fields of application. In this paper, we provide a structured literature review underlining relevant applications and explore their respective potentials. Thus, we show how synchronization effects can be exploited, and which systemic properties, such as network topology, characteristics and process time variation influence the occurrence of synchronization.},
note = {Leading manufacturing systems transformation \textendash Proceedings of the 55th CIRP Conference on Manufacturing Systems 2022},
keywords = {Control, Key Performance Indicators, Manufacturing, Manufacturing Networks, Production Planning, Synchronization},
pubstate = {published},
tppubtype = {inproceedings}
}
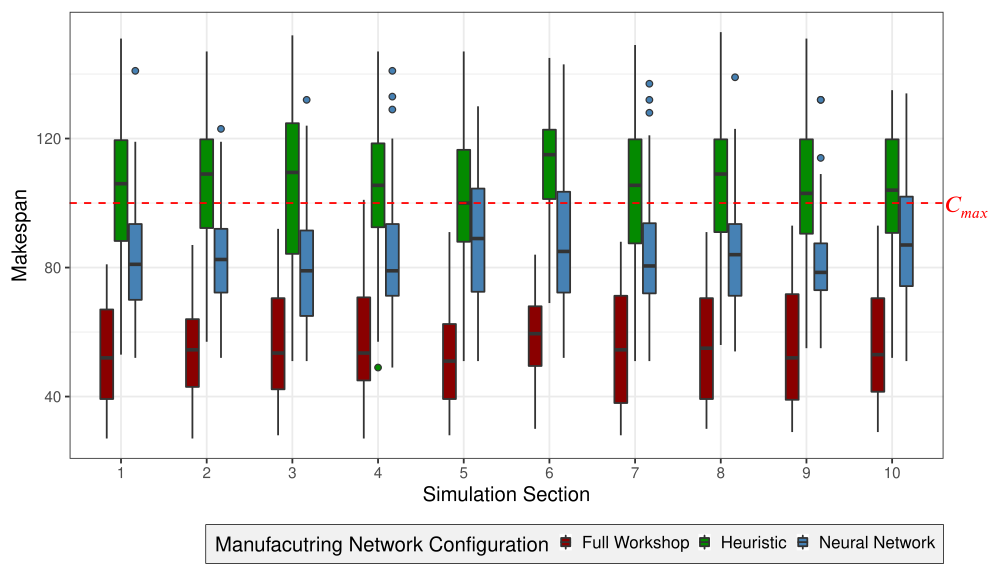
Oliver Antons; Julia C. Arlinghaus
Data-driven and autonomous manufacturing control in cyber-physical production systems Artikel
In: Computers in Industry, Bd. 141, S. 103711, 2022, ISSN: 0166-3615.
Abstract | Links | BibTeX | Schlagwörter: Autonomous production control, Machine learning, Neural network, Production planning and control
@article{ANTONS2022103711,
title = {Data-driven and autonomous manufacturing control in cyber-physical production systems},
author = {Oliver Antons and Julia C. Arlinghaus},
url = {https://www.sciencedirect.com/science/article/pii/S0166361522001087},
doi = {https://doi.org/10.1016/j.compind.2022.103711},
issn = {0166-3615},
year = {2022},
date = {2022-01-01},
urldate = {2022-01-01},
journal = {Computers in Industry},
volume = {141},
pages = {103711},
abstract = {Modern manufacturing networks consist of cyber-physical systems (CPS) which offer an array of interesting capabilities, ranging from local computation over data generation to communication capabilities. As traditional control approaches fail to fully leverage these capabilities, the last decade has seen a renewed interest in distributed control approaches based on autonomous entities. In this article, we study the synergistic potentials of autonomous control and machine learning in a job-shop setting, addressing challenges of modern manufacturing such as market fluctuation and process time variance, thus leveraging the potentials of CPS in order to flexibly configure manufacturing networks and achieve cost-minimal production. We utilize a multi-agent based discrete-event simulation to compare this novel approach to a traditional heuristic, underlining the potentials and advantages of data-driven control approaches.},
keywords = {Autonomous production control, Machine learning, Neural network, Production planning and control},
pubstate = {published},
tppubtype = {article}
}
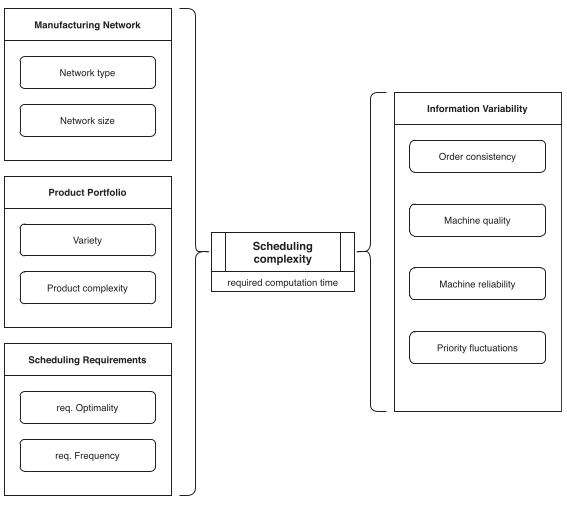
Oliver Antons; Julia C. Arlinghaus
In: IEEE Journal of Emerging and Selected Topics in Industrial Electronics, Bd. 3, Nr. 1, S. 31-38, 2022.
Abstract | Links | BibTeX | Schlagwörter: Complexity Theory, Decentralized Control, Job-shop scheduling, Manufacturing, Optimization, Production
@article{antons2021ieee,
title = {A Manufacturing Scheduling Complexity Framework and Agent-Based Comparison of Centralized and Distributed Control Approaches},
author = {Oliver Antons and Julia C. Arlinghaus},
url = {https://doi.org/10.1109/JESTIE.2021.3100272},
doi = {10.1109/JESTIE.2021.3100272},
year = {2022},
date = {2022-01-01},
urldate = {2022-01-01},
journal = {IEEE Journal of Emerging and Selected Topics in Industrial Electronics},
volume = {3},
number = {1},
pages = {31-38},
abstract = {Centralized approaches are often employed to control manufacturing networks in practice. The introduction of industrial cyber-physical systems driven by advances in microcontroller, sensor, and networking technologies is providing distributed control systems with the technical requirements needed to mitigate the drawbacks of centralized control, such as long optimization times that result in long planning horizons and inflexibility. While such distributed control approaches respond to the growing challenges faced by industry in terms of flexibility, resilience, and lot sizes, the inherent myopia of autonomous agents may discourage practical application. In this article, we develop a scheduling complexity framework derived from the literature, which allows researchers and prationers alike to evaluate the suitability of both centralized and distributed control approaches for manufacturing planning and control. This framework utilizes quantifiable environment variables, which influence we study by means of a multiagent discrete event simulation.},
keywords = {Complexity Theory, Decentralized Control, Job-shop scheduling, Manufacturing, Optimization, Production},
pubstate = {published},
tppubtype = {article}
}
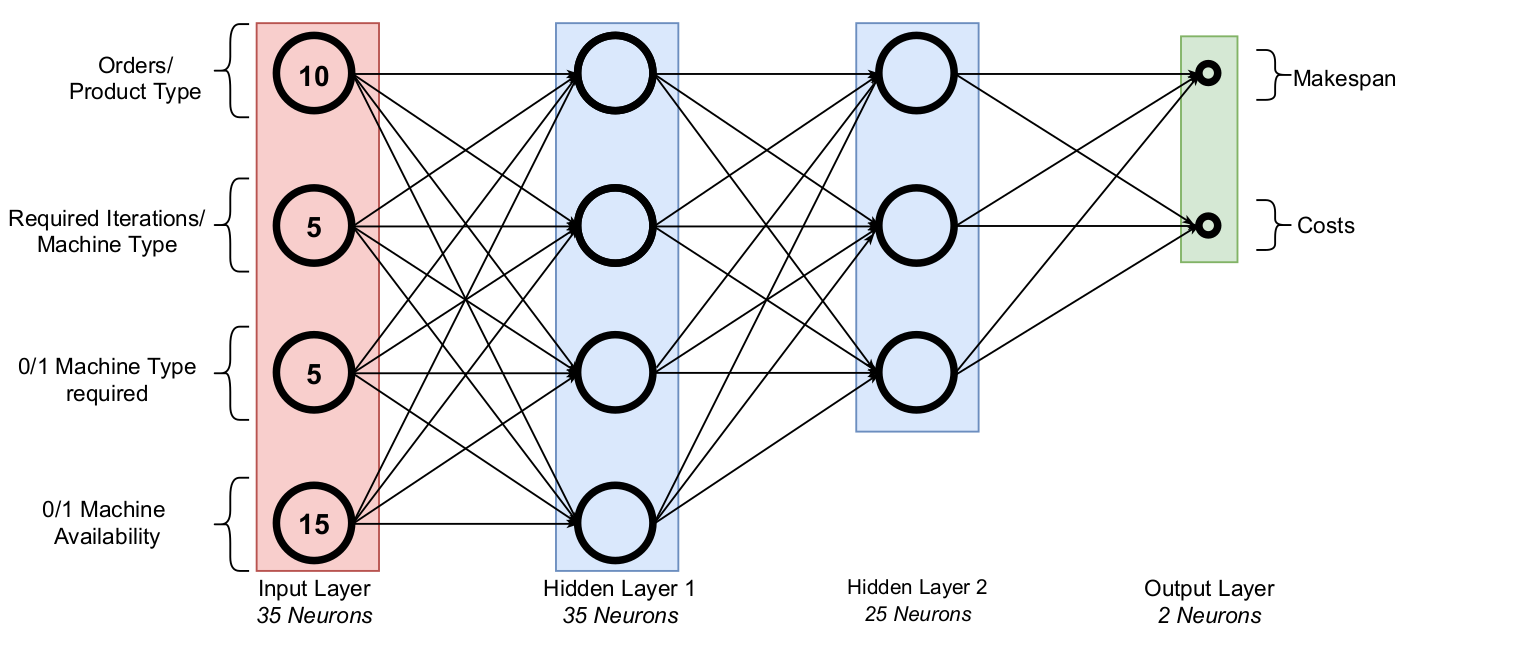
Oliver Antons; Julia C. Arlinghaus
Machine Learning and Autonomous Control—A Synergy for Manufacturing Proceedings Article
In: Borangiu, Theodor; Trentesaux, Damien; Leitão, Paulo; Cardin, Olivier; Joblot, Laurent (Hrsg.): Service Oriented, Holonic and Multi-agent Manufacturing Systems for Industry of the Future, S. 417–428, Springer International Publishing, Cham, 2022, ISBN: 978-3-030-99108-1.
Abstract | Links | BibTeX | Schlagwörter: Distributed control, Machine learning, Manufacturing, Neural network
@inproceedings{10.1007/978-3-030-99108-1_30,
title = {Machine Learning and Autonomous Control\textemdashA Synergy for Manufacturing},
author = {Oliver Antons and Julia C. Arlinghaus},
editor = {Theodor Borangiu and Damien Trentesaux and Paulo Leit\~{a}o and Olivier Cardin and Laurent Joblot},
url = {https://link.springer.com/chapter/10.1007/978-3-030-99108-1_30},
doi = {10.1007/978-3-030-99108-1_30 },
isbn = {978-3-030-99108-1},
year = {2022},
date = {2022-01-01},
urldate = {2022-01-01},
booktitle = {Service Oriented, Holonic and Multi-agent Manufacturing Systems for Industry of the Future},
pages = {417\textendash428},
publisher = {Springer International Publishing},
address = {Cham},
abstract = {This papers studies the synergistic potentials of machine learning and distributed control approaches in a job-shop setting. We utilize a multi-agent based discrete-event simulation to model distributed control in conjunction with a neural network to predict the optimal workshop configuration given fluctuating production demands. Within this simulation model, we study the potential cost and time savings, showing various potentials in the synergistic utilization of distributed control and machine learning for production planning and control in a job-shop manufacturing network.},
keywords = {Distributed control, Machine learning, Manufacturing, Neural network},
pubstate = {published},
tppubtype = {inproceedings}
}
2021
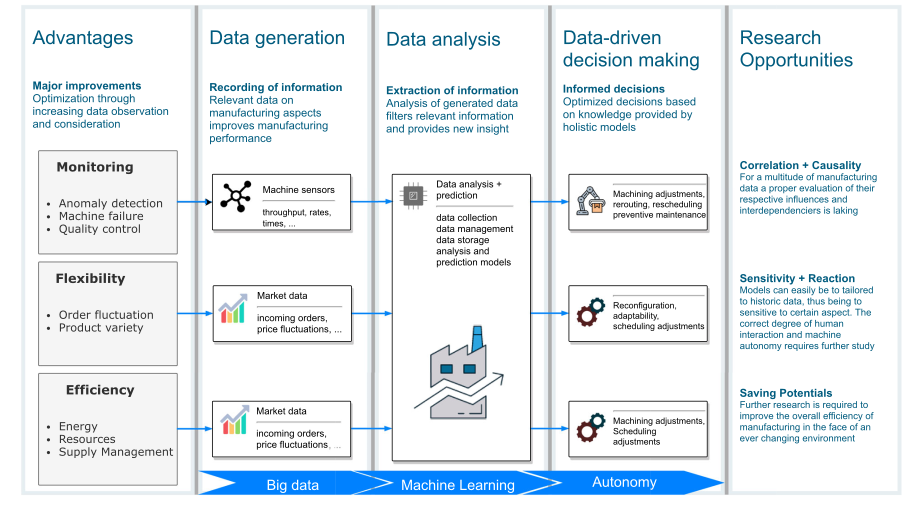
Oliver Antons; Julia C. Arlinghaus
In: International Journal of Production Research, Bd. 60, Ausg. 13, Nr. 0, S. 1-19, 2021.
Abstract | Links | BibTeX | Schlagwörter: Autonomous production control, Autonomy, Decentralized Control, Industry 4.0, Production planning and control
@article{Antons2021Distributing,
title = {Distributing decision-making authority in manufacturing \textendash review and roadmap for the factory of the future},
author = {Oliver Antons and Julia C. Arlinghaus},
url = {https://doi.org/10.1080/00207543.2022.2057255},
doi = {10.1080/00207543.2022.2057255},
year = {2021},
date = {2021-07-27},
urldate = {2021-07-27},
journal = {International Journal of Production Research},
volume = {60},
number = {0},
issue = {13},
pages = {1-19},
publisher = {Taylor \& Francis},
abstract = {The question of the benefits of autonomous control is more important than ever: production managers, governments and society hope that the vision of smart and digital production systems with high flexibility and low costs may save the value adding and therefore welfare in the high wage, industrialised countries. At the same time, the discussion on the social implications of autonomous objects and decentralised control approaches is growing. Looking back on the history of production research and practice, we find that there has been a constant ply among scholars and production managers between the advantages of the two concepts of centralised and decentralised control approaches. In this article, we study the concept of autonomy in production planning and control, enabled by cyber-physical systems and the distribution of decision-making authority. Based on a profound structured literature review, we analyse the perception of autonomy, the technological requirements and the increasing complexities of modern smart manufacturing. Moreover, we find that recently several research streams suggest the advantages and benefits of autonomous control concepts compared to traditional centralised approaches based on qualitative analysis and identify a distinct lack of quantitative results.},
keywords = {Autonomous production control, Autonomy, Decentralized Control, Industry 4.0, Production planning and control},
pubstate = {published},
tppubtype = {article}
}
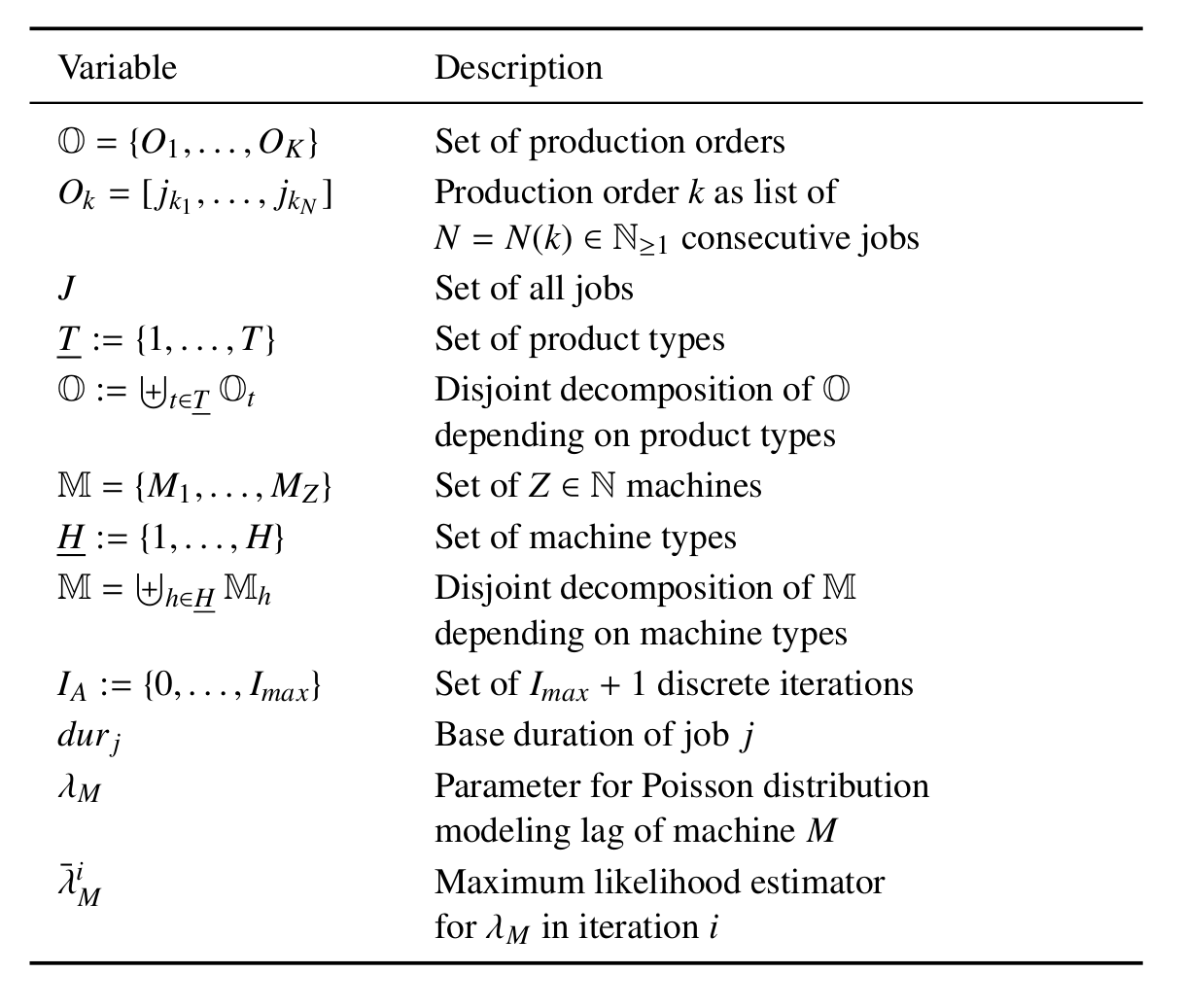
Oliver Antons; Julia C. Arlinghaus
Adaptive self-learning distributed and centralized control approaches for smart factories Proceedings Article
In: S. 1577-1582, 2021, ISSN: 2212-8271, (54th CIRP CMS 2021 - Towards Digitalized Manufacturing 4.0).
Abstract | Links | BibTeX | Schlagwörter: Autonomy, Cyber-physical system, Data analytics, Decision-making, Discrete-event simulation, Distributed control, Industry 4.0, Multi-agent system, Self-learning, Smart factory
@inproceedings{ANTONS20211577,
title = {Adaptive self-learning distributed and centralized control approaches for smart factories},
author = {Oliver Antons and Julia C. Arlinghaus},
url = {https://www.sciencedirect.com/science/article/pii/S2212827121011641},
doi = {https://doi.org/10.1016/j.procir.2021.11.266},
issn = {2212-8271},
year = {2021},
date = {2021-01-01},
urldate = {2021-01-01},
journal = {Procedia CIRP},
volume = {104},
pages = {1577-1582},
abstract = {The increasing application of cyber-physical systems creates a manufacturing environment in which the technical requirements for distributed control approaches, self-learning systems and analytics of previously untapped data are given. While distributed control approaches are capable to evaluate this information locally and react immediately, centralized approaches react inertly to analyzed machine performance data. In this paper, we study the performance and ability to address the ever increasing challenges in industry of both types of control approaches within an established multi-agent based discrete event simulation.},
note = {54th CIRP CMS 2021 - Towards Digitalized Manufacturing 4.0},
keywords = {Autonomy, Cyber-physical system, Data analytics, Decision-making, Discrete-event simulation, Distributed control, Industry 4.0, Multi-agent system, Self-learning, Smart factory},
pubstate = {published},
tppubtype = {inproceedings}
}
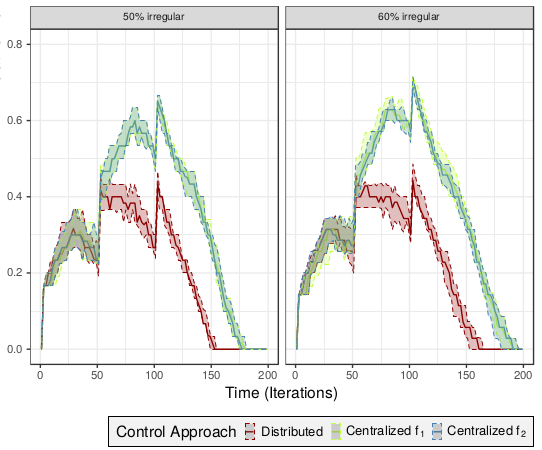
Oliver Antons; Julia C. Arlinghaus
Distributed control for Industry 4.0 -A comparative simulation study Proceedings Article
In: S. 516-521, 2021, ISSN: 2405-8963, (17th IFAC Symposium on Information Control Problems in Manufacturing INCOM 2021).
Abstract | Links | BibTeX | Schlagwörter: Control, Discrete-event simulation, Distributed control, Make-to-order, Manufacturing planning, Multi-agent system
@inproceedings{ANTONS2021516,
title = {Distributed control for Industry 4.0 -A comparative simulation study},
author = {Oliver Antons and Julia C. Arlinghaus},
url = {https://www.sciencedirect.com/science/article/pii/S2405896321007801},
doi = {https://doi.org/10.1016/j.ifacol.2021.08.059},
issn = {2405-8963},
year = {2021},
date = {2021-01-01},
urldate = {2021-01-01},
journal = {IFAC-PapersOnLine},
volume = {54},
number = {1},
pages = {516-521},
abstract = {The recent decades have seen various market changes that industrial manufactures need to address. Increasing individualization of products and rapid demand fluctuations require new control approaches. In this article, we study the performance of centralized and distributed control approaches with regard to work in progress in a make-to-order environment. We utilize a multi-agent system in a discrete-event simulation to model several control approaches and provide various insights into potential benefits of distributed control concepts given rapidly fluctuating manufacturing demand.},
note = {17th IFAC Symposium on Information Control Problems in Manufacturing INCOM 2021},
keywords = {Control, Discrete-event simulation, Distributed control, Make-to-order, Manufacturing planning, Multi-agent system},
pubstate = {published},
tppubtype = {inproceedings}
}
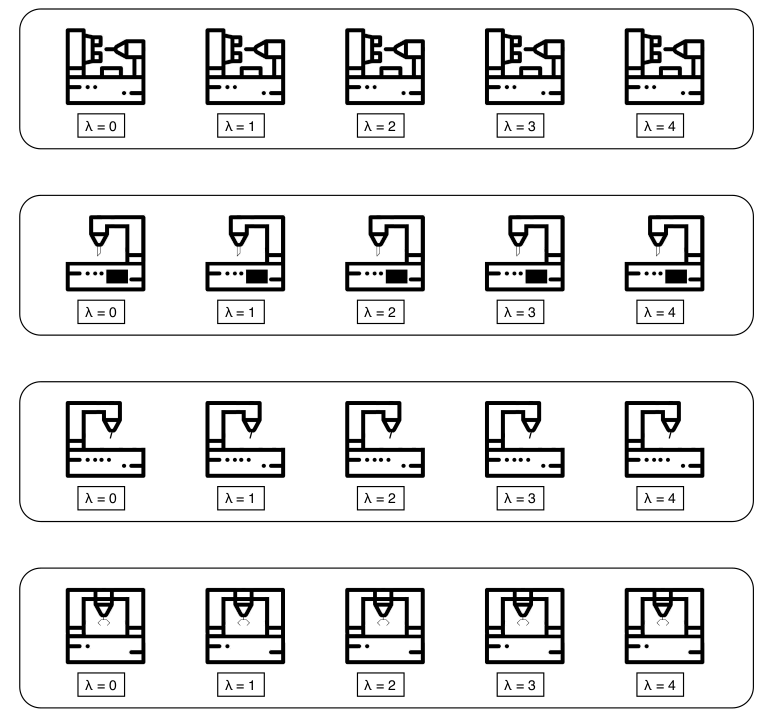
Oliver Antons; Julia C. Arlinghaus
Learning Distributed Control for Job Shops-A Comparative Simulation Study Proceedings Article
In: Service Oriented, Holonic and Multi-Agent Manufacturing Systems for Industry of the Future: Proceedings of SOHOMA 2020, S. 193–202, Springer International Publishing 2021.
Abstract | Links | BibTeX | Schlagwörter: Discrete-event simulation, Job-shop scheduling, Multi-agent system, Production planning and control, Self-learning
@inproceedings{antons2021learning,
title = {Learning Distributed Control for Job Shops-A Comparative Simulation Study},
author = {Oliver Antons and Julia C. Arlinghaus},
url = {https://link.springer.com/book/10.1007/978-3-030-69373-2},
doi = {10.1007/978-3-030-69373-2},
year = {2021},
date = {2021-01-01},
urldate = {2021-01-01},
booktitle = {Service Oriented, Holonic and Multi-Agent Manufacturing Systems for Industry of the Future: Proceedings of SOHOMA 2020},
pages = {193--202},
organization = {Springer International Publishing},
abstract = {This paper studies the potentials of learning and benefits of local data processing in a distributed control setting. We deploy a multi-agent system in the context of a discrete-event simulation to model distributed control for a job shop manufacturing system with variable processing times and multi-stage production processes. Within this simulation, we compare queue length estimation as dispatching rule against a variation with learning capability, which processes additional historic data on a machine agent level, showing the potentials of learning and coordination for distributed control in PPC.},
keywords = {Discrete-event simulation, Job-shop scheduling, Multi-agent system, Production planning and control, Self-learning},
pubstate = {published},
tppubtype = {inproceedings}
}
2020
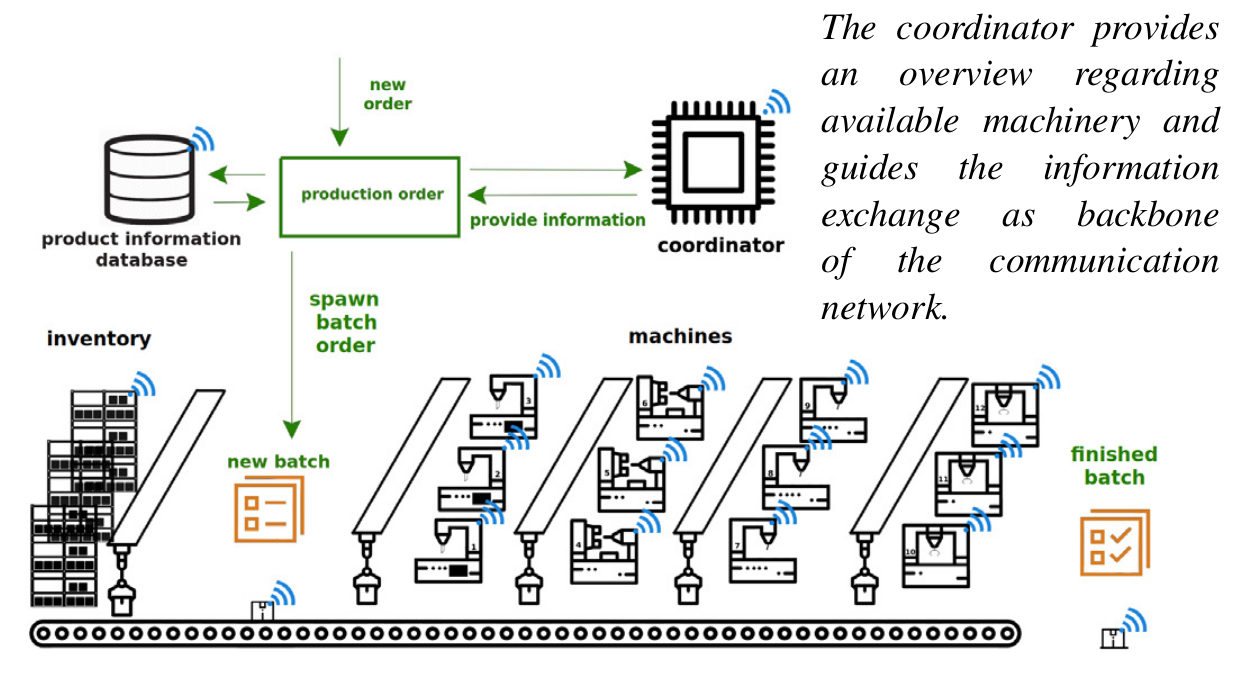
Oliver Antons; Julia C. Arlinghaus
Designing decision-making authorities for smart factories Proceedings Article
In: S. 316–322, Elsevier, 2020.
Abstract | Links | BibTeX | Schlagwörter: Autonomy, Cyber-physical system, Decision-making, Discrete-event simulation, Distributed control, Industry 4.0, Intelligent Product, Multi-agent system, Smart factory
@inproceedings{antons2020designing,
title = {Designing decision-making authorities for smart factories},
author = {Oliver Antons and Julia C. Arlinghaus},
url = {https://www.sciencedirect.com/science/article/pii/S2212827120306120},
doi = {10.1016/j.procir.2020.04.047},
year = {2020},
date = {2020-01-01},
urldate = {2020-01-01},
journal = {Procedia CIRP},
volume = {93},
pages = {316--322},
publisher = {Elsevier},
abstract = {Smart factories are an appealing vision, addressing ever increasing challenges in industry. Driven by advances in microcontroller, sensor and networking technologies, all entities, such as machines, products, load carriers, within a smart factory could become intelligent and able to assess their own situation and to attain their own goals successfully. However, local decision may exacerbate achieving global logistics performance due to the entities intrinsic selfishness. In this paper, we explore the trade-off between local decision-making and global performance management and derive first guidelines for the situation-specific design of a distributed control authority for smart factories.},
keywords = {Autonomy, Cyber-physical system, Decision-making, Discrete-event simulation, Distributed control, Industry 4.0, Intelligent Product, Multi-agent system, Smart factory},
pubstate = {published},
tppubtype = {inproceedings}
}
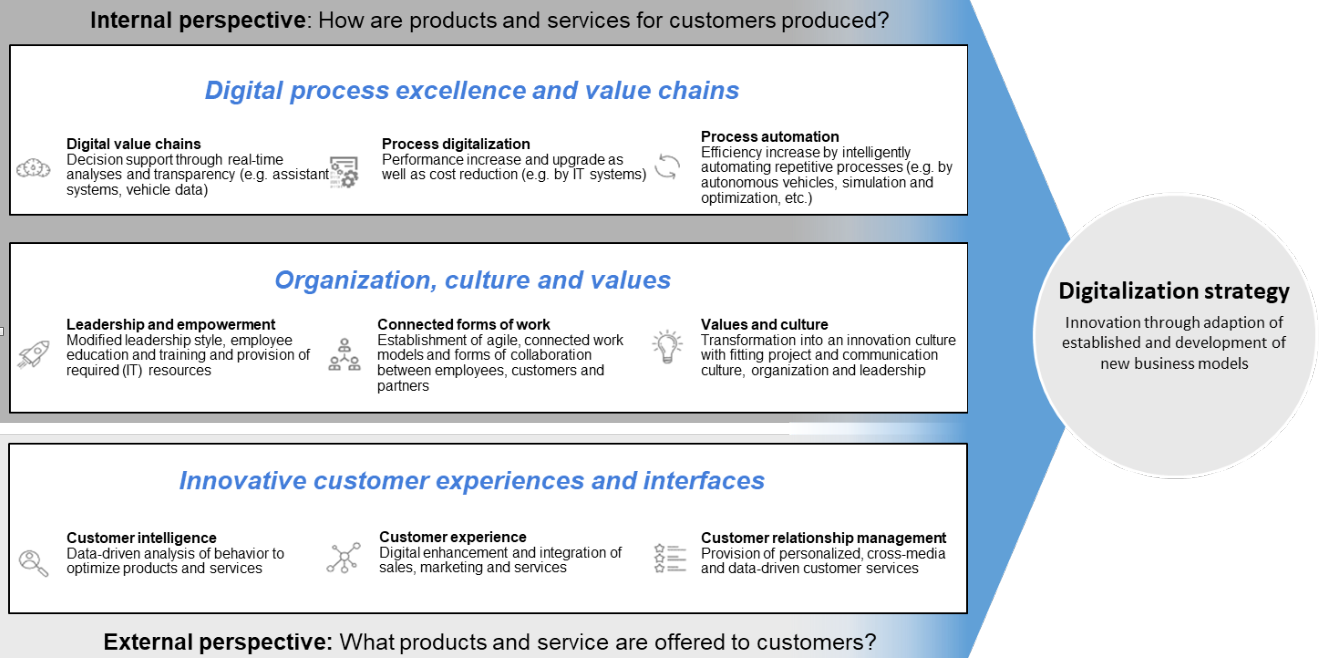
Julia C. Arlinghaus; Oliver Antons
Management für Digitalisierung und Industrie 4.0 Buchkapitel
In: Handbuch Industrie 4.0: Recht, Technik, Gesellschaft, S. 1121–1145, Springer, 2020.
Abstract | Links | BibTeX | Schlagwörter:
@inbook{arlinghaus2020management,
title = {Management f\"{u}r Digitalisierung und Industrie 4.0},
author = {Julia C. Arlinghaus and Oliver Antons},
url = {https://link.springer.com/chapter/10.1007/978-3-662-58474-3_58},
doi = {10.1007/978-3-662-58474-3_58},
year = {2020},
date = {2020-01-01},
urldate = {2020-01-01},
booktitle = {Handbuch Industrie 4.0: Recht, Technik, Gesellschaft},
pages = {1121--1145},
publisher = {Springer},
abstract = {Globale Trends ver\"{a}ndern wie und was europ\"{a}ische Unternehmen produzieren. Die fortschreitende Globalisierung und die permanente und direkte Verf\"{u}gbarkeit relevanter Informationen durch das Internet beeinflussen aber nicht nur die Struktur der globalen Wertsch\"{o}pfungsnetzwerke, sondern zwingen Unternehmen auch, unter immer gr\"{o}\sserem Zeit-, Qualit\"{a}ts-, Innovations- und Kostendruck zu arbeiten. Infolgedessen steigt die Komplexit\"{a}t der Produkte, der Wertsch\"{o}pfungsnetze und der dahinterliegenden Planungs- und Steuerungsprozesse. Vor diesem Hintergrund ist die unter dem Begriff "Industrie 4.0" zusammengefasste Vision zu einem Hoffnungstr\"{a}ger f\"{u}r die europ\"{a}ische Wirtschaft geworden. Dabei reicht diese Vision l\"{a}ngst \"{u}ber den Ursprung des Begriffs \textendash einer digitalisierten, intelligenten Fabrik \textendash hinaus und umfasst nahezu alle Gesch\"{a}ftsbereiche. Der damit f\"{u}r Unternehmen essenzielle Wandlungsprozess wird dabei meist als "Digitale Transformation" bezeichnet. Dabei handelt es sich jedoch nicht um eine einmalige Anpassung mit klarem Anfangs- und Endpunkt, sondern um einen fortw\"{a}hrenden Anpassungsprozess, den jedes Unternehmen f\"{u}r sich spezifisch gestalten und f\"{u}hren muss.},
keywords = {},
pubstate = {published},
tppubtype = {inbook}
}
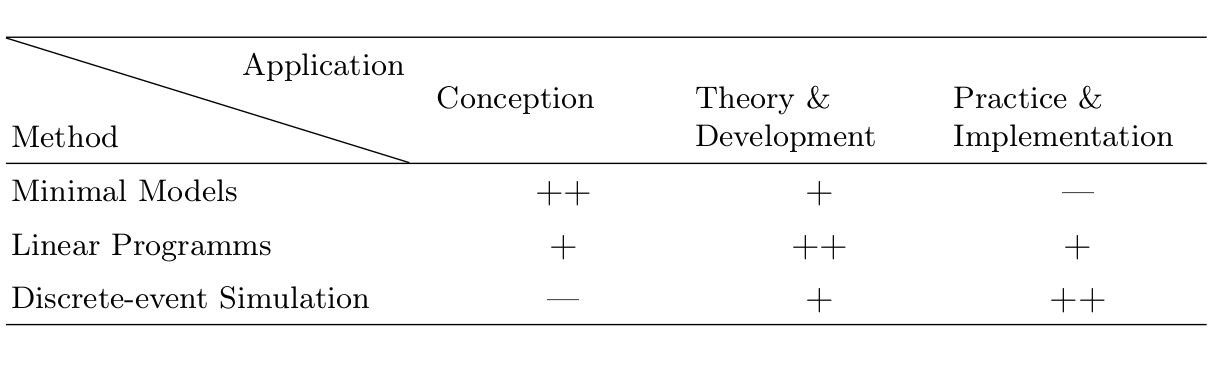
Oliver Antons; Julia C. Arlinghaus
Modelling Autonomous Production Control: A Guide to Select the Most Suitable Modelling Approach Proceedings Article
In: International Conference on Dynamics in Logistics, S. 245–253, Springer 2020.
Abstract | Links | BibTeX | Schlagwörter: Autonomous production control, Discrete-event simulation, Linear programming, Minimal models, Production planning and control
@inproceedings{antons2020modelling,
title = {Modelling Autonomous Production Control: A Guide to Select the Most Suitable Modelling Approach},
author = {Oliver Antons and Julia C. Arlinghaus},
url = {https://link.springer.com/chapter/10.1007/978-3-030-44783-0_24},
doi = {10.1007/978-3-030-44783-0_24},
year = {2020},
date = {2020-01-01},
urldate = {2020-01-01},
booktitle = {International Conference on Dynamics in Logistics},
pages = {245--253},
organization = {Springer},
abstract = {This paper studies and compares Minimal Models, Linear Programming and Discrete-event Simulation as approaches to model Production Planning and Control with regard to their ability to include the concept of autonomous control. After a brief explanation of autonomous control in production planning, the three aforementioned concepts are introduced in detail. We derive their benefits and drawbacks for different scenarios, and subsequently give advice when to deploy each method, applicable for researchers and practioners alike.},
keywords = {Autonomous production control, Discrete-event simulation, Linear programming, Minimal models, Production planning and control},
pubstate = {published},
tppubtype = {inproceedings}
}
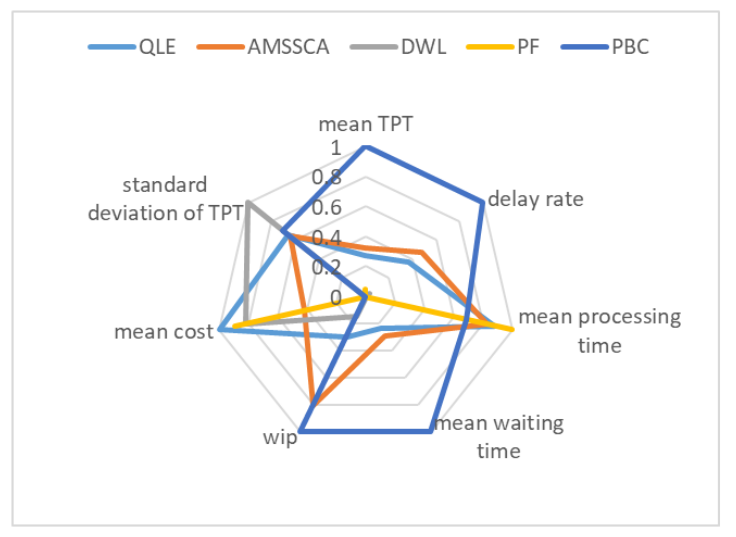
Ziqi Zhao; Oliver Antons; Julia C. Arlinghaus
Autonomous Production Control Methods-Job Shop Simulations Proceedings Article
In: International Conference on Dynamics in Logistics, S. 227–235, Springer 2020.
Abstract | Links | BibTeX | Schlagwörter: Autonomous production control, Industry 4.0, Production planning and control
@inproceedings{zhao2020autonomous,
title = {Autonomous Production Control Methods-Job Shop Simulations},
author = {Ziqi Zhao and Oliver Antons and Julia C. Arlinghaus},
url = {https://link.springer.com/chapter/10.1007/978-3-030-44783-0_22},
doi = {10.1007/978-3-030-44783-0_22},
year = {2020},
date = {2020-01-01},
urldate = {2020-01-01},
booktitle = {International Conference on Dynamics in Logistics},
pages = {227--235},
organization = {Springer},
abstract = {With the development of Industry 4.0 and the Internet of Things, autonomous production control is regarded as a feasible and promising approach for meeting the increasing challenges of complexity and flexibility. To implement autonomous production control methods in the practice, a deeper understanding of their characteristics is necessary. This research provides a comparative perspective on existing methods. We study selected autonomous production control methods under various scenarios, and derive insights for the design of such systems in industrial practice.},
keywords = {Autonomous production control, Industry 4.0, Production planning and control},
pubstate = {published},
tppubtype = {inproceedings}
}
2019
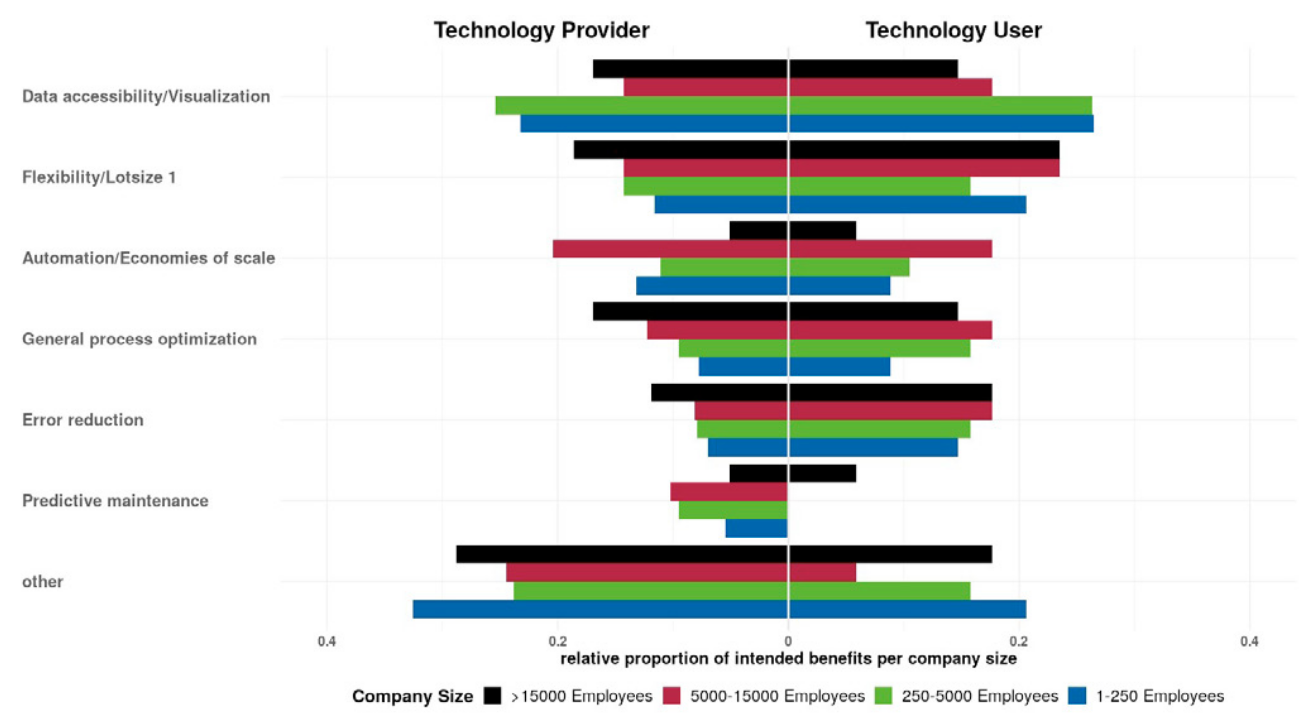
Manuel Zimmermann; Eugenia Rosca; Oliver Antons; Julia C. Bendul
Supply chain risks in times of Industry 4.0: Insights from German cases Proceedings Article
In: S. 1755–1760, Elsevier, 2019.
Abstract | Links | BibTeX | Schlagwörter: Digitalization, Industry 4.0, Risk factors, Risk management, Technologies
@inproceedings{zimmermann2019supply,
title = {Supply chain risks in times of Industry 4.0: Insights from German cases},
author = {Manuel Zimmermann and Eugenia Rosca and Oliver Antons and Julia C. Bendul},
url = {https://www.sciencedirect.com/science/article/pii/S2405896319314363},
doi = {10.1016/j.ifacol.2019.11.455},
year = {2019},
date = {2019-01-01},
urldate = {2019-01-01},
journal = {IFAC-PapersOnLine},
volume = {52},
number = {13},
pages = {1755--1760},
publisher = {Elsevier},
abstract = {This study investigates the impact of Industry 4.0 technologies on supply chain risks and examines which factors moderate this relationship. Drawing on a database of 300 Industry 4.0 projects all around Germany, the study explores empirically how Industry 4.0 technologies are employed to address various sources of various risks within production and supply chains. In this context, not only relevant technologies, existing risk factors and intended benefits are explored, but also factors that affect the relationship between these elements. Therefore, this paper shed light on the question which technologies are suitable and applicable for which user groups in which situations.},
keywords = {Digitalization, Industry 4.0, Risk factors, Risk management, Technologies},
pubstate = {published},
tppubtype = {inproceedings}
}
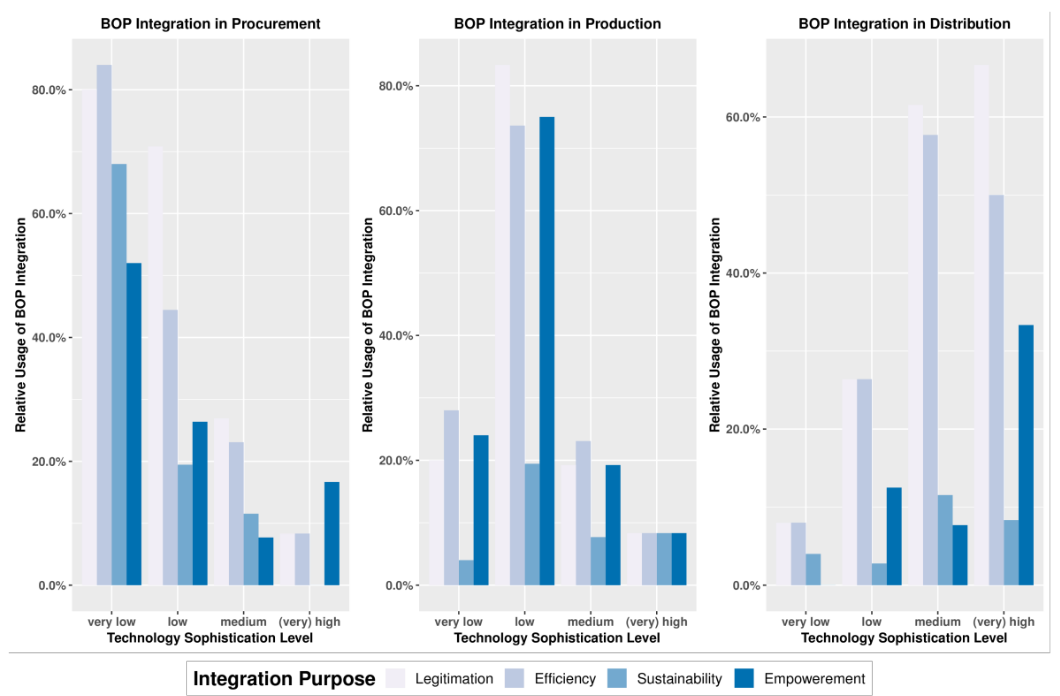
Stephanie Knizkov; Oliver Antons; Julia C. Bendul
Designing Inclusive Supply Chains for Sustainability: Empirical Insights from the Bottom of the Global Economic Pyramid Proceedings Article
In: EurOMA19, 2019.
Abstract | BibTeX | Schlagwörter: Base of the Pyramid, Co-creation, Sustainability, Sustainable Development
@inproceedings{Knizkov-2019,
title = {Designing Inclusive Supply Chains for Sustainability: Empirical Insights from the Bottom of the Global Economic Pyramid},
author = {Stephanie Knizkov and Oliver Antons and Julia C. Bendul},
year = {2019},
date = {2019-01-01},
urldate = {2019-01-01},
booktitle = {EurOMA19},
abstract = {Integration of the population at the Bottom of the Pyramid (BOP) within company operations was identified as pivotal for realizing both value for companies operating in these challenging markets, as well as for harnessing the full potential of the BOP proposition in fostering value for individuals living in them. However, research on the topic remains predominantly simplistic, with nuanced and empiric based argumentation relatively scarce. In this paper we address this gap and provide a clear overview of how companies integrate BOP individuals in the different stages of their supply-chain, and how this integration facilitates different levels of sustainability depth.},
keywords = {Base of the Pyramid, Co-creation, Sustainability, Sustainable Development},
pubstate = {published},
tppubtype = {inproceedings}
}
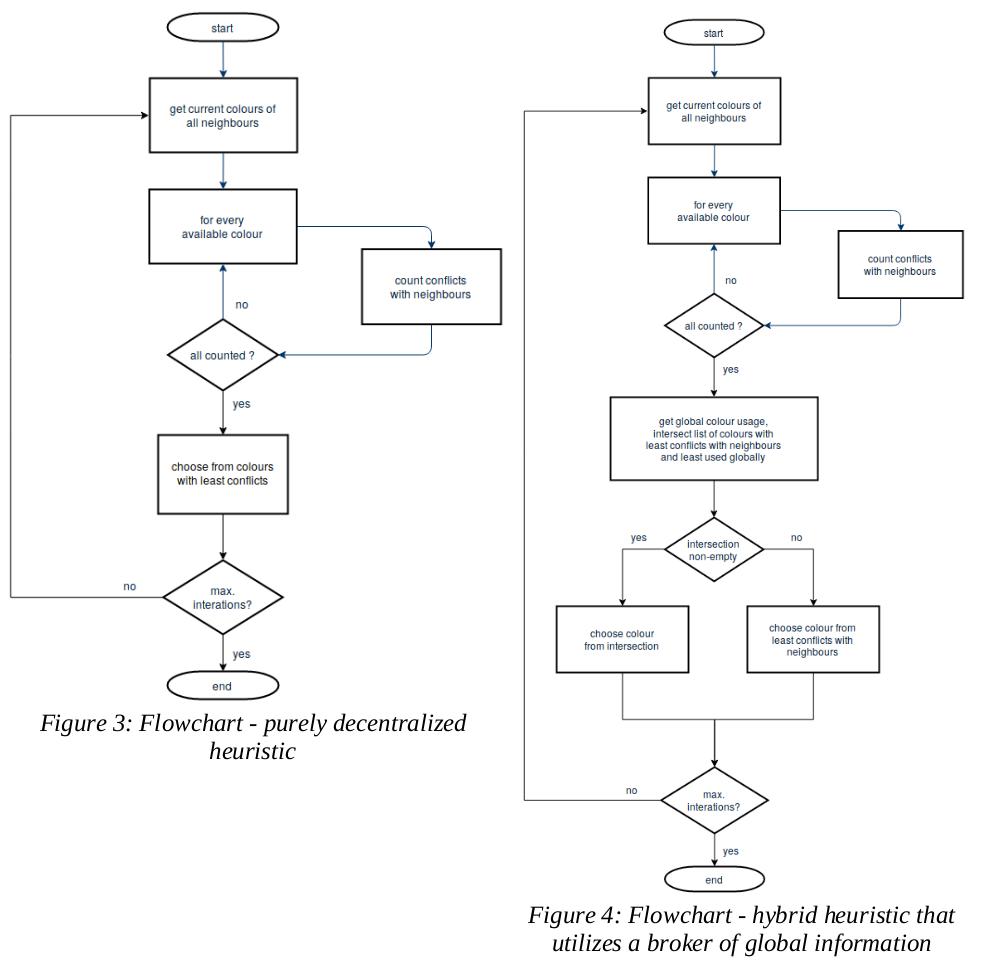
Oliver Antons; Julia C. Bendul
The impact of information and communication flow structures on logistics performance in Industry 4.0 production systems with distributed control Proceedings Article
In: EurOMA19, 2019.
Abstract | BibTeX | Schlagwörter: Distributed control, Graph colouring dynamics, Scheduling
@inproceedings{Antons-2019-ID692,
title = {The impact of information and communication flow structures on logistics performance in Industry 4.0 production systems with distributed control},
author = {Oliver Antons and Julia C. Bendul},
year = {2019},
date = {2019-01-01},
urldate = {2019-01-01},
booktitle = {EurOMA19},
abstract = {This paper studies the influence of accessible information and communication in distributed control systems for Industry 4.0 applications. To this end, we compare a purely decentralized and a hybrid heuristic in a multi-agent system in order to solve graph colouring dynamics. By means of simulations, both heuristics are compared, and while no heuristic clearly dominates the other, insights on design challenges in distributed control are derived.},
keywords = {Distributed control, Graph colouring dynamics, Scheduling},
pubstate = {published},
tppubtype = {inproceedings}
}
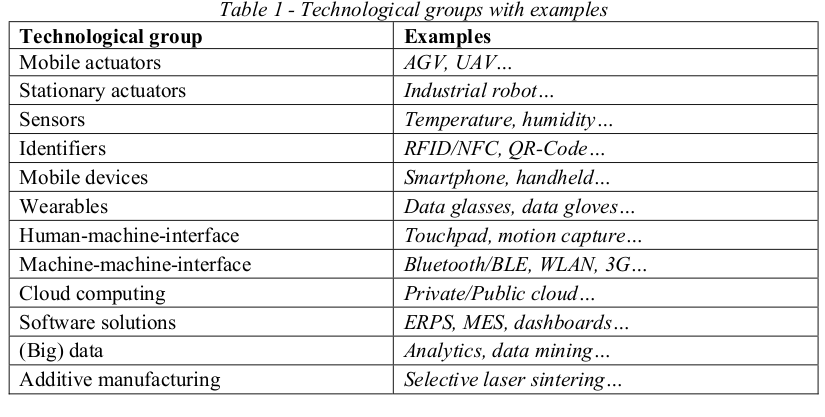
Manuel Zimmermann; Oliver Antons; Julia C. Bendul
Determinants of risk factors and benefits associated with Industry 4.0 technologies: Insights from German cases Proceedings Article
In: EurOMA19, 2019.
Abstract | BibTeX | Schlagwörter: Digitalization, Industry 4.0, Risk management
@inproceedings{Zimmermann-2019-ID693,
title = {Determinants of risk factors and benefits associated with Industry 4.0 technologies: Insights from German cases},
author = {Manuel Zimmermann and Oliver Antons and Julia C. Bendul},
year = {2019},
date = {2019-01-01},
urldate = {2019-01-01},
booktitle = {EurOMA19},
abstract = {This study investigates the application of current Industry 4.0 technologies as measures to counteract risk factors within companies and supply chains. Drawing on a database of more than 300 Industry 4.0 projects all around Germany, this study not only empirically explores which technologies and risk factors are currently relevant among practitioners \textendash but also evaluates the applicability of various technological solutions for the purpose of mitigating existing supply chain risks.},
keywords = {Digitalization, Industry 4.0, Risk management},
pubstate = {published},
tppubtype = {inproceedings}
}
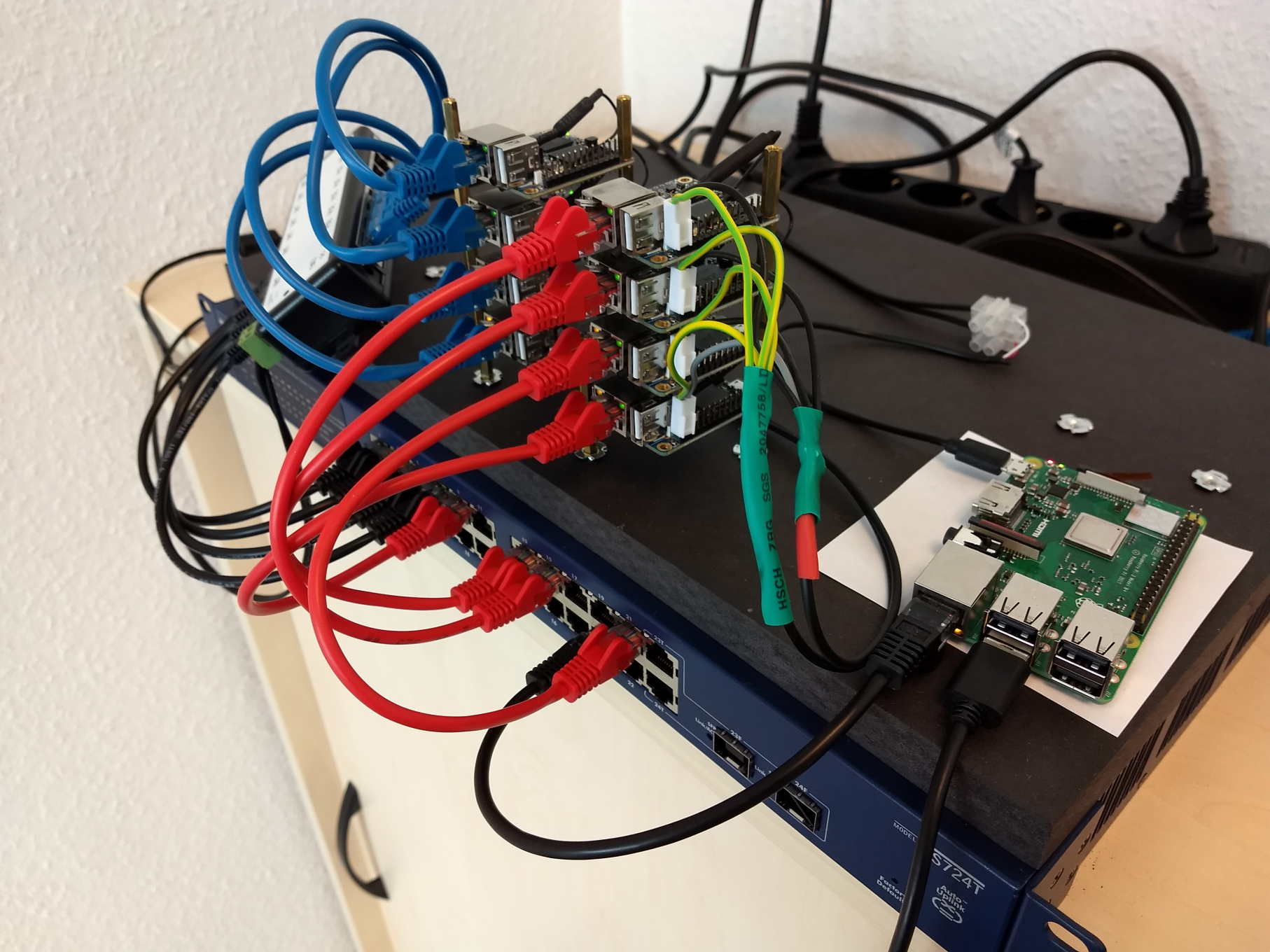
Oliver Antons; Julia C. Bendul
Decision making in industry 4.0--a comparison of distributed control approaches Proceedings Article
In: International Workshop on Service Orientation in Holonic and Multi-Agent Manufacturing, S. 329–339, Springer 2019.
Abstract | Links | BibTeX | Schlagwörter: Distributed control, Graph colouring dynamics, Industry 4.0, Multi-agent system
@inproceedings{antons2019decision,
title = {Decision making in industry 4.0--a comparison of distributed control approaches},
author = {Oliver Antons and Julia C. Bendul},
url = {https://link.springer.com/chapter/10.1007/978-3-030-27477-1_25},
doi = {10.1007/978-3-030-27477-1_25},
year = {2019},
date = {2019-01-01},
urldate = {2019-01-01},
booktitle = {International Workshop on Service Orientation in Holonic and Multi-Agent Manufacturing},
pages = {329--339},
organization = {Springer},
abstract = {This paper studies the influence of available information and coordination in distributed control systems for Industry 4.0. A purely decentralized and a hybrid heuristic are deployed in a Multi-Agent System in order to solve graph coloring dynamics. By means of simulation a multitude of instances is evaluated, showing a superior performance of the hybrid heuristic in most cases, underlining the importance of information availability and coordination in distributed control.},
keywords = {Distributed control, Graph colouring dynamics, Industry 4.0, Multi-agent system},
pubstate = {published},
tppubtype = {inproceedings}
}